Survey of Disparity Map Algorithms Intended for Real Time Stereoscopic Depth Estimation
semanticscholar(2021)
摘要
Autonomous vehicles rely on depth estimates derived from several sensor modalities for navigation and object detection. Stereoscopic depth estimation infers real-world distance to objects under a given target pixel from the displacement of the corresponding pixel in the other image of the stereo pair and known baseline distance between cameras. This approach provides dense depth estimates with inexpensive equipment that can be used to augment cheaper sensors to reduce overall system cost while maintaining good performance. However, estimating the correspondence of pixels between images in stereo pairs to establish relative displacement is computationally expensive. While traditional realtime disparity algorithms typically sacrifice accuracy for speed, new deep-learning models promise to retain accuracy while achieving real-time performance on dedicated hardware. This article evaluates two such algorithms, DeepPruner from Uber Research and the Pyramid Stereo Matching Network by Chang et al, which have ranked well in the KITTI Stereo competition. The traditional Stereo Block Matching Algorithm packaged in OpenCV is used as a baseline for comparison. It was found that all three algorithms performed well on simple stereo pairs from the 2001 dataset while DeepPruner performed the best on complex scenes followed by PSMNet.
更多查看译文
AI 理解论文
溯源树
样例
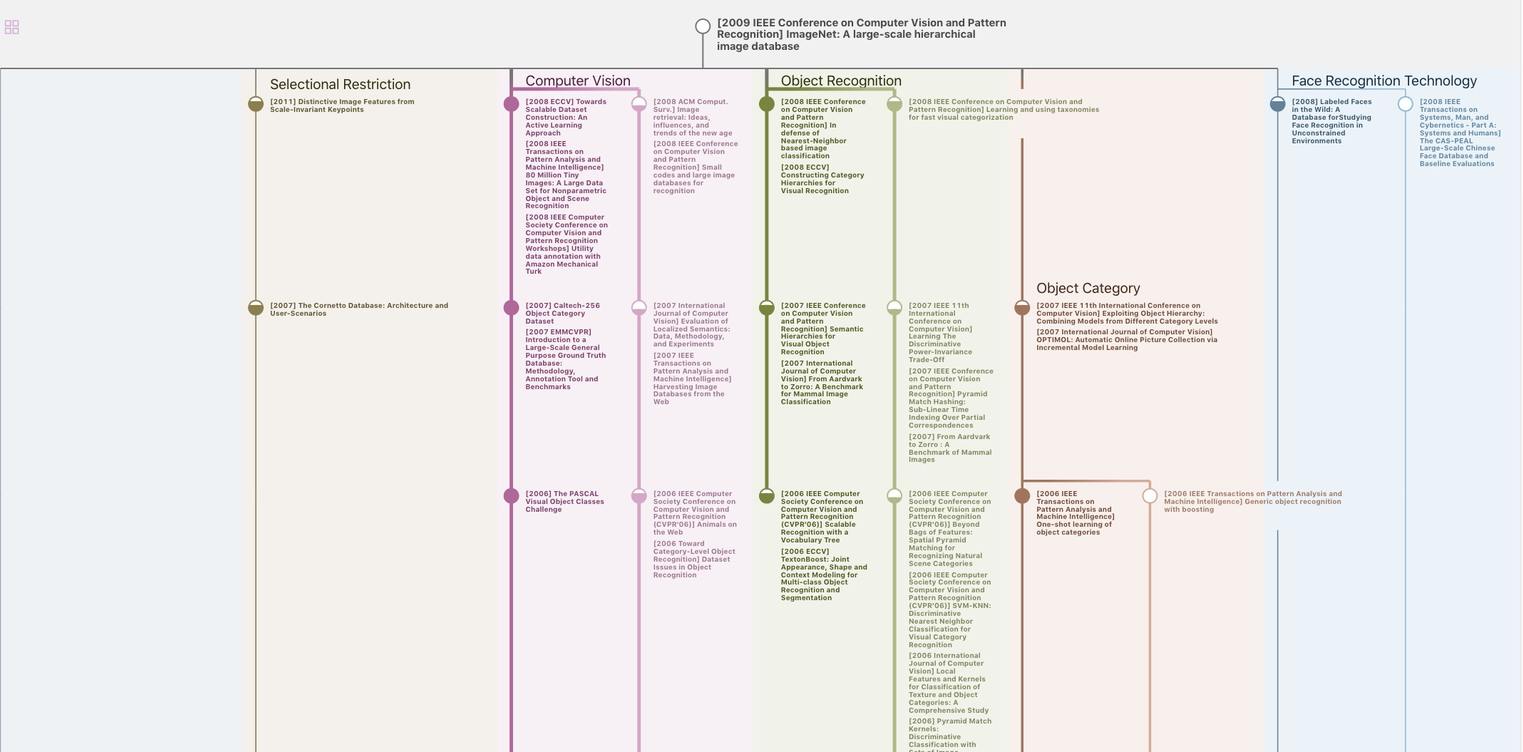
生成溯源树,研究论文发展脉络
Chat Paper
正在生成论文摘要