Development and Implementation of an Early Warning System for High Risk Patients for Suicide Attempt Using Deep Learning and Electronic Health Records (Preprint)
semanticscholar(2018)
摘要
BACKGROUND
Suicide is the 10th leading cause of death in the US. Over the past 15 years, the total suicide rate has increased 24% from 10.5 to 13.0 per 100,000 people. In Massachusetts the rate of death by suicide is three times the rate of homicide deaths. Approximately 60% of suicides die on the first attempt. Of the remaining 40% who survive the index attempt and receive emergency or hospital level of care, rates of subsequent completed suicide are exceptionally high, ranging from 2.3% to 4%. A recent study determined that risk factors for repeat suicide attempt and suicide differed, with alcohol use, younger age and cluster B personality disorders among the attempters and older age and alcohol use among the suicide completers. This data is from a small sample in Catalonia, Spain and whether it is generalizable to populations in the USA is yet to be determined. An early warning system (EWS) for suicide attempt could prove valuable for identifying those at risk of suicide attempts, and the contribution of repeated attempts to the risk of eventual death by suicide.
OBJECTIVE
In this study we sought to develop an early warning system (EWS) for high risk suicide attempt patients through the development of a population-based risk stratification surveillance system. Importantly, this EWS was designed to support case managers, primary care and mental health care practitioners participating in accountable care programs. The continuous use of the system in this program will help assess the ongoing EWS effectiveness.
METHODS
Data from individual patient electronic health records (EHRs) from the Berkshire Health System located in Pittsfield, MA. Advanced machine-learning algorithms and Deep Neural Networks were utilized in the process of feature selection and model building. A final risk score was calculated for each individual and calibrated to indicate the probability of a suicide attempt in the following one-year time period. Risk scores were subjected to individual level analysis in order to aid in the interpretation of the results for health care providers managing the at-risk cohorts.
RESULTS
The one-year suicide attempt risk model attained an area under the curve (AUC) of 0.792 and 0.769 in the retrospective and prospective cohorts, respectively. The suicide attempt rate in the “very high risk” category was 60 times greater than the population baseline when tested in the prospective cohorts, 10 times greater in the “high risk” group, and 5 times greater in the “medium risk” bin. Mental health disorders including depression, bipolar disorders and anxiety, along with substance abuse, impulse control disorders, clinical utilization indicators, and socio-economic determinants were recognized as significant features associated with incident suicide attempt.
CONCLUSIONS
Utilizing a single EHR dataset, an EWS for the determination of risk for suicide attempt was successfully developed and prospectively validated using deep learning modeling techniques.
更多查看译文
AI 理解论文
溯源树
样例
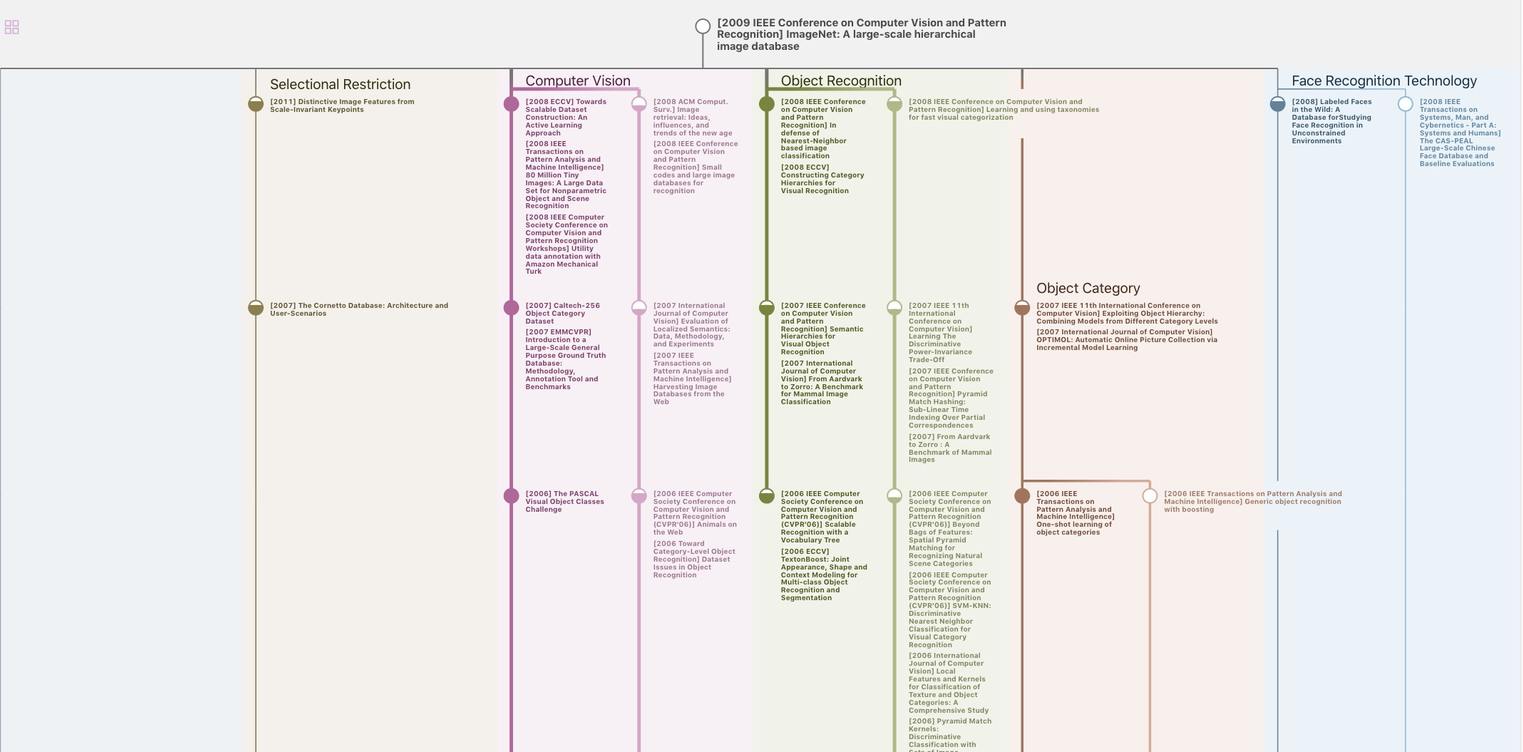
生成溯源树,研究论文发展脉络
Chat Paper
正在生成论文摘要