Identifying Causal Effects in Experiments with Spillovers and Non-compliance
Journal of Econometrics(2021)
摘要
This paper shows how to use a randomized saturation experimental design to identify and estimate causal effects in the presence of spillovers–one person’s treatment may affect another’s outcome–and one-sided non-compliance–subjects can only be offered treatment, not compelled to take it up. Two distinct causal effects are of interest in this setting: direct effects quantify how a person’s own treatment changes her outcome, while indirect effects quantify how her peers’ treatments change her outcome. We consider the case in which spillovers occur only within known groups, and take-up decisions do not depend on peers’ offers. In this setting we point identify local average treatment effects, both direct and indirect, in a flexible random coefficients model that allows for both heterogenous treatment effects and endogeneous selection into treatment. We go on to propose a feasible estimator that is consistent and asymptotically normal as the number and size of groups increases. We apply our estimator to data from a large-scale job placement services experiment, and find negative indirect treatment effects on the likelihood of employment for those willing to take up the program. These negative spillovers are offset by positive direct treatment effects from own take-up.
更多查看译文
关键词
C21,C26
AI 理解论文
溯源树
样例
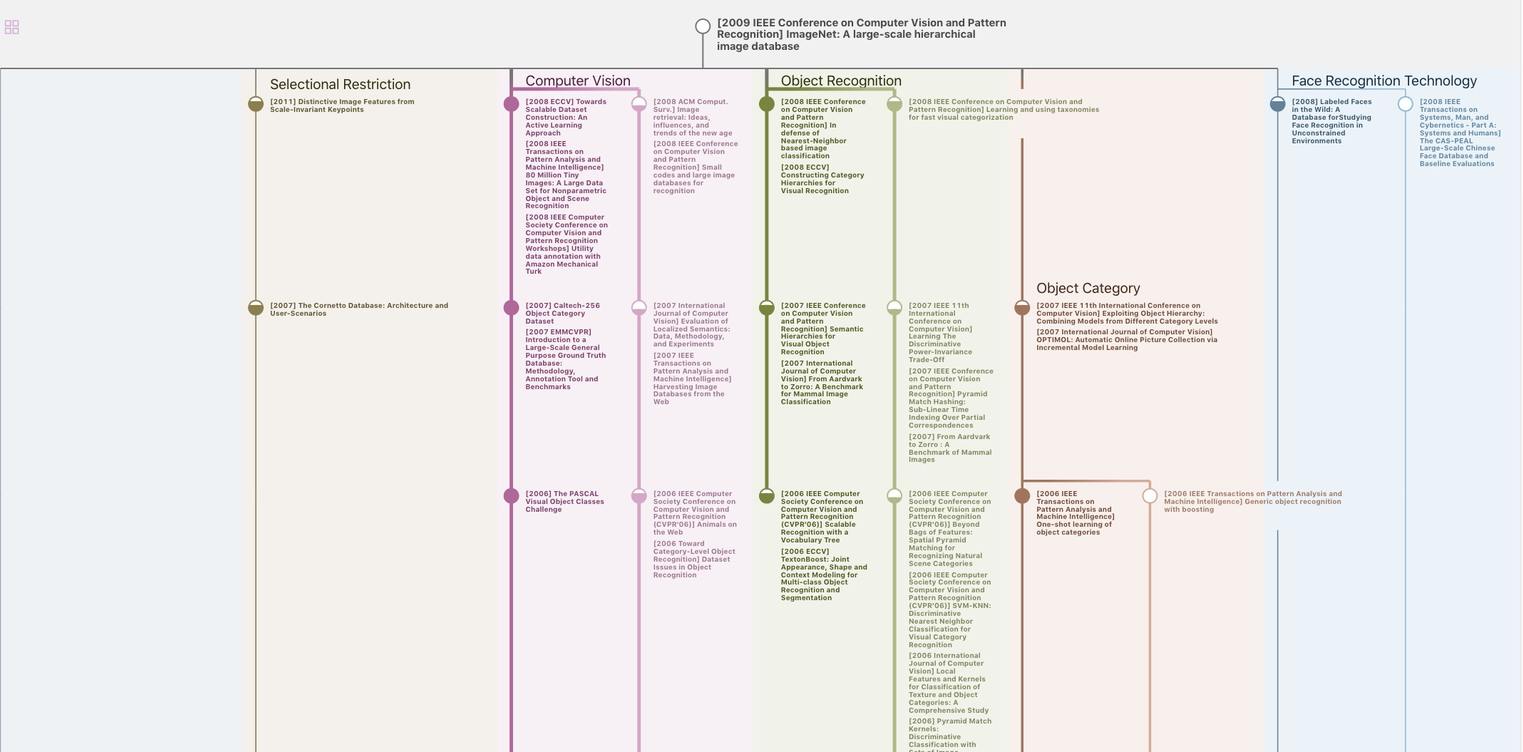
生成溯源树,研究论文发展脉络
Chat Paper
正在生成论文摘要