SalemGarcia_2021
semanticscholar(2021)
Abstract
We systematically misjudge our own performance in simple economic tasks. First, we generally overestimate our ability to make correct choices – a bias called overconfidence. Second, we are more confident in our choices when we seek gains than when we try to avoid losses – a bias we refer to as the valence-induced confidence bias. Strikingly, these two biases are also present in reinforcement-learning contexts, despite the fact that outcomes are provided trial-by-trial and could, in principle, be used to recalibrate confidence judgments online. How confidence biases emerge and are maintained in reinforcement-learning contexts is thus puzzling and still unaccounted for. To explain this paradox, we propose that confidence biases stem from learning biases, and test this hypothesis using data from five experiments, where we concomitantly assessed instrumental choices and confidence judgments, during learning and transfer phases. Our results first show that participants’ choices in both tasks are best accounted for by a reinforcement-learning model featuring context-dependent learning and confirmatory update. We then demonstrate that the complex, biased pattern of confidence judgments elicited during both tasks can be explained by an overweighting of the learned value of the chosen option in the computation of confidence judgments. We finally show that, consequently, the individual learning-model parameters responsible for the learning biases – confirmatory updating and outcome context-dependency – are predictive of the individual metacognitive biases. We conclude suggesting that the metacognitive biases originate from fundamentally biased learning computations.
MoreTranslated text
AI Read Science
Must-Reading Tree
Example
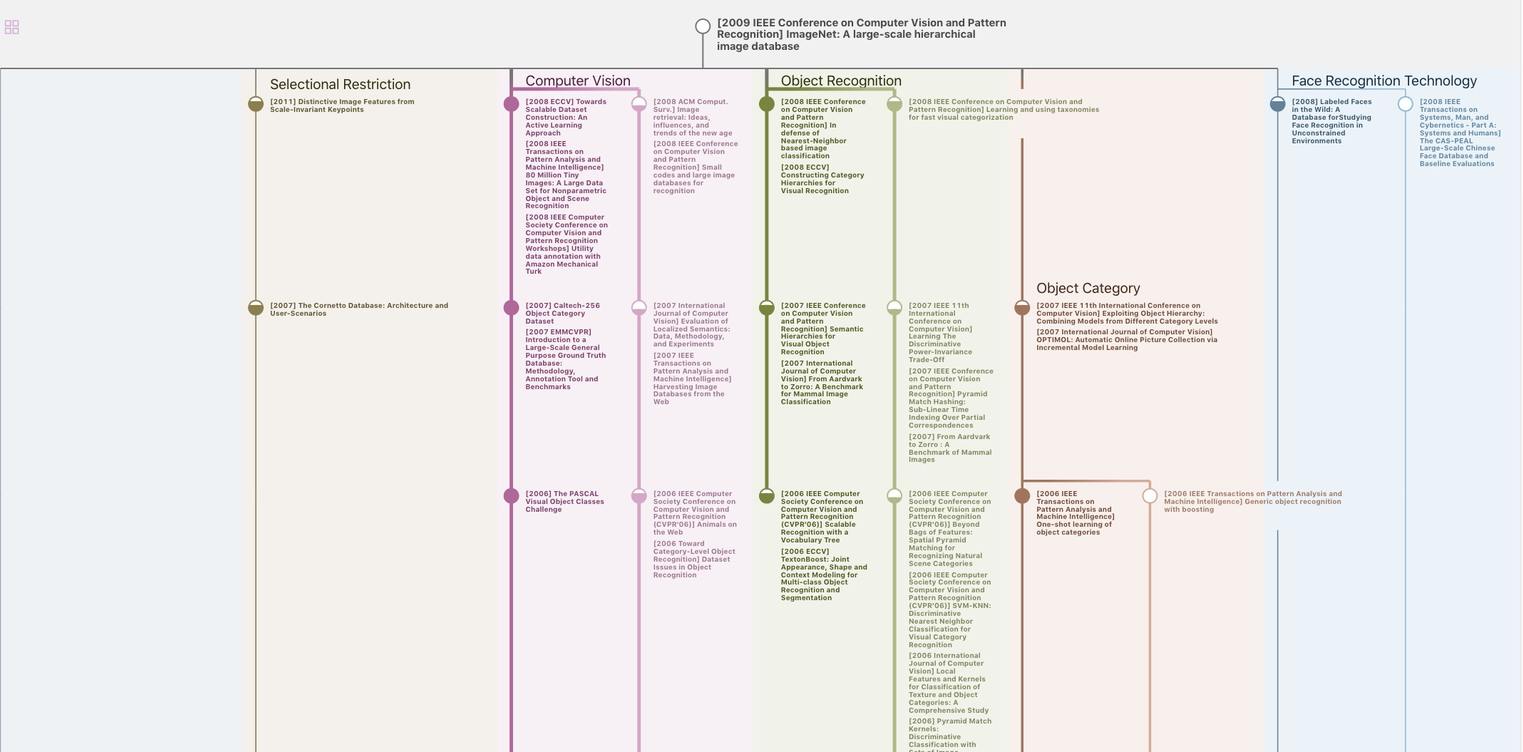
Generate MRT to find the research sequence of this paper
Chat Paper
Summary is being generated by the instructions you defined