On the Parameterized Complexity of Learning First-Order Logic
PROCEEDINGS OF THE 41ST ACM SIGMOD-SIGACT-SIGAI SYMPOSIUM ON PRINCIPLES OF DATABASE SYSTEMS (PODS '22)(2022)
摘要
We analyse the complexity of learning first-order queries in a model-theoretic framework for supervised learning introduced by (Grohe and Turan, TOCS 2004). Previous research on the complexity of learning in this framework focussed on the question of when learning is possible in time sublinear in the background structure. Here we study the parameterized complexity of the learning problem. We have two main results. The first is a hardness result, showing that learning first-order queries is at least as hard as the corresponding model-checking problem, which implies that on general structures it is hard for the parameterized complexity class AW[*]. Our second main contribution is a fixed-parameter tractable agnostic PAC learning algorithm for first-order queries over sparse relational data (more precisely, over nowhere dense background structures).
更多查看译文
关键词
first-order query learning, agnostic probably approximately correct learning, nowhere dense graph, parameterized complexity, fixed-parameter tractable, Boolean classification, supervised learning, first-order logic
AI 理解论文
溯源树
样例
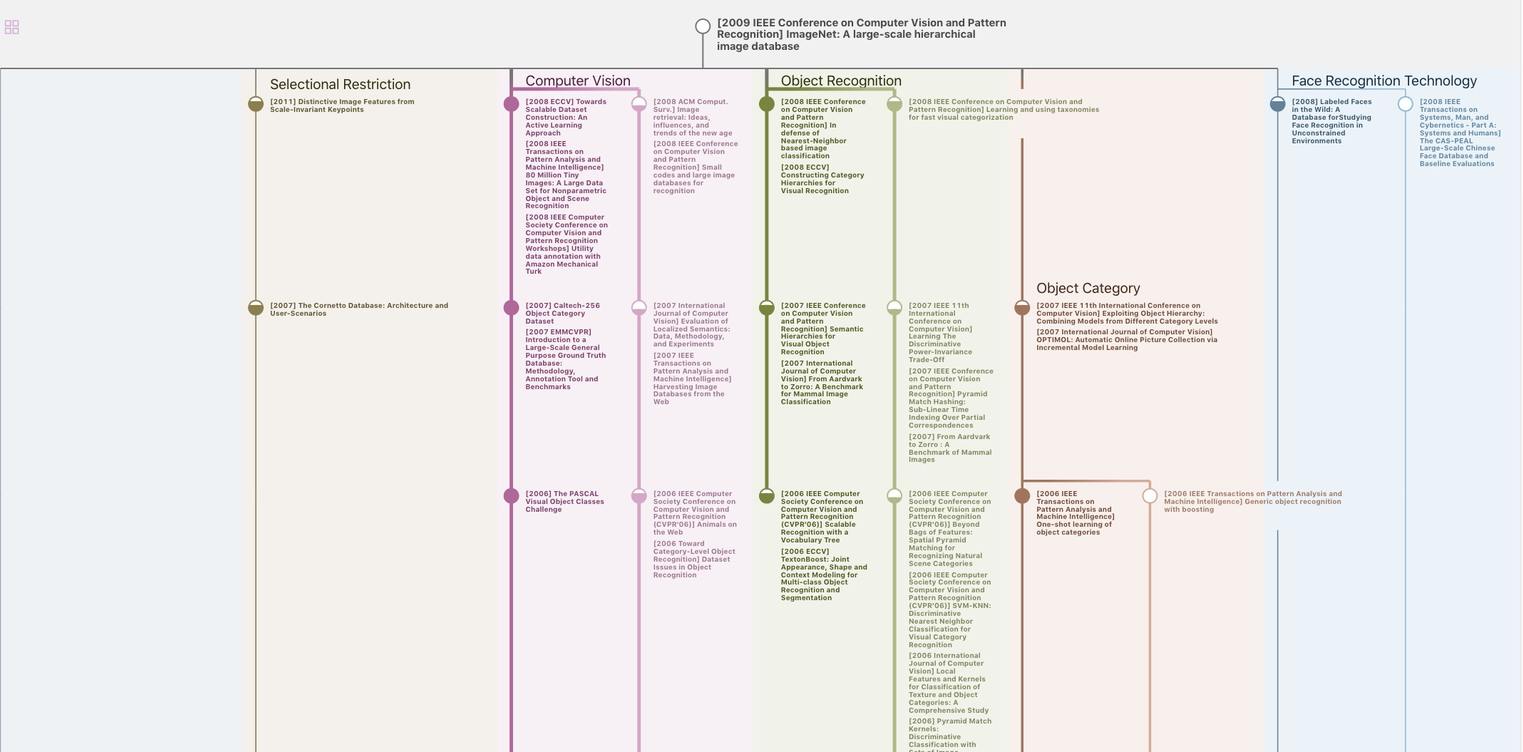
生成溯源树,研究论文发展脉络
Chat Paper
正在生成论文摘要