Make Aspect-Based Sentiment Classification Go Further: Step into the Long-Document-level
Applied Intelligence(2021)
Key words
Aspect-based sentiment analysis,Hierarchical architecture,Data transformation,Long document
AI Read Science
Must-Reading Tree
Example
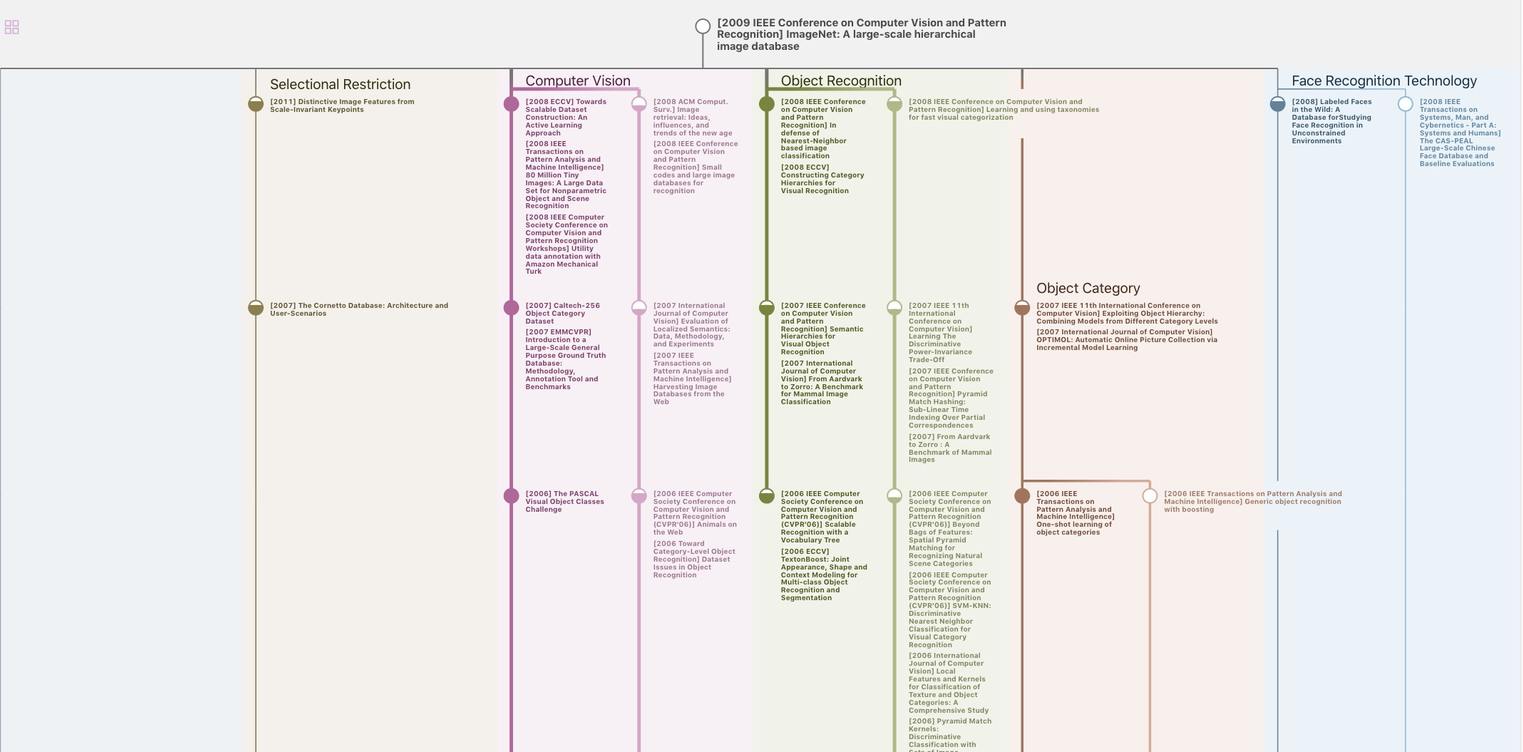
Generate MRT to find the research sequence of this paper
Chat Paper
Summary is being generated by the instructions you defined