Emotion recognition in humans and machine using posed and spontaneous facial expression
semanticscholar(2019)
摘要
In the wake of rapid advances in automatic affect analysis, commercial software tools for facial affect recognition have attracted considerable attention in the past few years. While several options now exist to analyze dynamic video data, less is known about the relative performance of these classifiers, in particular when facial expressions are spontaneous rather than posed. In the present work, we tested eight out-of-the-box machine classifiers (Affectiva's Affdex, CrowdEmotion's FaceVideo, Emotient's Facet, Microsoft's Cognitive Services, MorphCast's EmotionalTracking, Neurodatalab's EmotionRecognition, VicarVision's FaceReader and VisageTechnologies' FaceAnalysis), and compared their emotion recognition performance to that of human observers. For this, a total of 938 videos were sampled from two large databases that conveyed the basic six emotions (happiness, sadness, anger, fear, surprise, and disgust) either in posed (BU-4DFE) or spontaneous (UT-Dallas) form. Results revealed a recognition advantage for human observers over machine classification. Among the eight classifiers, there was considerable variance in recognition accuracy ranging from 49 \% to 62 \%. Subsequent analyses per type of expression revealed that performance by the two best performing classifiers approximated those of human observers, suggesting high agreement for posed expressions. However, accuracy was consistently lower for spontaneous affective behavior. Overall, the recognition of happiness was most successful across classifiers and databases, whereas confusion rates suggested system-specific biases favoring classification of certain expressions over others. The findings indicate shortcomings of existing out-of-the-box classifiers for measuring emotions, and highlight the need for more spontaneous facial datasets that can act as a benchmark in the training and testing of automatic emotion recognition systems.
更多查看译文
AI 理解论文
溯源树
样例
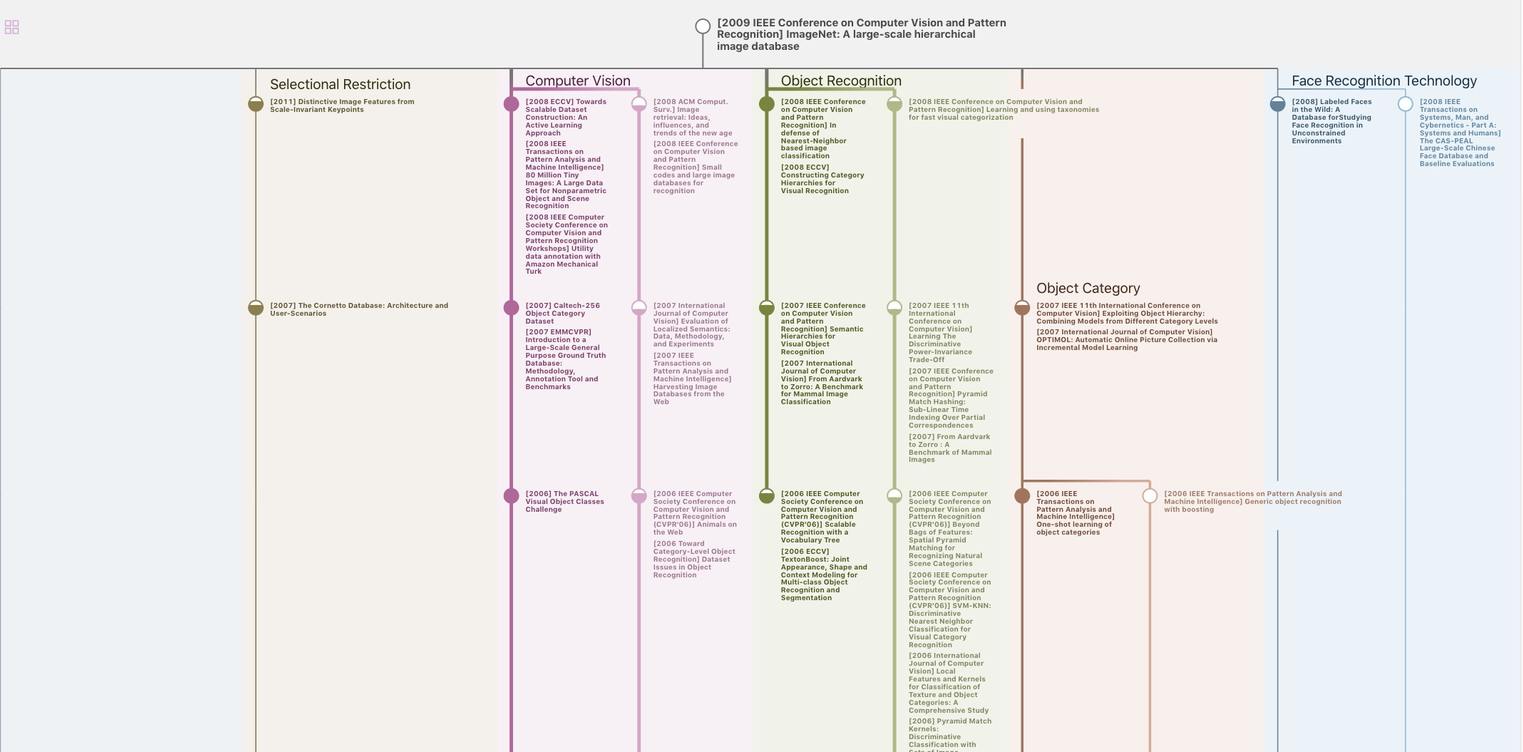
生成溯源树,研究论文发展脉络
Chat Paper
正在生成论文摘要