Evaluating word embeddings for computational pragmatics
semanticscholar(2021)
摘要
Word embeddings are a popular set of machine learning techniques that are used in natural language processing to create a semantic model of a corpus, representing semantic relationship within a geometric space. In this space every word is mapped to a vector so that words with similar meanings are represented close to one another while words with different meanings are distant. In this work we briefly present a novel use of word embedding for computational pragmatics in which we used word2vec Skip-Gram (henceforth SG) to explore the inferential model generated by algorithmic personalization on Facebook. We used their experiment in a recent study in which we implemented Eco's model reader as an inferential model. In a nutshell, we found that we can simulate the model reader with word embedding to create computational inferential models, that are then compared to identify a possible divergence of interpretation paths. Our experiment conducted on Facebook showed that algorithmic personalization influences model readers, differentiating inferences across different timelines. Though for pragmatic goals, word embeddings have two significant issues that are 1) accuracy 2) variability. The standard way of evaluating the accuracy of word embeddings is to use intrinsic evaluation, using predefined analogies and similarities such as WordSim-353 (Finkelstein et al. 2002) and SimLex. However, this accuracy is quite hard to estimate while inquiring pragmatic aspects as we do not have any predefined pragmatic output. This work aims to review these methods for a computational pragmatic approach, highlighting possible limitations and adaptations. The review will cover three aspects: 1) Reproducibility of computational pragmatics 2) Reliability assessment for computational pragmatics inferences 3) Accuracy test for computational pragmatics goals
更多查看译文
AI 理解论文
溯源树
样例
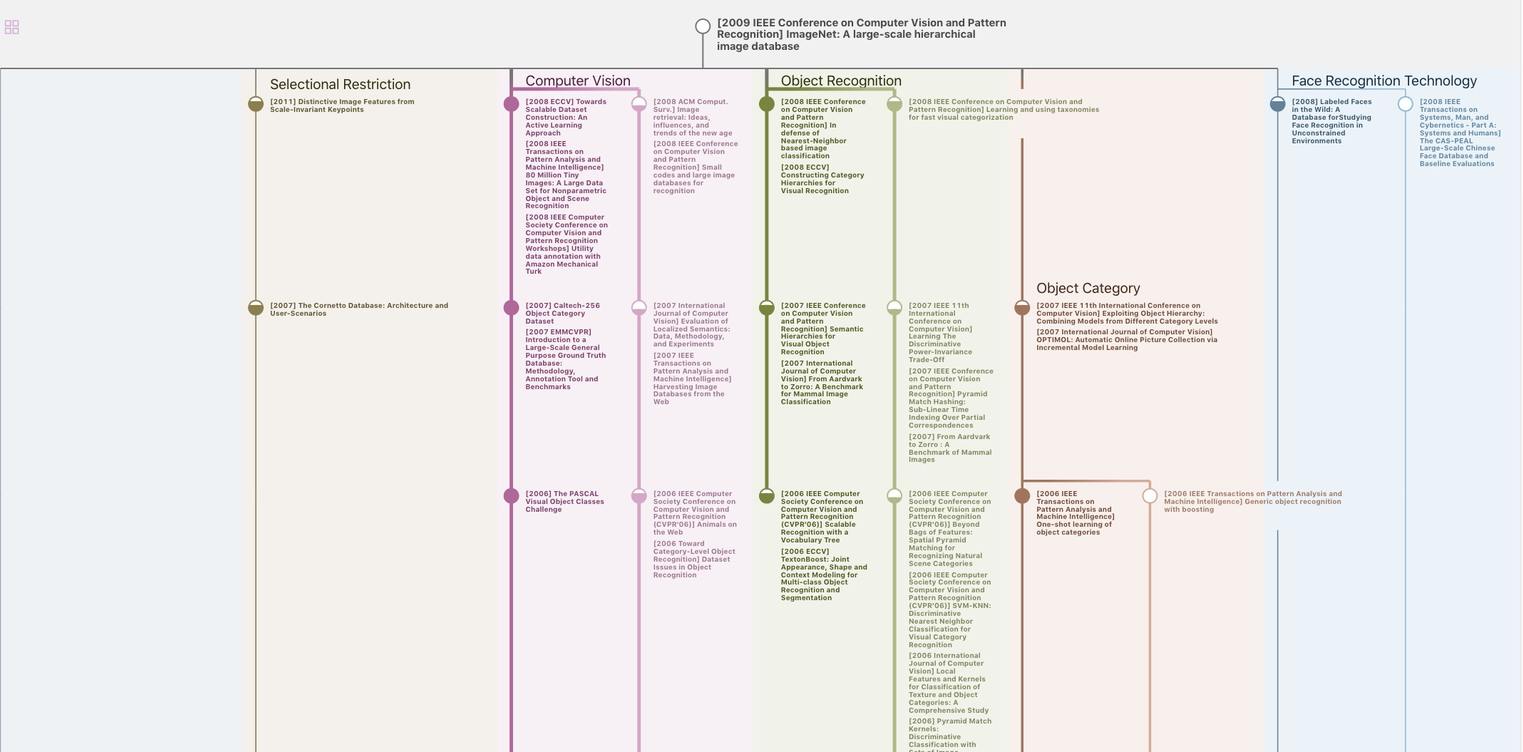
生成溯源树,研究论文发展脉络
Chat Paper
正在生成论文摘要