InfinityGAN: Towards Infinite-Pixel Image Synthesis
International Conference on Learning Representations (ICLR)(2022)
摘要
We present a novel framework, InfinityGAN, for arbitrary-sized image generation. The task is associated with several key challenges. First, scaling existing models to an arbitrarily large image size is resource-constrained, in terms of both computation and availability of large-field-of-view training data. InfinityGAN trains and infers in a seamless patch-by-patch manner with low computational resources. Second, large images should be locally and globally consistent, avoid repetitive patterns, and look realistic. To address these, InfinityGAN disentangles global appearances, local structures, and textures. With this formulation, we can generate images with spatial size and level of details not attainable before. Experimental evaluation validates that InfinityGAN generates images with superior realism compared to baselines and features parallelizable inference. Finally, we show several applications unlocked by our approach, such as spatial style fusion, multi-modal outpainting, and image inbetweening. All applications can be operated with arbitrary input and output sizes. Please find the full version of the paper at https://openreview.net/forum?id=ufGMqIM0a4b .
更多查看译文
关键词
synthesis,image,infinite-pixel
AI 理解论文
溯源树
样例
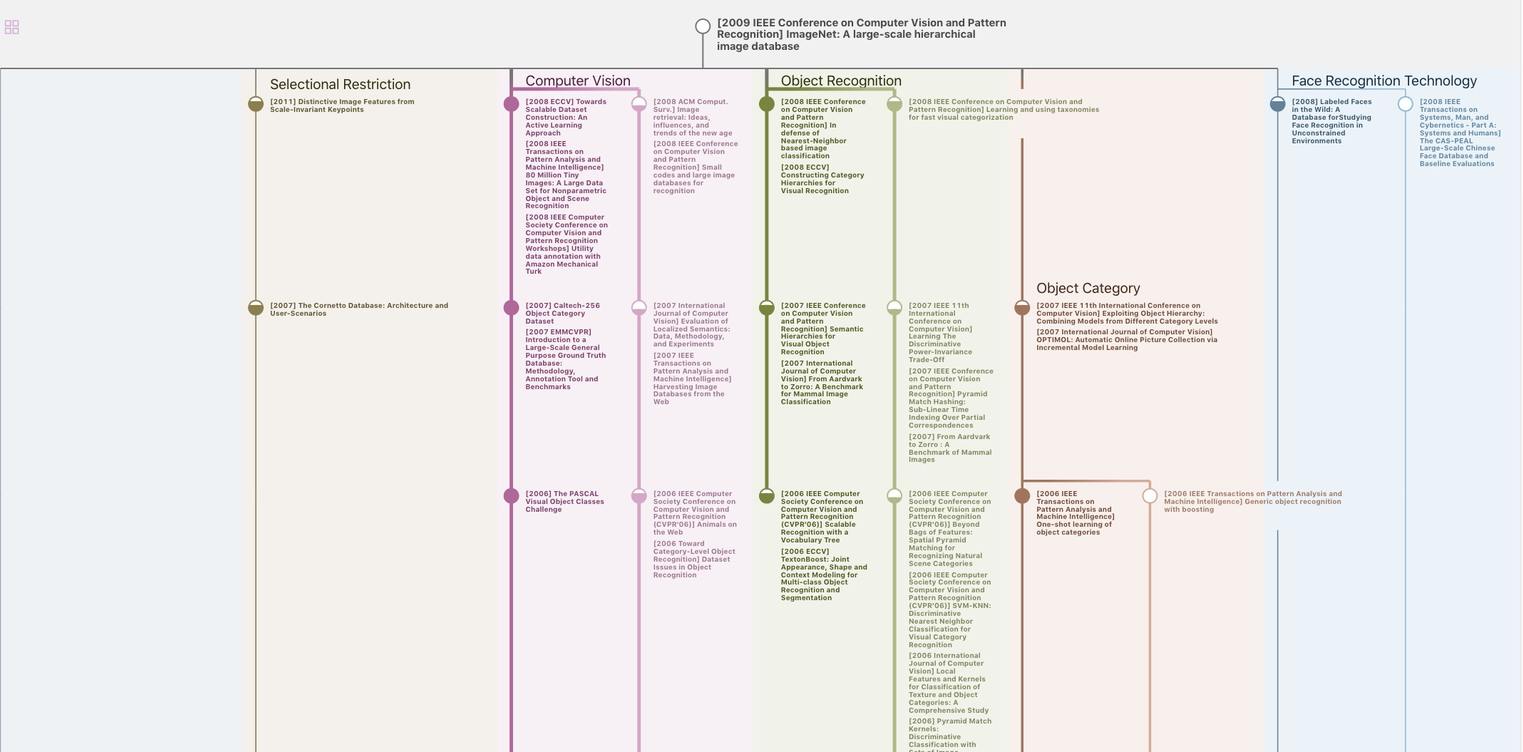
生成溯源树,研究论文发展脉络
Chat Paper
正在生成论文摘要