Minimizing Misclassification Cost in Healthcare Associated Infection
semanticscholar(2019)
摘要
Motivated by healthcare associated infection (HAI), which is estimated to cost US hospitals $9.8 billion per year, we develop a machine-learning based scheme to administer patient-targeted preventive measures upon admissions to the hospital. The model involves an objective function that acknowledges the asymmetry between the cost of missing an infection and the cost of a ”false alarm,” the former (real cost to the hospital) could be order-of-magnitude higher than the latter (minor expenses for certain preventive measures, which are nonetheless imperfect). A two-step algorithm is devised to solve the problem in a much more efficient manner than a black-box algorithm such as deep neutral network, and with a clearly interpretable solution. We also provide convergence and rate-of-convergence results, in the form of distribution-free probabilistic guarantees, using a variation of the Dvoretzkey-Kiefer-Wolfowitz bound (as refined by Massart). We illustrate the performance of the model with real data from several largest hospitals in New York City.
更多查看译文
AI 理解论文
溯源树
样例
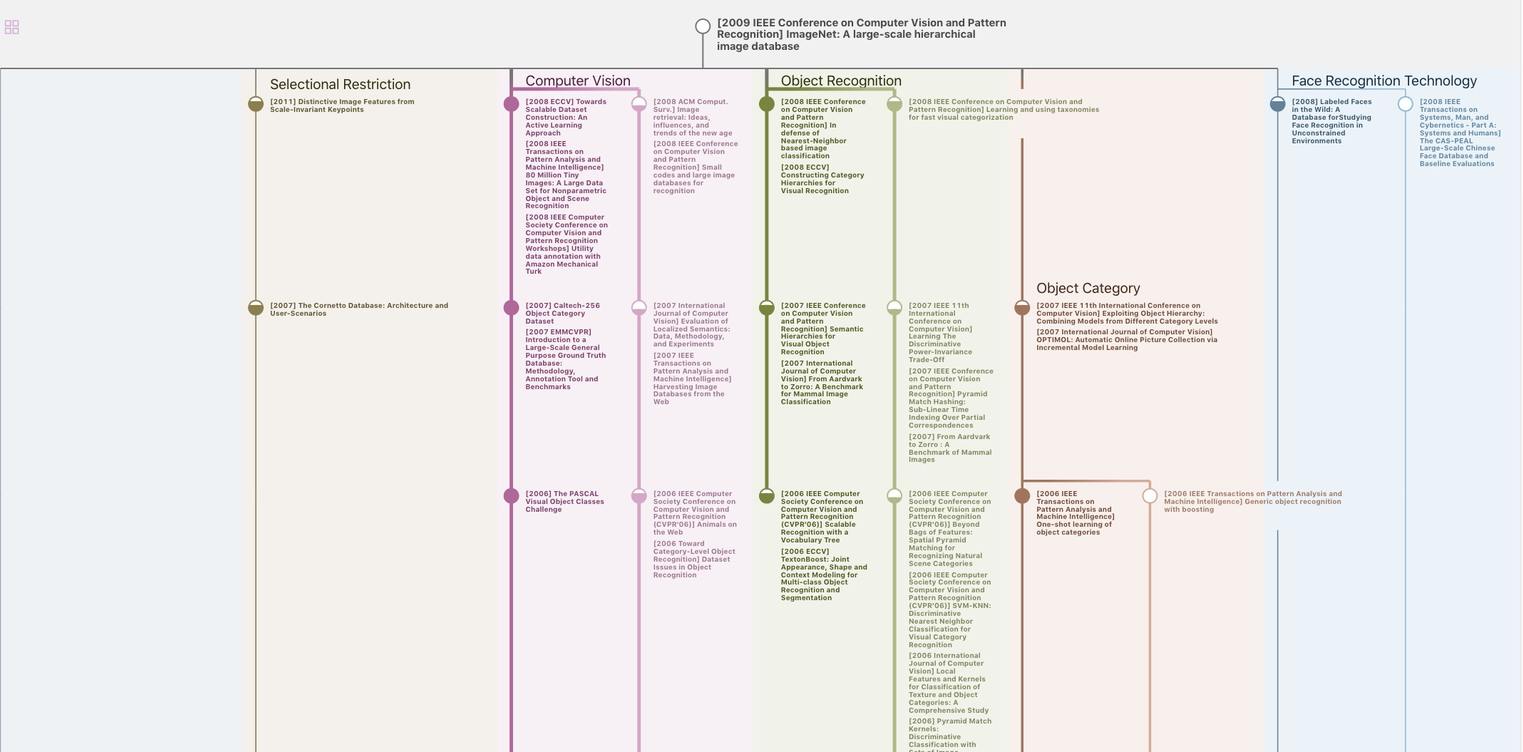
生成溯源树,研究论文发展脉络
Chat Paper
正在生成论文摘要