Learning to Shape Rewards using a Game of Switching Controls
ArXiv(2021)
Abstract
Reward shaping (RS) is a powerful method in reinforcement learning (RL) for overcoming the problem of sparse or uninformative rewards. However, RS typically relies on manually engineered shaping-reward functions whose construction is timeconsuming and error-prone. It also requires domain knowledge which runs contrary to the goal of autonomous learning. We introduce Reinforcement Learning Optimal Shaping Algorithm (ROSA), an automated RS framework in which the shapingreward function is constructed in a novel Markov game between two agents. A reward-shaping agent (Shaper) uses switching controls to determine which states to add shaping rewards and their optimal values while the other agent (Controller) learns the optimal policy for the task using these shaped rewards. We prove that ROSA, which easily adopts existing RL algorithms, learns to construct a shapingreward function that is tailored to the task thus ensuring efficient convergence to high performance policies. We demonstrate ROSA’s congenial properties in three carefully designed experiments and show its superior performance against state-of-the-art RS algorithms in challenging sparse reward environments.
MoreTranslated text
Key words
shape rewards,switching controls,learning
AI Read Science
Must-Reading Tree
Example
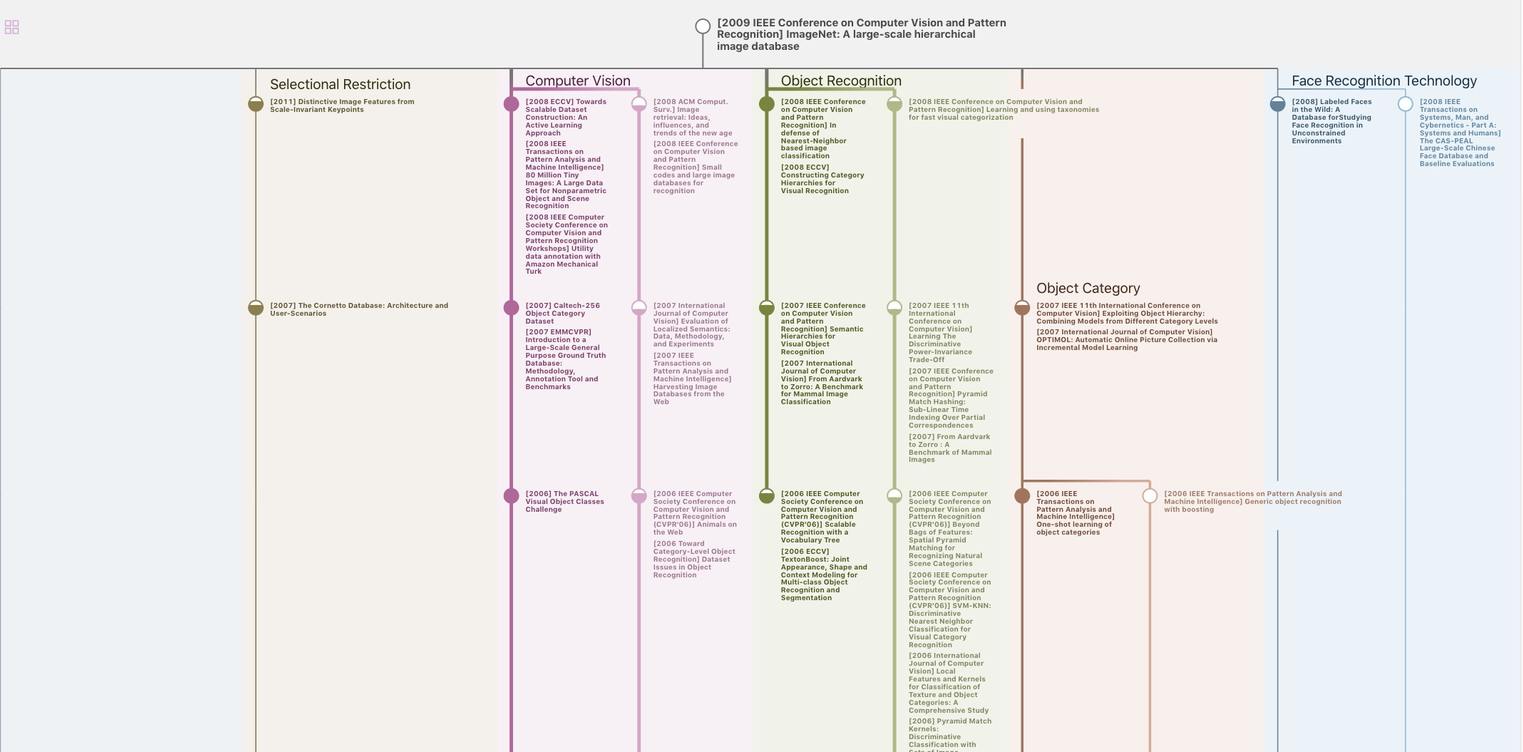
Generate MRT to find the research sequence of this paper
Chat Paper
Summary is being generated by the instructions you defined