Appropriate Evaluation of Diagnostic Utility of Machine Learning Algorithm Generated Images.
Annual Conference on Neural Information Processing Systems(2020)
摘要
Generative machine learning (ML) methods can reduce time, cost, and radiation associated with medical image acquisition, compression, or generation techniques. While quantitative metrics are commonly used in the evaluation of ML generated images, it is unknown how well these quantitative metrics relate to the diagnostic utility of images. Here, fellowshiptrained radiologists provided diagnoses and qualitative evaluations on chest radiographs reconstructed from the current standard JPEG2000 or variational autoencoder (VAE) techniques. Cohen’s kappa coefficient measured the agreement of diagnoses based on different reconstructions. Methods that produced similar Fréchet inception distance (FID) showed similar diagnostic performances. Thus in place of timeintensive expert radiologist verification, an appropriate target FID – an objective quantitative metric – can evaluate the clinical utility of ML generated medical images.
更多查看译文
AI 理解论文
溯源树
样例
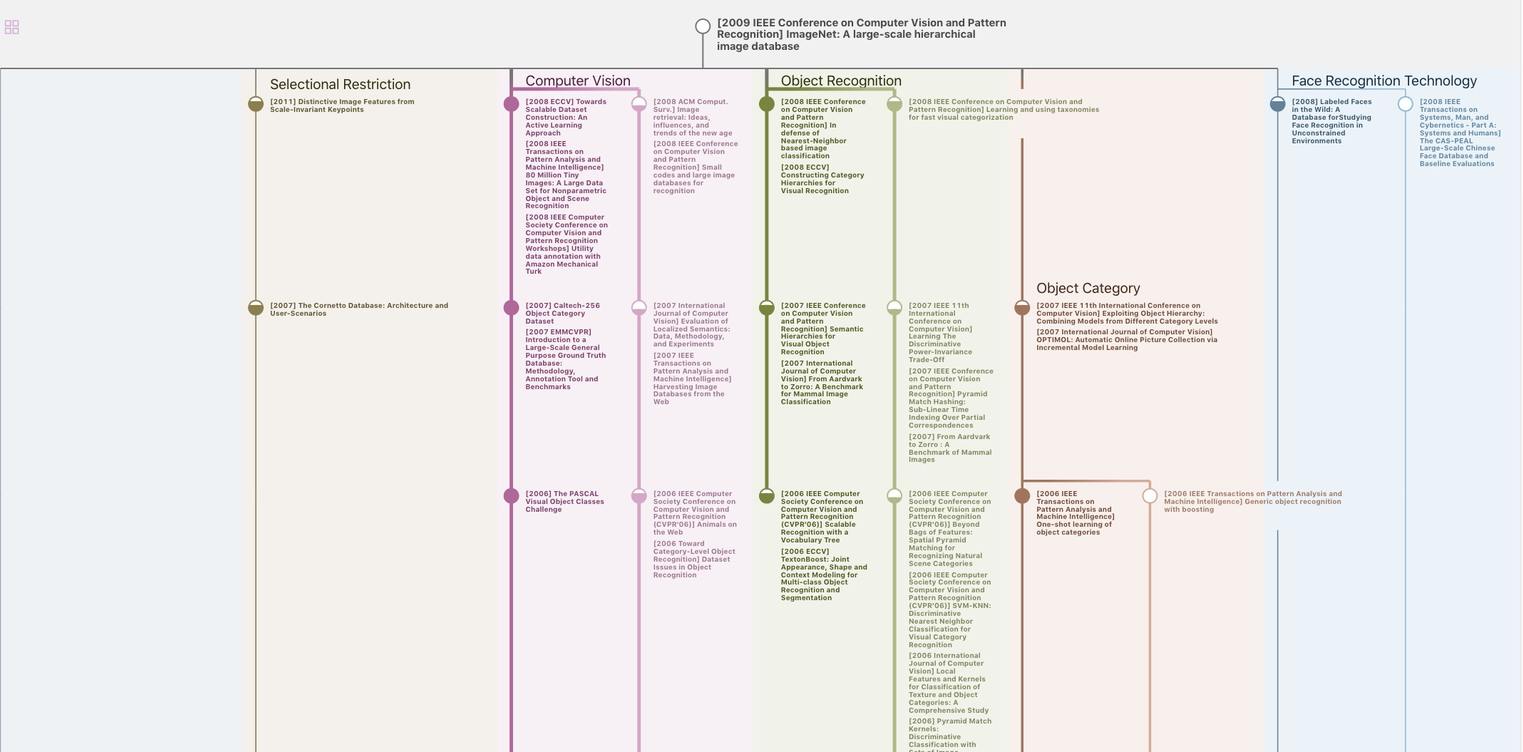
生成溯源树,研究论文发展脉络
Chat Paper
正在生成论文摘要