Theory Acquisition as Constraint-Based Program Synthesis.
CogSci(2021)
摘要
What computations enable humans to leap from mere observations to rich explanatory theories? Prior work has focused on stochastic algorithms that rely on random, local perturbations to model the search for satisfactory theories. Here we introduce a new approach inspired by the practice of ‘debugging’ from computer programming, whereby learners use past experience to constrain future proposals, and are thus able to consider large leaps in their current theory to fix specific deficiencies. We apply our ‘debugging’ algorithm to the magnetism domain introduced by (Ullman, Goodman, & Tenenbaum, 2010) and compare its efficiency and accuracy to their stochastic-search algorithm. We find that our algorithm not only requires fewer iterations to find a solution, but that the solutions it finds more reliably recover the correct latent theories, and are more robust to sparse data. Our findings suggest the promise of such constraint-based approaches to emulate the way humans efficiently navigate large, discrete hypothesis spaces.
更多查看译文
AI 理解论文
溯源树
样例
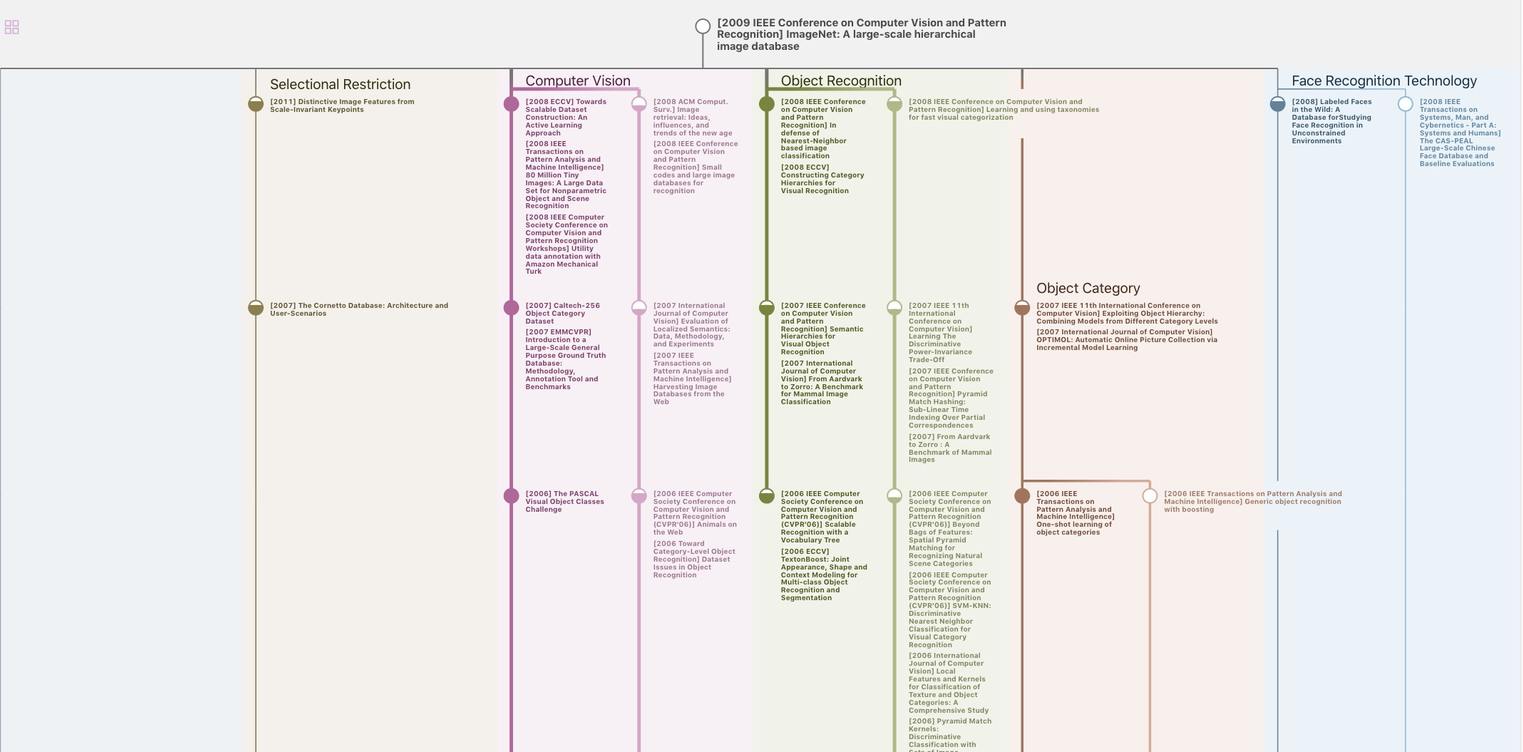
生成溯源树,研究论文发展脉络
Chat Paper
正在生成论文摘要