Ein eLearning Tool zur Identifizierung von Population, Intervention, Vergleich und Ergebnis für Medizinstudierende
semanticscholar(2020)
摘要
Medical research presents its results in the form of published papers in scientific journals. One of the most important online platforms for researching published papers is PubMed, in which the abstracts of all articles are published even before their official publication. However, the use of this data in evidence-based medicine is still an extremely timeconsuming task, as the structure of abstracts can vary between different study designs, disciplines and research groups greatly. As of 2019 PubMed contained 5.7 million articles with a current growth rate of approximately 500,000 documents per year. Even if modern search engines work better than in the past, it is still necessary, that the researcher at least reads the abstracts of all potentially relevant articles. Population, Interaction, Comparison, Outcome (PICO) are basic elements of medical studies. The use of machine learning is a state-of-the-art method for label predictions in texts. Such a model learns to perform annotations on the basis of already annotated texts (data set). An example of such a data set with annotations of PICO elements in medical texts is ebmnlp, which has been used for comparison several times in this paper. The basis of this work was the data set already available from the KConnect project consisting of abstracts of medical studies with already annotated PICO elements. The annotation tool developed for the KConnect project was extended to an eLearning system, with which further abstracts could be annotated (picoweb data set). To simplify things for the users, Intervention and Comparison were combined to form Therapy. In the next step a neural network (SciBERT) was trained with the picoweb data set. This achieved f1-scores of 0.87 for the detection of Population and 0.83 for Therapy. For comparison, the same model was trained with the ebmnlp data set, which achieved an f1-score of 0.81 for Population and 0.72 for Therapy.
更多查看译文
AI 理解论文
溯源树
样例
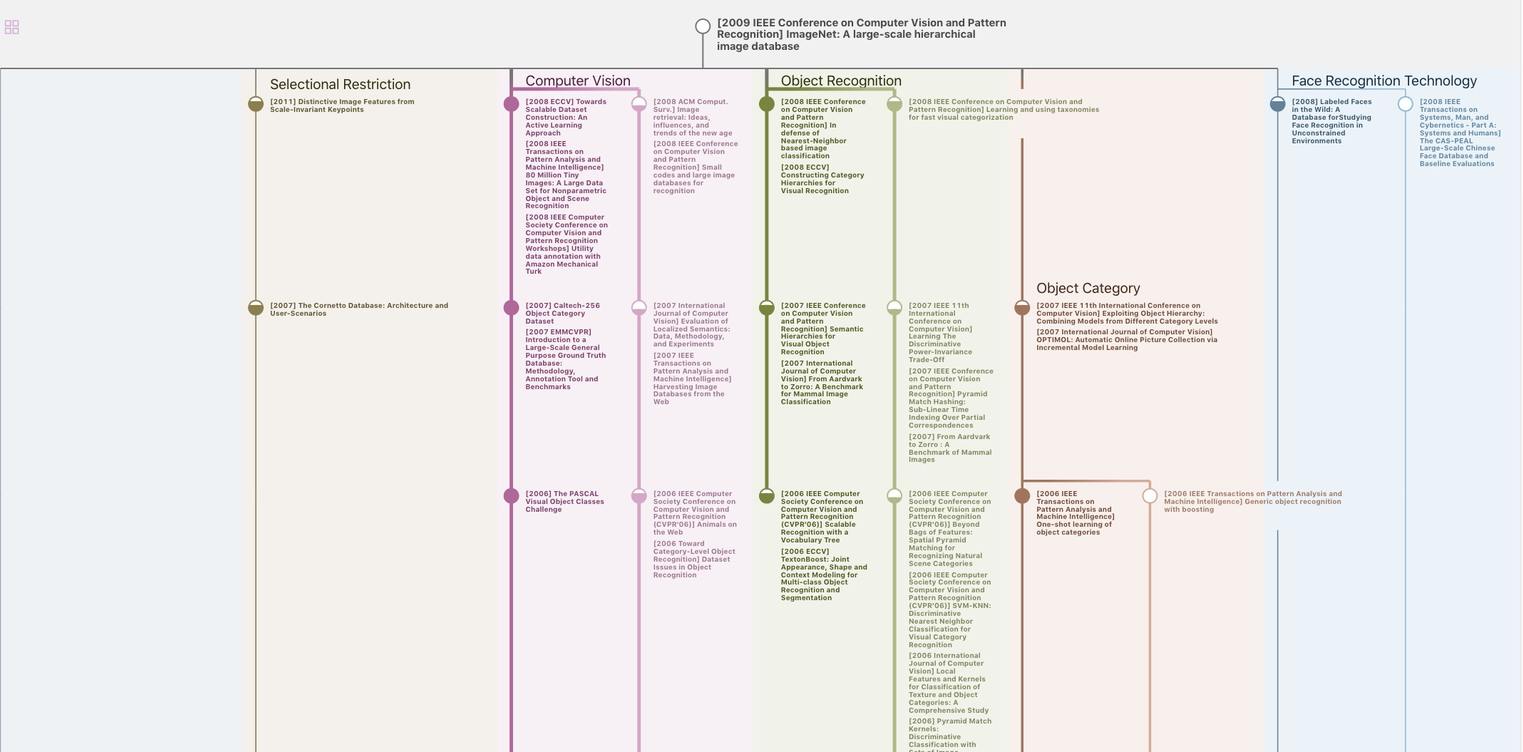
生成溯源树,研究论文发展脉络
Chat Paper
正在生成论文摘要