D2CL: A Dense Dilated Convolutional LSTM Model for Sea Surface Temperature Prediction
IEEE JOURNAL OF SELECTED TOPICS IN APPLIED EARTH OBSERVATIONS AND REMOTE SENSING(2021)
摘要
Accurately predicting sea surface temperature (SST) is practically important to many applications, such as weather forecasting, ocean environment protection, and marine disaster prevention. The major challenge for predicting SST is to capture both the spatial and temporal characteristics of SST, which has not yet been well addressed by the existing methods. In this work, we proposed a novel dense dilated convolutional LSTM (D2CL) model to predict SST. D2CL first integrates dilated convolutional network and LSTM to learn spatial and temporal features from the SST data simultaneously. Then, it uses multiple feature extractors of different dilated kernel sizes to learn features of varying scales. Finally, it introduces dense connection to reduce feature loss during the training process and achieves SST prediction in an encoder-decoder style. We have conducted extensive experiments over two real datasets to validate the effectiveness of D2CL and all the proposed enhancing techniques. As suggested by the experimental results, D2CL outperforms the existing methods and could achieve accurate SST prediction for as long as seven days in future.
更多查看译文
关键词
Predictive models,Ocean temperature,Mathematical models,Feature extraction,Data models,Atmospheric modeling,Support vector machines,Big data analytics,dilated ConvLSTM,sea surface temperature (SST) prediction,spatial and temporal features
AI 理解论文
溯源树
样例
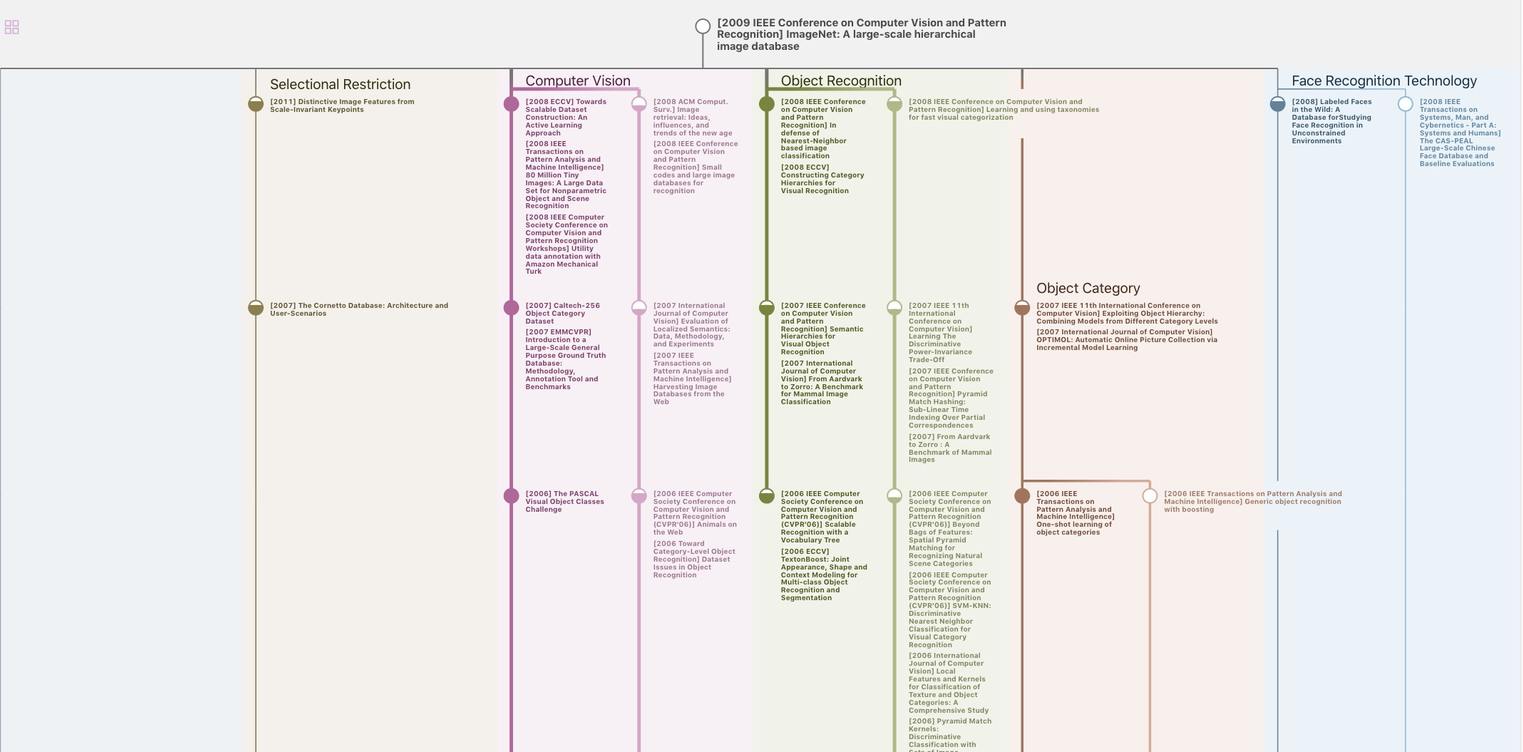
生成溯源树,研究论文发展脉络
Chat Paper
正在生成论文摘要