A Data Change Rule Based Empirical Framework for Labeling Unlabeled Time Series Driving Data
semanticscholar(2020)
摘要
Most of the driving maneuver classification methods
follow supervised learning techniques and utilize ground truth in order to train classifiers. However, collecting ground truth is the most troublesome, expensive, and significant task of classification and effects a classifier’s performance. The work proposes an empirical framework for automatic labeling of timeseries data that can be further used in training phrases during semi-supervised learning. The proposed algorithm generates class labels and find that generated label of 4895 data matched with 11077 manual labeled data. The work analyzes the challenges involved in the driving time series data labeling. So, reasons behind mismatch of data label can also be explained.
更多follow supervised learning techniques and utilize ground truth in order to train classifiers. However, collecting ground truth is the most troublesome, expensive, and significant task of classification and effects a classifier’s performance. The work proposes an empirical framework for automatic labeling of timeseries data that can be further used in training phrases during semi-supervised learning. The proposed algorithm generates class labels and find that generated label of 4895 data matched with 11077 manual labeled data. The work analyzes the challenges involved in the driving time series data labeling. So, reasons behind mismatch of data label can also be explained.
查看译文
AI 理解论文
溯源树
样例
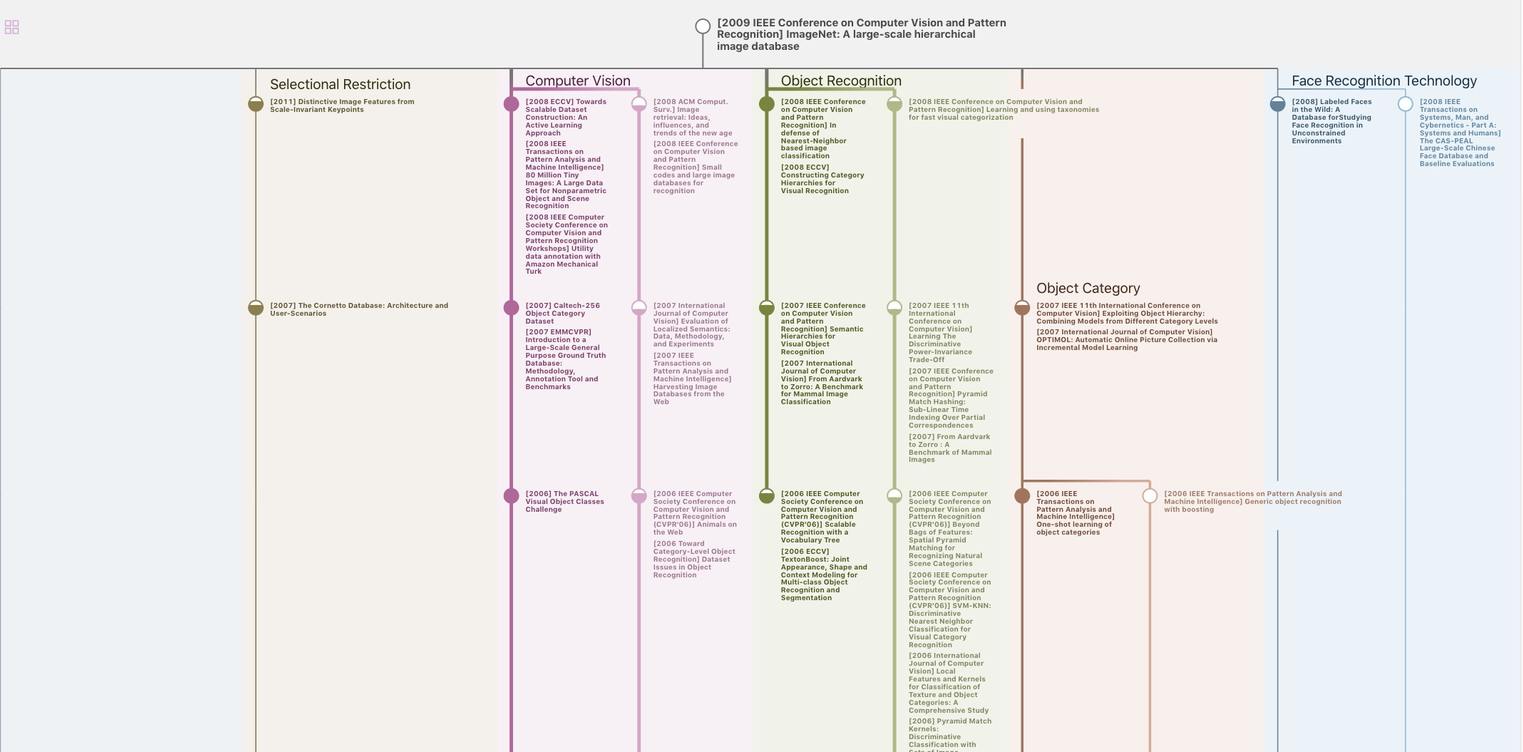
生成溯源树,研究论文发展脉络
Chat Paper
正在生成论文摘要