Preference Learning for Real-World Multi-Objective Decision Making
semanticscholar(2020)
摘要
In experimentation at Internet firms, it is common to make product decisions based on multiple competing or complementary objectives where the experimenter needs to select the most “favorable” option with the highest utility. However, despite the prevalence of this decision problem, such multi-objective decisions are often made in arguably sub-optimal ways. In this paper, we propose a framework that enables us to suggest the configuration in a potentially large action space that is expected to maximize the experimenter’s utility function. We demonstrate the efficacy of preference learning and our proposed framework with two user studies conducted at a large Internet firm. We show how the learned preference models can accurately recover machine learning engineers’ preferences in the user study and are most useful when trained on near-optimal regions where real-world tradeoffs are expected to happen the most.
更多查看译文
AI 理解论文
溯源树
样例
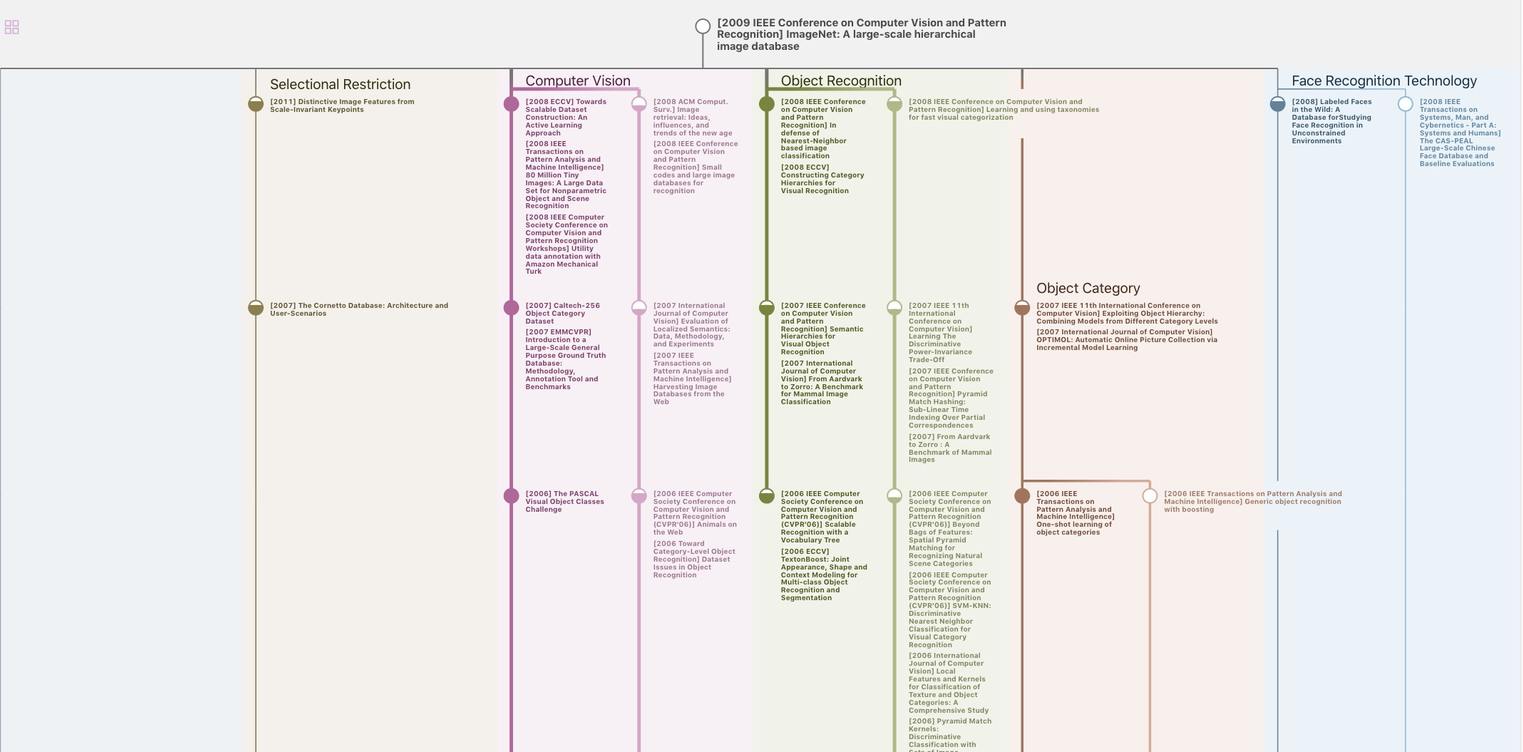
生成溯源树,研究论文发展脉络
Chat Paper
正在生成论文摘要