Combining contextual and neurophysiological information for predicting driver’s turning intent
semanticscholar(2021)
摘要
Turning through oncoming traffic at unsignalized intersections can lead to safety-critical situations contributing to 7.4% of all non-severe vehicle crashes [Harding, 2014]. An important reason for these crashes are human errors in the form of incorrect estimation of the gap size with respect to the oncoming vehicle. Human intention prediction could help reduce the frequency of these safety -critical situations by predicting dangerous turning manoeuvres in advance. Turning behaviour at intersections has been investigated and modelled by several researchers over the past decades (e.g. [Ragland, 2006][Yan, 2007]). Though most research focused on demographic and contextual information, like the gap size in the stream of oncoming vehicles as predictors for the turning intention, [Damm, 2019] presented a case study for how the combination of neurophysiological sensors and contextual information could be used to predict and reflect in low-level control the human’s turning intention to improve safety of turning manoeuvres. The aim of the current study was to enhance the accuracy of the underlying intention prediction beyond that first case-study. It addresses more thoroughly the creation of a turning intention model based on the combination of neurophysiological whole-head fNIRS brain activation measurements and contextual information about the traffic situation recorded during a driving simulator study. We show how the inclusion of fNIRS measurements can increase the performance of such a model in comparison to a context-only intention prediction model.
更多查看译文
AI 理解论文
溯源树
样例
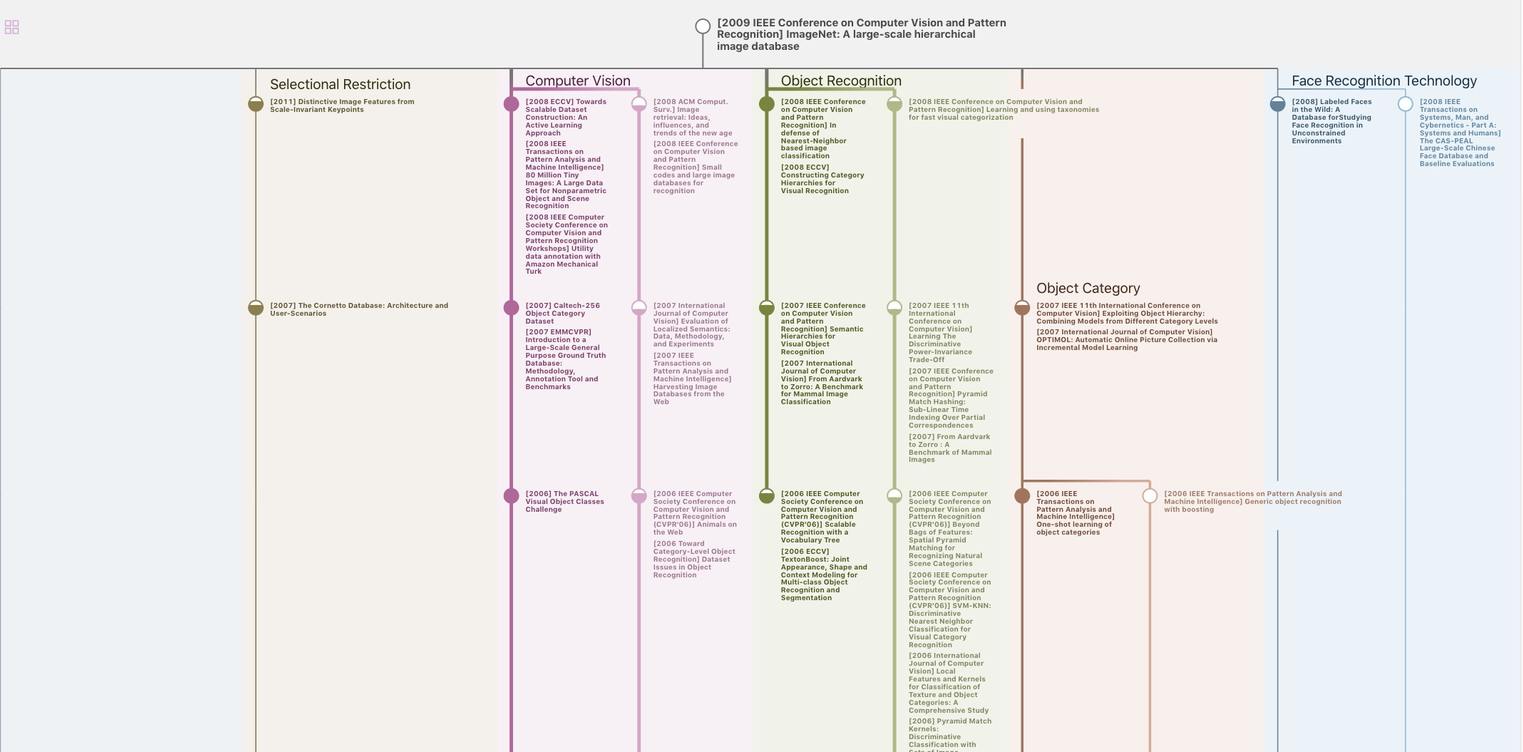
生成溯源树,研究论文发展脉络
Chat Paper
正在生成论文摘要