Learning to Attack Distributionally Robust Federated Learning
semanticscholar(2021)
摘要
We propose a two-stage attack framework that leverages the power of distribution matching and deep reinforcement learning to learn attack policies against federated learning. Our two-stage attack effectively learns an attack policy that minimizes the robustness levels of distributionally robust federated models, and substantially jeopardizes the performance of the federated learning systems even when the server imposes defense mechanisms. Our work brings new insights into how to attack federated learning systems with model-based reinforcement learning.
更多查看译文
AI 理解论文
溯源树
样例
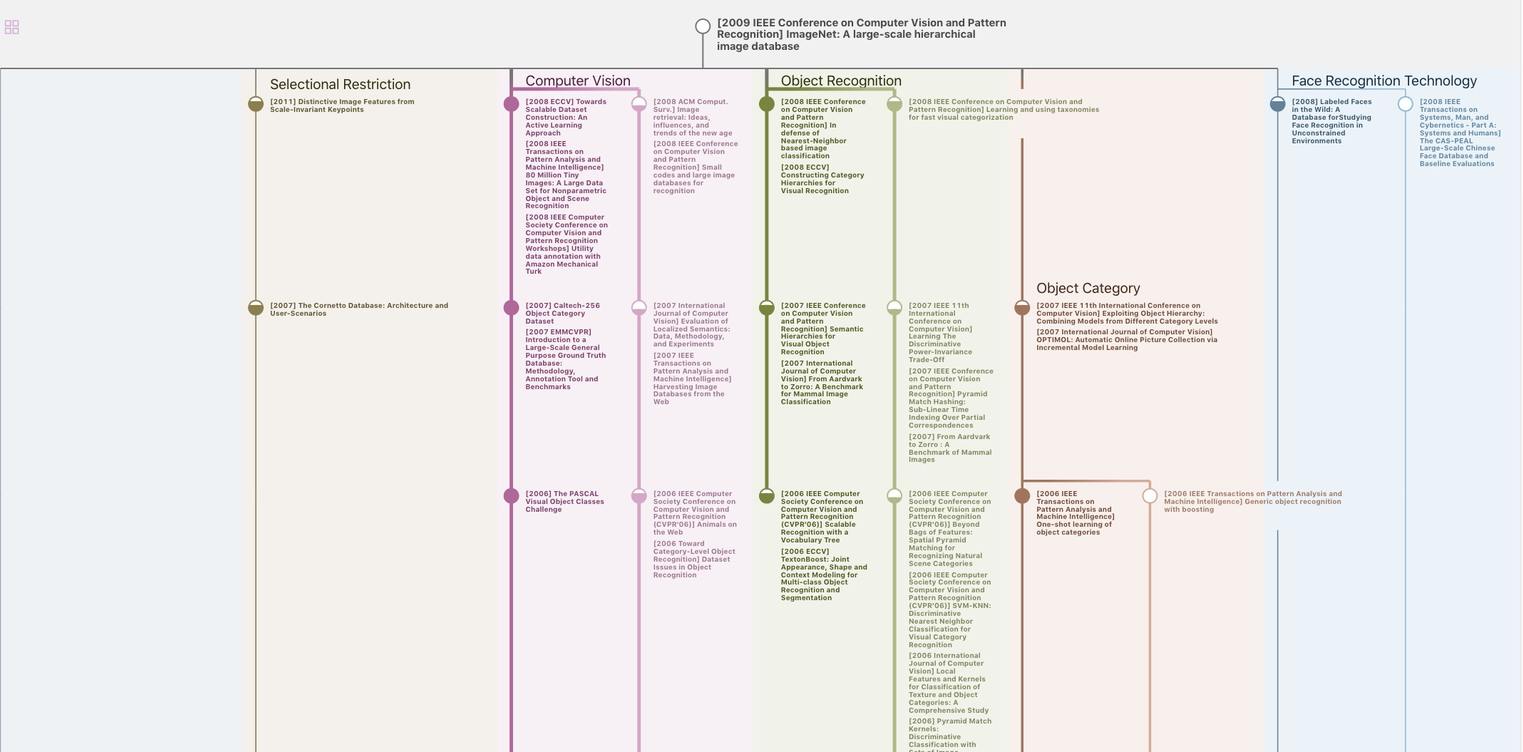
生成溯源树,研究论文发展脉络
Chat Paper
正在生成论文摘要