Reformed QANet-Optimizing the Spatial Complexity of QANet
semanticscholar(2021)
摘要
The feed-forward QANet architecture replaced the bidirectional LSTMs of traditional Q&A models’ encoder components with convolution + self-attention to increase the speed of the model without sacrificing accuracy [1]. We achieved scores of 64.5 EM/67.9 F1 on the dev set and 61.64 EM/65.30 F1 on the test set. While the parallel nature of QANet’s CNN architecture allows for a significant speed boost, it entails GPU memory requirements to reap those benefits. We preform an ablation study to measure changes to spatial complexity, speed, and performance on the QANet architecture, replacing the self attention and feed-forward layer with LSH attention, reversible residual networks, and an entire reformer. We found that implementing LSH attention successfully decreased memory usage while maintaining reasonable performance. While the other modifications did not quite maintain the original QANet model’s EM and FI scores, they significantly improved memory complexity. 1 Key Information to include External collaborators: None, Mentor: Mandy Lu, Sharing project: False
更多查看译文
AI 理解论文
溯源树
样例
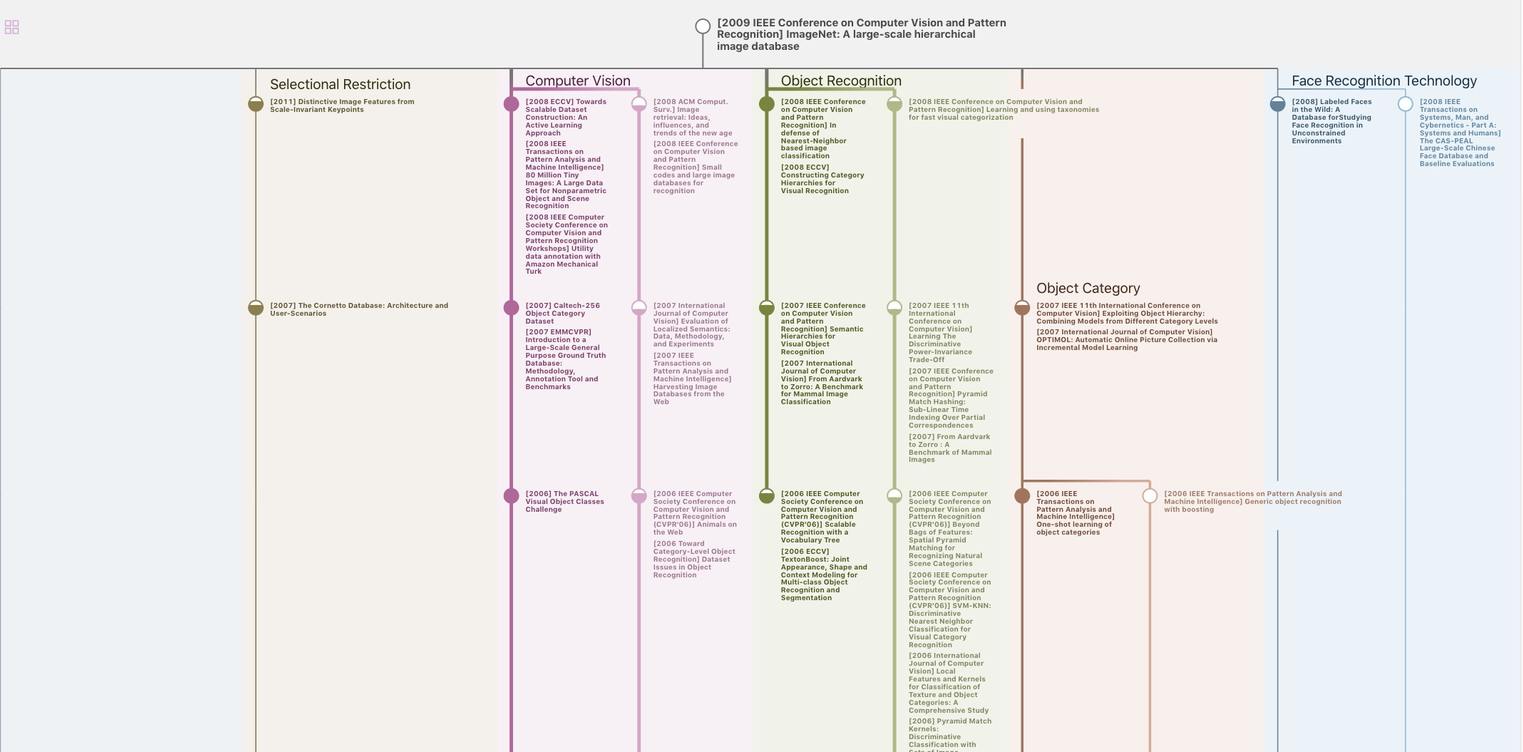
生成溯源树,研究论文发展脉络
Chat Paper
正在生成论文摘要