Predicting risk of stroke from lab tests using machine learning algorithms (Preprint)
semanticscholar(2020)
摘要
UNSTRUCTURED
Stroke, a cerebrovascular disease, is one of the major causes of death. It is also causing a health burden for both the patients and the healthcare systems. One of the important risk factors of stroke is health behavior which is an increasing focus of prevention. In addition, chronic diseases such as hypertension, diabetes, cardiac diseases, and asthma are potential risk factors for stroke. There are a lot of machine learning that built using predictors such as lifestyle or radiology imaging. However, there are no models built using lab tests. The aim of the study is to fill this gap by building prediction models to predict stroke from lab tests. We utilized the National Health and Nutrition Examination Survey (NHNES) data sets to develop models that would predict stroke from patient lab tests. We found that accurate and sensitive machine learning models can be created to predict stroke from lab tests. The results showed that prediction with the best tested algorithm random forest could reach the highest accuracy (ACC = 0.96) when all the attributes were used. The model proposed can be integrated with electronic health records to provide a real-time prediction of stroke from lab tests. Due to the data, we could not predict the type of stroke wither hemorrigic or ischemic. In future studies, we aim to use data that provide different types of stroke and explore the data to build a prediction model of each type.
更多查看译文
AI 理解论文
溯源树
样例
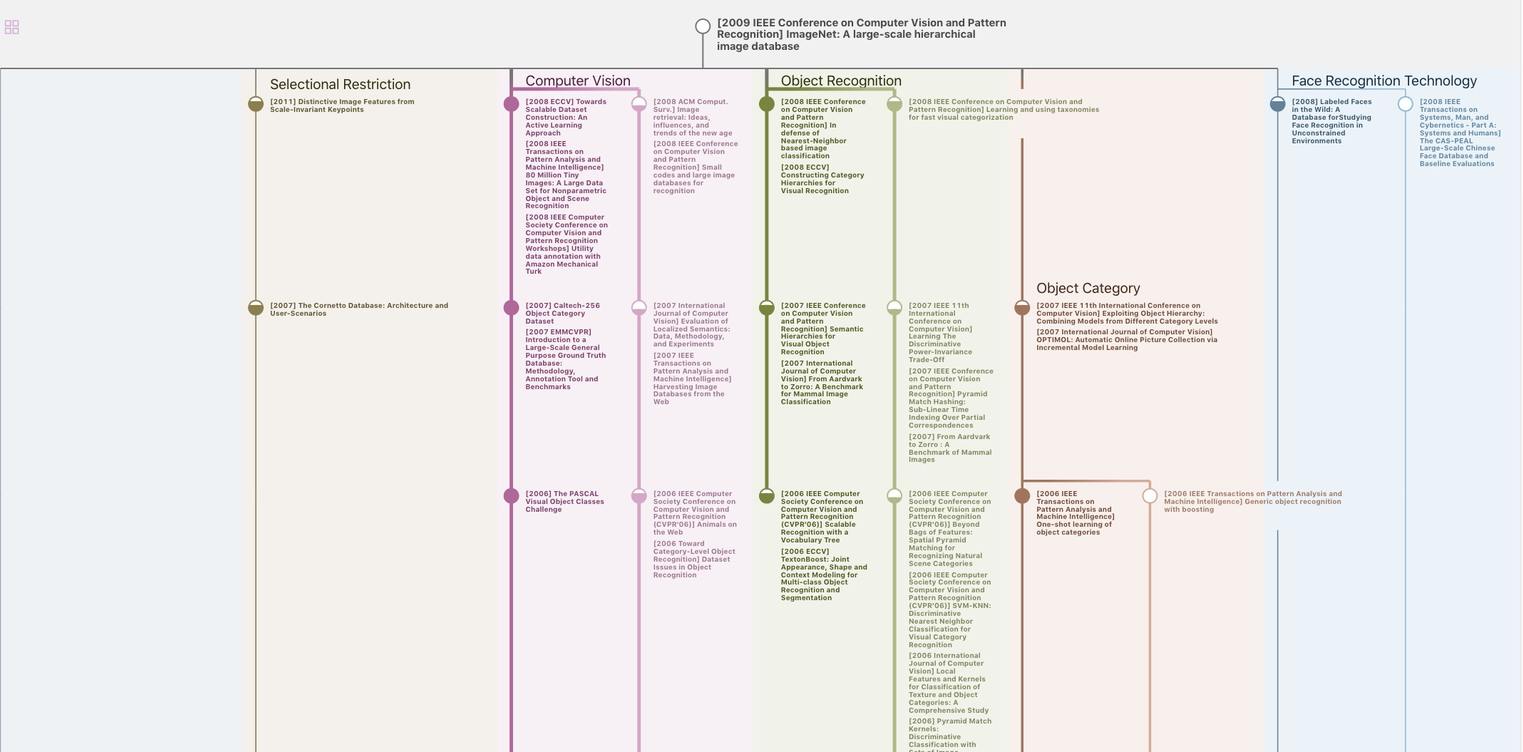
生成溯源树,研究论文发展脉络
Chat Paper
正在生成论文摘要