Boosting RANSAC via Dual Principal Component Pursuit.
arXiv (Cornell University)(2021)
摘要
In this paper, we revisit the problem of local optimization in RANSAC. Once a so-far-the-best model has been found, we refine it via Dual Principal Component Pursuit (DPCP), a robust subspace learning method with strong theoretical support and efficient algorithms. The proposed DPCP-RANSAC has far fewer parameters than existing methods and is scalable. Experiments on estimating two-view homographies, fundamental and essential matrices, and three-view homographic tensors using large-scale datasets show that our approach consistently has higher accuracy than state-of-the-art alternatives.
更多查看译文
关键词
dual principal component pursuit,ransac
AI 理解论文
溯源树
样例
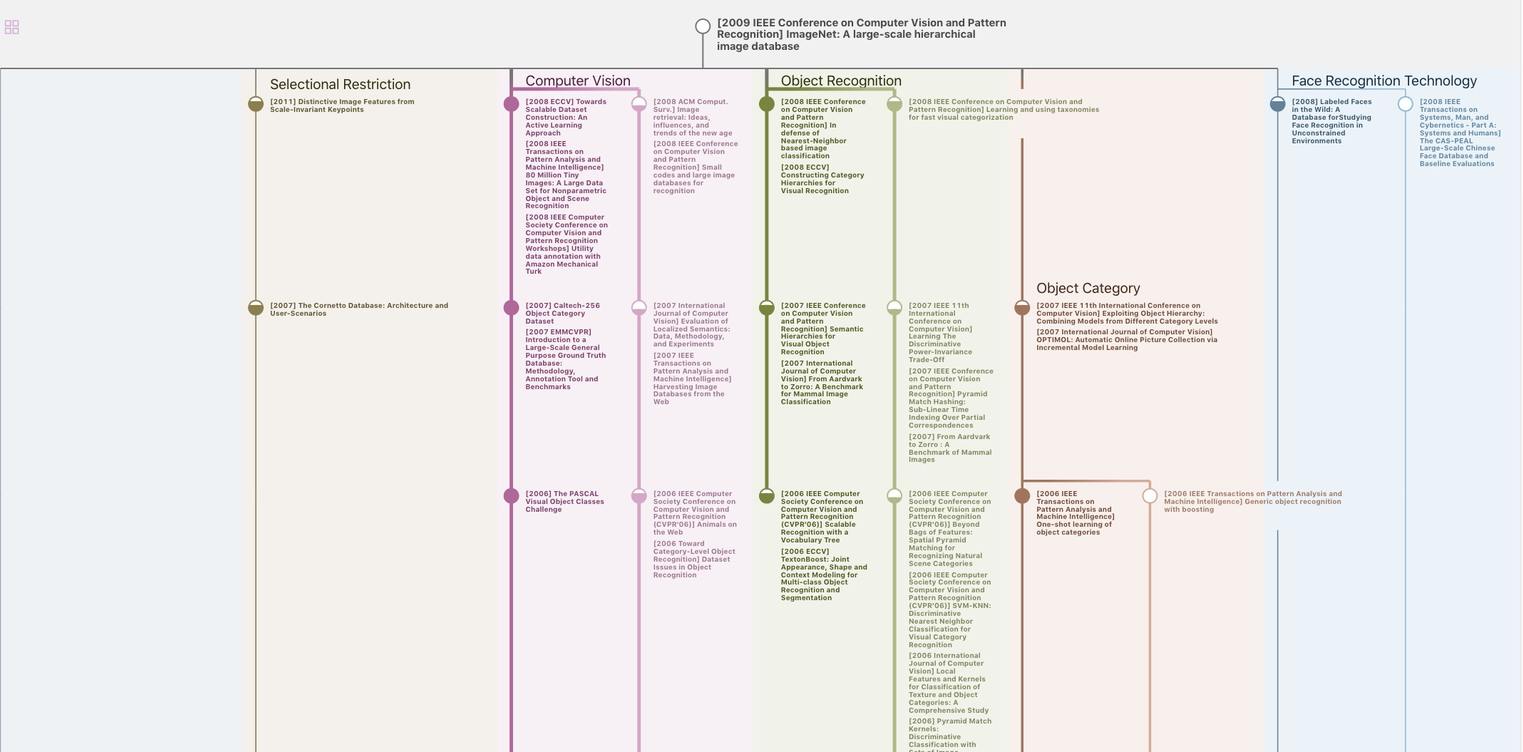
生成溯源树,研究论文发展脉络
Chat Paper
正在生成论文摘要