Covering rough set-based incremental feature selection for mixed decision system
SOFT COMPUTING(2022)
摘要
Covering rough sets conceptualize different types of features with their respective generated coverings. By integrating these coverings into a single covering, covering rough set-based feature selection finds valuable features from a mixed decision system with symbolic, real-valued, missing-valued and set-valued features. Existing approaches to covering rough set-based feature selection, however, are intractable to handle large mixed data. Therefore, an efficient strategy of incremental feature selection is proposed by presenting a mixed data set in sample subsets one after another. Once a new sample subset comes in, the relative discernible relation of each feature is updated to disclose incremental feature selection scheme that decides the strategies of increasing informative features and removing redundant features. The incremental scheme is applied to establish two incremental feature selection algorithms from large or dynamic mixed data sets. The first algorithm updates the feature subset upon the sequent arrival of sample subsets and returns the reduct when no further sample subsets are obtained. The second one merely updates the relative discernible relations and finds the reduct when no subsets are obtained. Extensive experiments demonstrate that the two proposed incremental algorithms, especially the second one speeds up covering rough set-based feature selection without sacrificing too much classification performance.
更多查看译文
关键词
Covering rough sets, Feature selection, Incremental learning, Dynamic mixed data
AI 理解论文
溯源树
样例
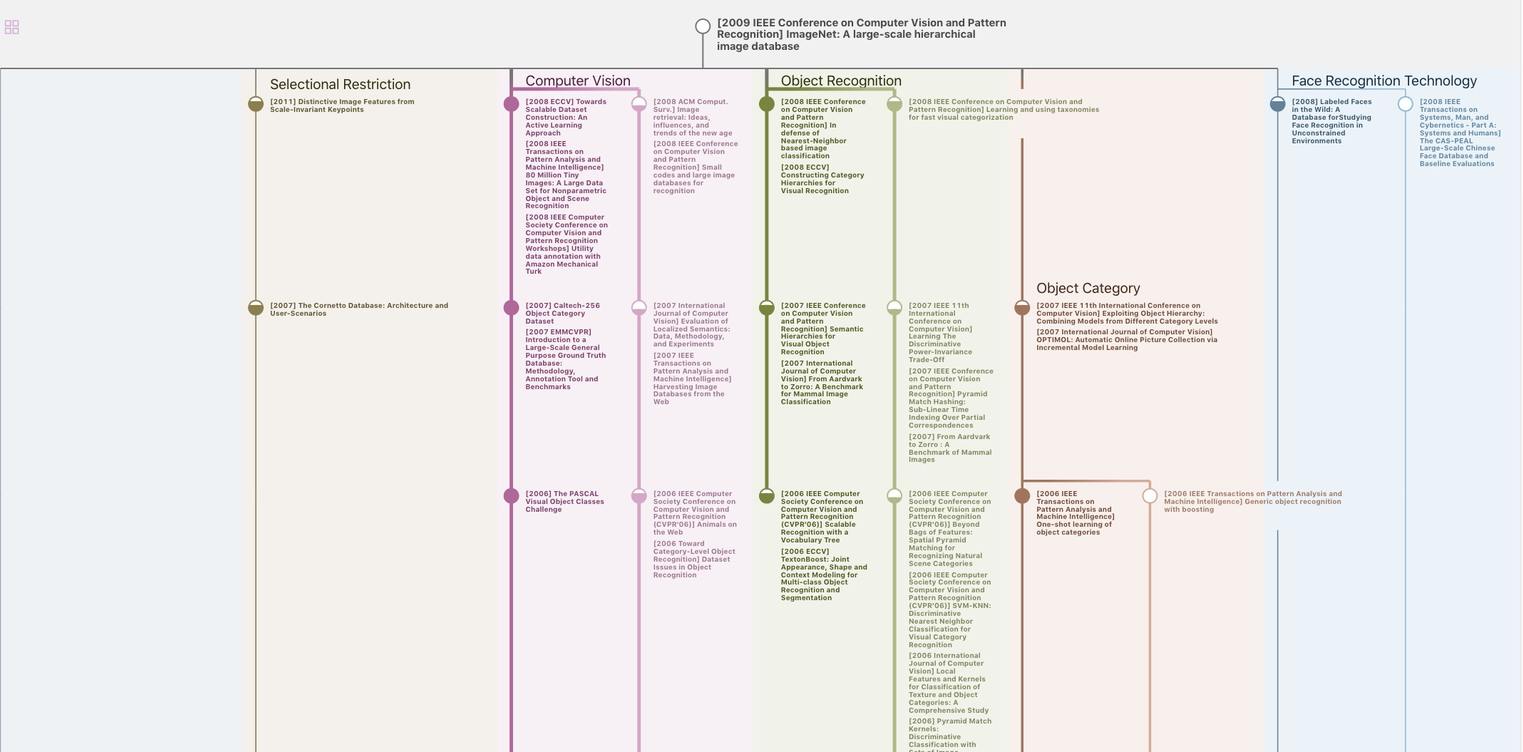
生成溯源树,研究论文发展脉络
Chat Paper
正在生成论文摘要