Implementing Predictive Model for Child Mortality in Afghanistan
Proceedings of Sixth International Congress on Information and Communication Technology(2021)
摘要
Reduction of child mortality and improving child health are health priorities in underdeveloped and developing countries. Afghanistan has a high rank of child mortality. Therefore, the ability to predict child mortality is beneficial and a good preventive measure. This study aims to develop a child mortality predictive model by utilizing data mining classification algorithms and identify the most suitable classifier among the five popular machine learning techniques. These are K-Nearest Neighbors (K-NN), Naïve Bayes (NB), Neural Network (NN), Random Forest (RF), and Support Vector Machine (SVM). The dataset used is the Afghanistan Demographic and Health Survey (AfDHS). The well-known Correlation-based Feature Selection (CFS) algorithm is employed to select the top 13 attributes during the data preprocessing. The classification in this study comprises two categories, ‘Alive’ and ‘Dead’. Preparation of the dataset is carefully done to ensure well-balanced samples in each category. The validation of the predictive models is assessed by means of Accuracy, Precision, Recall, and Area Under the Receiver Operating Characteristic Curve (AUC). The study reveals that Random Forest is the best classifier. The result obtained from this study can be beneficial to child health improvement programs in Afghanistan as well as in policy-making, especially when resources are limited.
更多查看译文
关键词
Afghanistan, Afghanistan demographic and health survey, Classifiers, Child mortality, Data mining
AI 理解论文
溯源树
样例
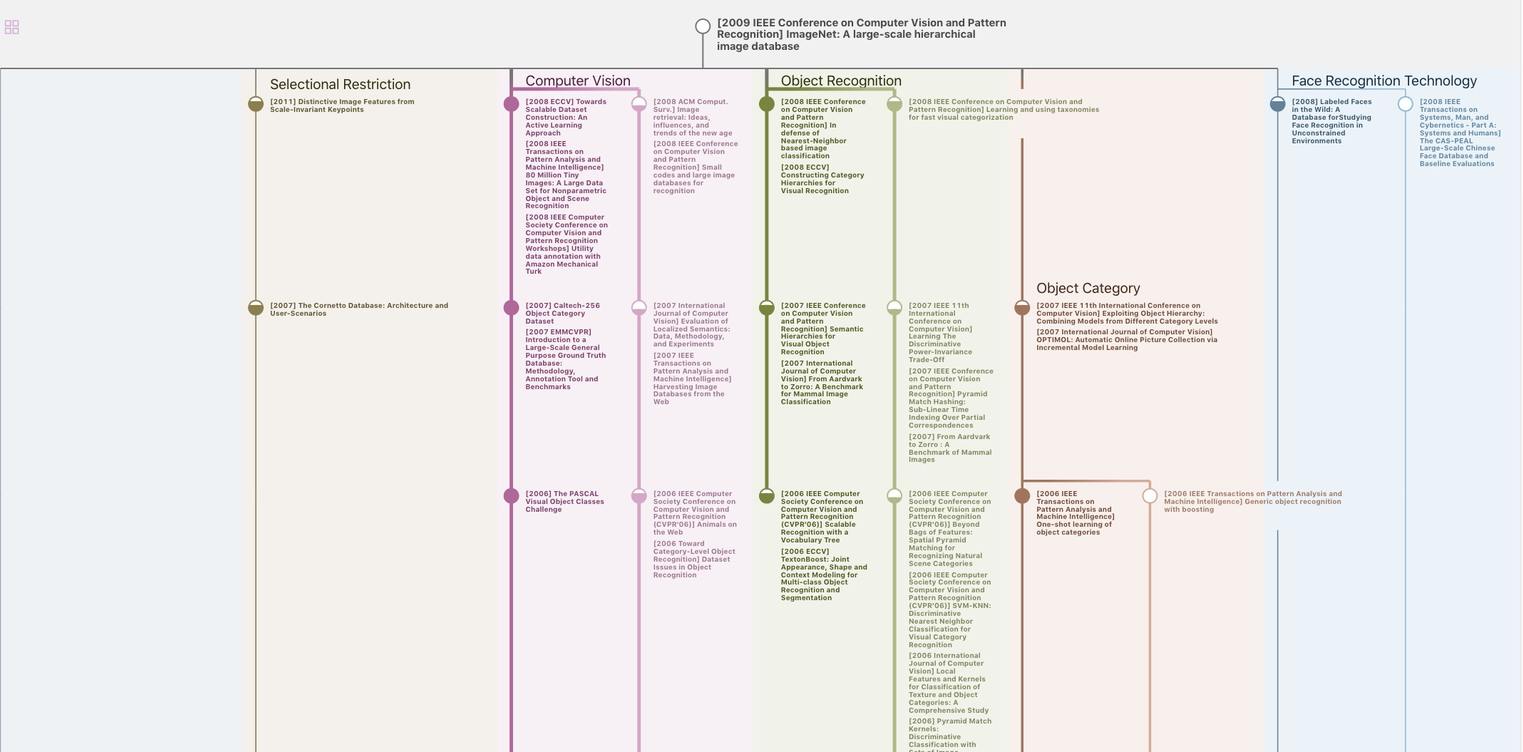
生成溯源树,研究论文发展脉络
Chat Paper
正在生成论文摘要