Optimum Application of Hybrid Data Driven Models With Two Step Verification Method at Mangla Watershed, Pakistan
semanticscholar(2021)
摘要
In this study, 03 ensemble and decomposition methods (DMs) i.e., empirical mode decomposition (EMD), ensemble empirical mode decomposition (EEMD) and improved complete ensemble empirical mode decomposition with additive noise (ICEEMDAN) were coupled artificial intelligence and machine learning based method AI-ML, i.e., multilayer perceptron (MLP), support vector regression (SVR) to develop 06 fundamental hybrid models to predict streamflow with one-month lead time. Developed models in this study were categorized into runoff models (RMs) and rainfall-runoff models (RRMs). Results indicated that (i) among standalone models (SMs), support vector regression (SVR) performs better than multilayer perceptron (MLP), (ii) decomposition methods (DMs) have ability to improve the accuracy rate of the standalone models (SMs) and, (iii) rainfall runoff models (RRMs) have shown great accuracy throughout the investigation as compared to runoff models (RMs). To compare model performances flow-hydrographs (FHG) were generated, 05 performance evaluation criteria (PEC) were used to quantify the model precision. Two step verification method i.e., extreme value analysis (EVA) and least value analysis (LVA) approaches were proposed to verify the performances. Among all developed hybrid models (HMs), i.e., EMD- (MLP, SVR), EEMD- (MLP, SVR) and ICEEMDAN- (MLP, SVR), rainfall-runoff ICEEMDAN-(SVR) model was selected as optimal model with MAE (59.56), RMSE (91.82), R (0.97) MAPE (8.75), and NSEC (0.97) for Mangla watershed, Pakistan.
更多查看译文
关键词
hybrid data driven models,mangla watershed,step verification method
AI 理解论文
溯源树
样例
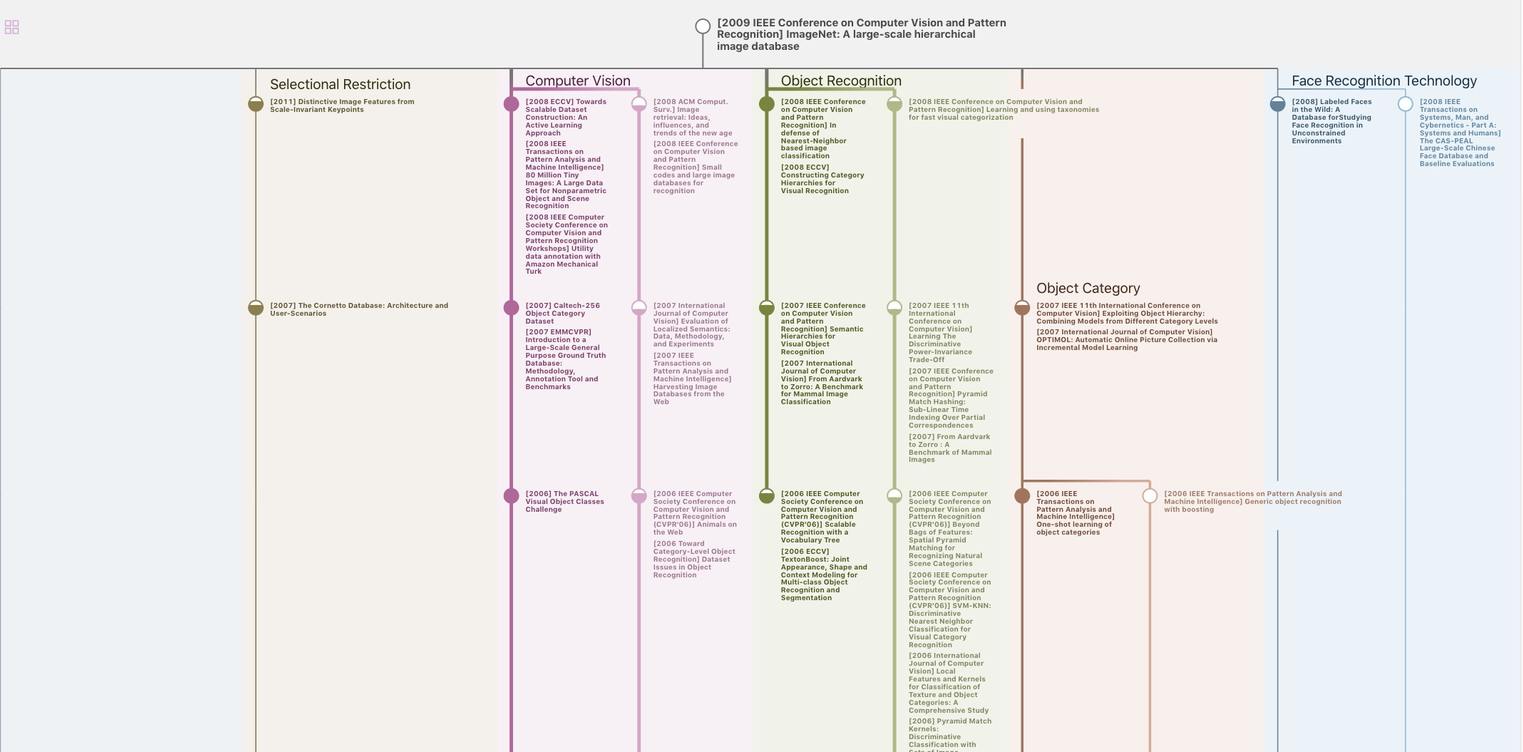
生成溯源树,研究论文发展脉络
Chat Paper
正在生成论文摘要