REASSEMBLY LEARNING FOR SOUND EVENT LOCALIZATION AND DETECTION USING CRNN AND TRELLISNET Technical Report
semanticscholar(2019)
摘要
This technical report proposes a deep learning based approach, reassembly learning, for polyphonic sound event localization and detection. Sound event localization and detection is a joint task of two dependent sub-tasks: sound event detection and direction of arrival estimation. Joint learning has performance degradation compared to learning each sub-task separately. For this reason, we propose a reassembly learning to design a single network that deals with dependent sub-tasks together. Reassembly learning is a method to divide multi-task into individual sub-tasks, to train each sub-task, and then to reassemble and fine-tune into a single network. Experimental results show that the reassembly learning has good performance in the sound event localization and detection. Besides, the convolutional recurrent neural networks have been known as a state of art in both sound classification and detection applications. In DCASE 2019 challenge task 3, we suggest new architecture, trellis network based on temporal convolution networks, which can replace the convolutional recurrent neural networks. Trellis network shows a strong point in the direction of arrival estimation and has the possibility of being applied to a variety of sound classification and detection applications.
更多查看译文
AI 理解论文
溯源树
样例
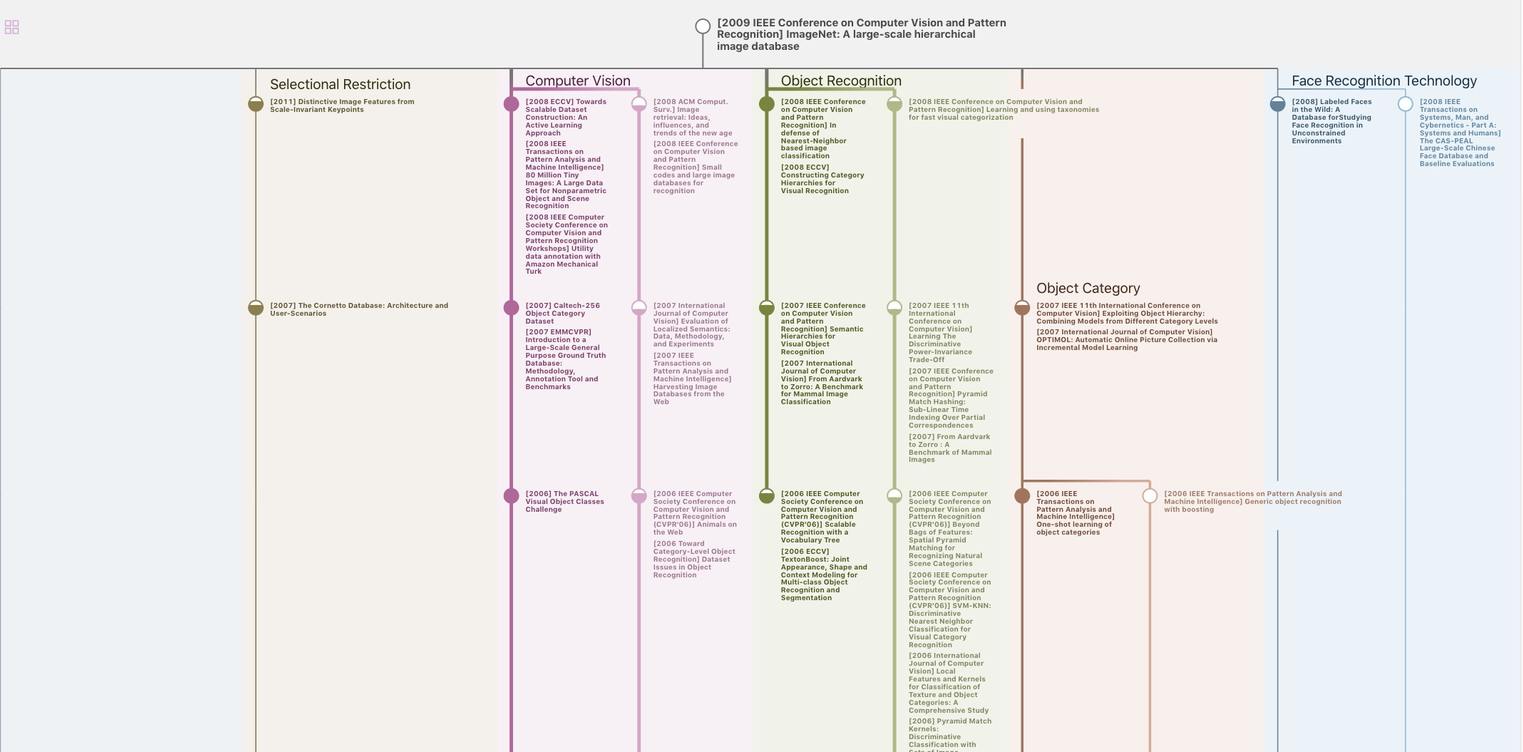
生成溯源树,研究论文发展脉络
Chat Paper
正在生成论文摘要