Finding Al's Faults with AAR/AI An Empirical Study
ACM Transactions on Interactive Intelligent Systems(2022)
摘要
Would you allow an AI agent to make decisions on your behalf? If the answer is "not always;' the next question becomes "in what circumstances"? Answering this question requires human users to be able to assess an AI agent-and not just with overall pass/fail assessments or statistics. Here users need to be able to localize an agent's bugs so that they can determine when they are willing to rely on the agent and when they are not. After-Action Review for AI (AAR/AI), a new AI assessment process for integration with Explainable AI systems, aims to support human users in this endeavor, and in this article we empirically investigate AAR/AI's effectiveness with domain-knowledgeable users. Our results show that AAR/AI participants not only located significantly more bugs than non-AAR/AI participants did (i.e., showed greater recall) but also located them more precisely (i.e., with greater precision). In fact, AAR/AI participants outperformed nonAAR/AI participants on every bug and were, on average, almost six times as likely as non-AAR/AI participants to find any particular bug. Finally, evidence suggests that incorporating labeling into the AAR/AI process may encourage domain-knowledgeable users to abstract above individual instances of bugs; we hypothesize that doing so may have contributed further to AAR/AI participants' effectiveness.
更多查看译文
关键词
AAR/AI, after-action review for AI, explainable AI (XAI)
AI 理解论文
溯源树
样例
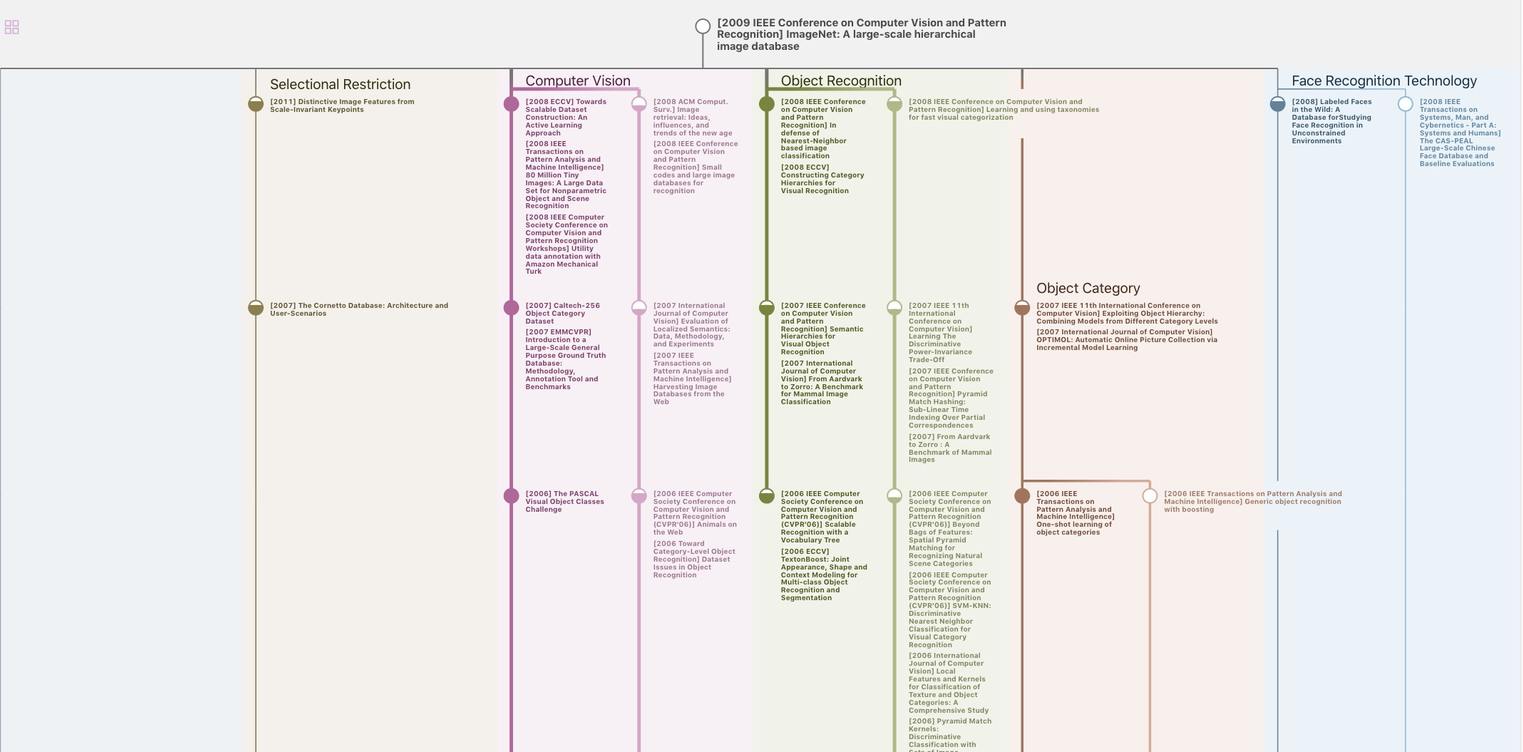
生成溯源树,研究论文发展脉络
Chat Paper
正在生成论文摘要