Threshold selection for cluster inference based on large deviation principles
HAL (Le Centre pour la Communication Scientifique Directe)(2021)
摘要
In the setting of regularly varying time series, a cluster of exceedances is a short period for which the supremum norm exceeds a high threshold. We propose to study a generalization of this notion considering short periods, or blocks, with p--norm above a high threshold. We derive large deviation principles of blocks and apply these results to improve cluster inference. We focus on blocks estimators and show they are consistent when we use large empirical quantiles from the p --norm of blocks as threshold levels. We derive an adaptive threshold selection method for cluster inference in p. Our approach focuses on the case p < $\infty$ rather than the classical one for p = $\infty$ where the bias is more difficult to control.
更多查看译文
关键词
cluster inference,large deviation principles,selection
AI 理解论文
溯源树
样例
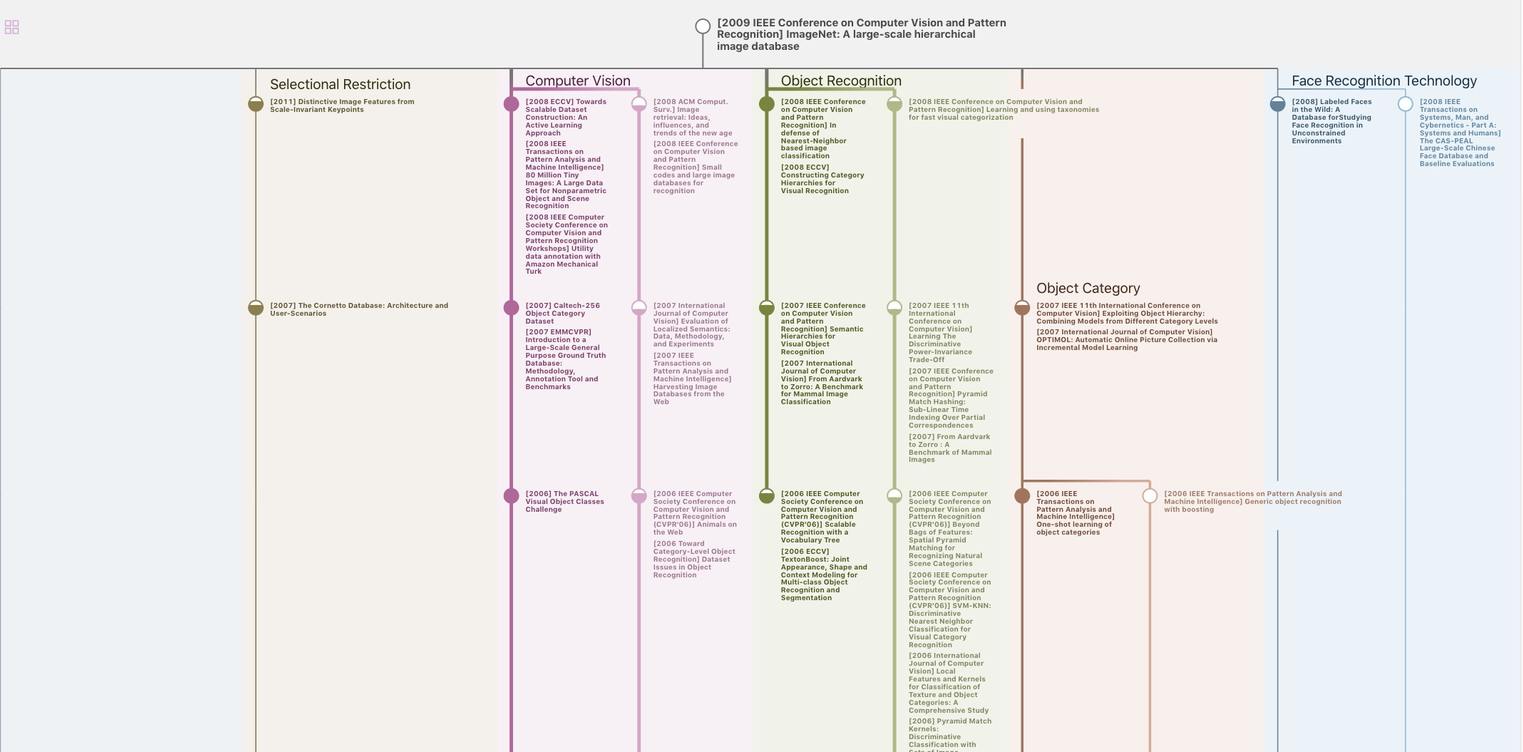
生成溯源树,研究论文发展脉络
Chat Paper
正在生成论文摘要