DUMP: A Dummy-point-based Local Differential Privacy Enhancement Approach under the Shuffle Model
semanticscholar(2021)
摘要
The shufflemodel is recently proposed to address the issue of severe utility loss in Local Differential Privacy (LDP) due to distributed data randomization. In the shuffle model, a shuffler is utilized to break the link between the user identity and the message uploaded to the data analyst. Since less noise needs to be introduced to achieve the same privacy guarantee, following this paradigm, the utility of privacy-preserving data collection is improved. In this paper, we propose DUMP (DUMmy-Point-based), a new framework for privacy-preserving histogram estimation in the shuffle model. In DUMP, dummy messages are introduced on the user side, creating an additional dummy blanket to further improve the utility of the shuffle model. In addition, this new privacy-preserving mechanism also achieves LDP privacy amplification effect to the user uploaded data against a curious shuffler. We instantiate DUMP by proposing two protocols: pureDUMP and mixDUMP, both of which achieving theoretical improvements on accuracy and communication complexity comparing with existing protocols. A comprehensive experimental evaluation is conducted to compare the proposed two designs under DUMP with the existing protocols. Experimental results on both synthetic and real-world datasets confirm our theoretical analysis and show that the proposed protocols achieve better utility than existing protocols under the same privacy guarantee. PVLDB Reference Format: Xiaochen Li, Weiren Liu, Hanwen Feng, Kunzhe Huang, Yuke Hu, Jinfei Liu, Kui Ren, and Zhan Qin. DUMP: A Dummy-point-based Local Differential Privacy Enhancement Approach under the Shuffle Model. PVLDB, 14(1): XXX-XXX, 2020. doi:XX.XX/XXX.XX PVLDB Artifact Availability: This work is licensed under the Creative Commons BY-NC-ND 4.0 International License. Visit https://creativecommons.org/licenses/by-nc-nd/4.0/ to view a copy of this license. For any use beyond those covered by this license, obtain permission by emailing info@vldb.org. Copyright is held by the owner/author(s). Publication rights licensed to the VLDB Endowment. Proceedings of the VLDB Endowment, Vol. 14, No. 1 ISSN 2150-8097. doi:XX.XX/XXX.XX The source code, data, and/or other artifacts have been made available at URL_TO_YOUR_ARTIFACTS.
更多查看译文
AI 理解论文
溯源树
样例
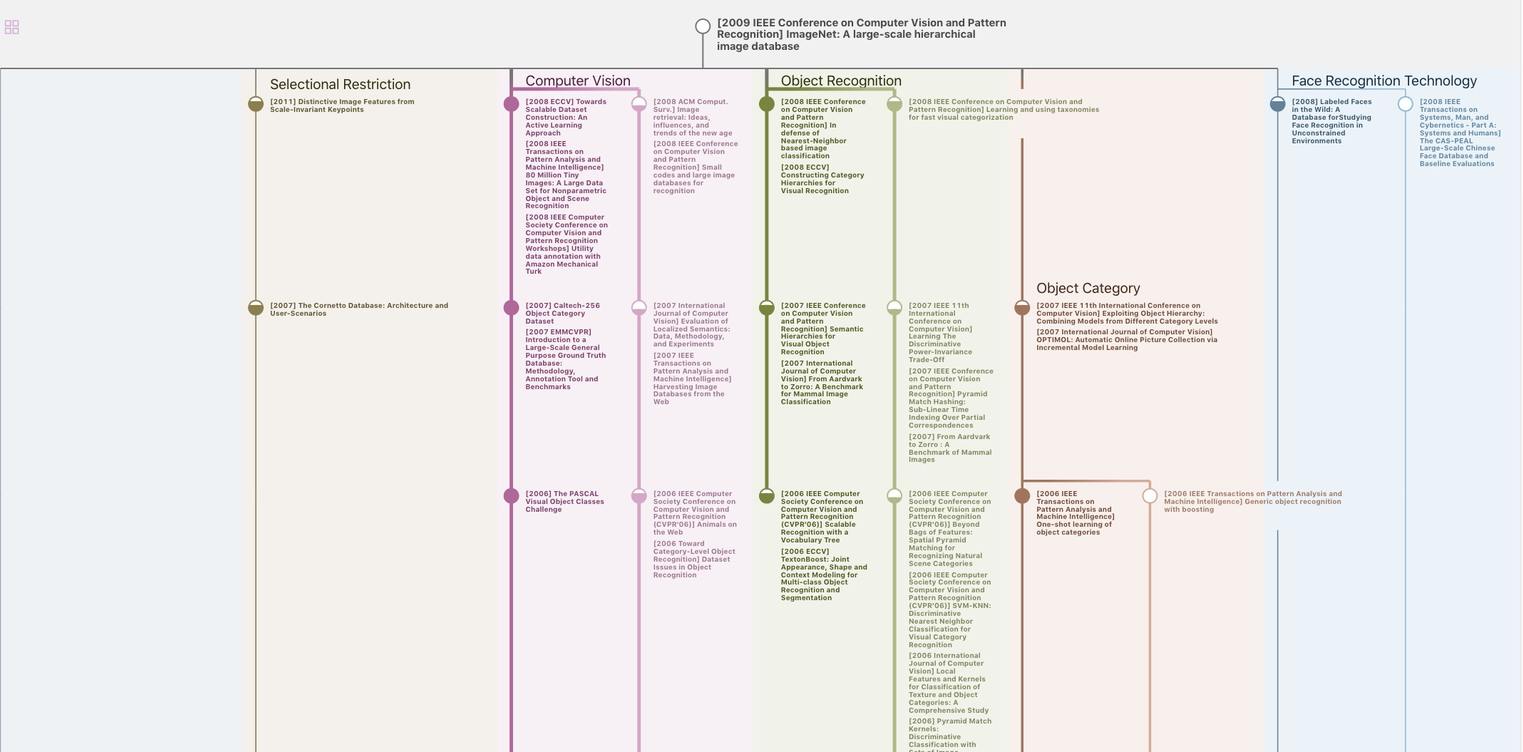
生成溯源树,研究论文发展脉络
Chat Paper
正在生成论文摘要