A Flexible Data-Driven Framework for COVID-19 Case Forecasting Deployed in a Developing-world Public Health Setting
medRxiv(2021)
摘要
Forecasting infection case counts and estimating accurate epidemiological parameters are critical components of managing the response to a pandemic. This paper describes a modular, extensible framework for a COVID-19 forecasting system, primarily deployed in Mumbai and Jharkhand, India. We employ a variant of the SEIR compartmental model motivated by the nature of the available data and operational constraints. We estimate best-fit parameters using sequential Model-Based Optimization (SMBO) and describe the use of a novel, fast, and approximate Bayesian model averaging method (ABMA) for parameter uncertainty estimation that compares well with a more rigorous Markov Chain Monte Carlo (MCMC) approach in practice. We address on-the-ground deployment challenges such as spikes in the reported input data using a novel weighted smooth-ing method. We describe extensive empirical analyses to evaluate the accuracy of our method on ground truth as well as against other state-of-the-art approaches. Finally, we outline deployment lessons and describe how inferred model parameters were used by government partners to interpret the state of the epidemic and how model forecasts were used to estimate staffing and planning needs essential for addressing COVID-19 hospital burden.
更多查看译文
AI 理解论文
溯源树
样例
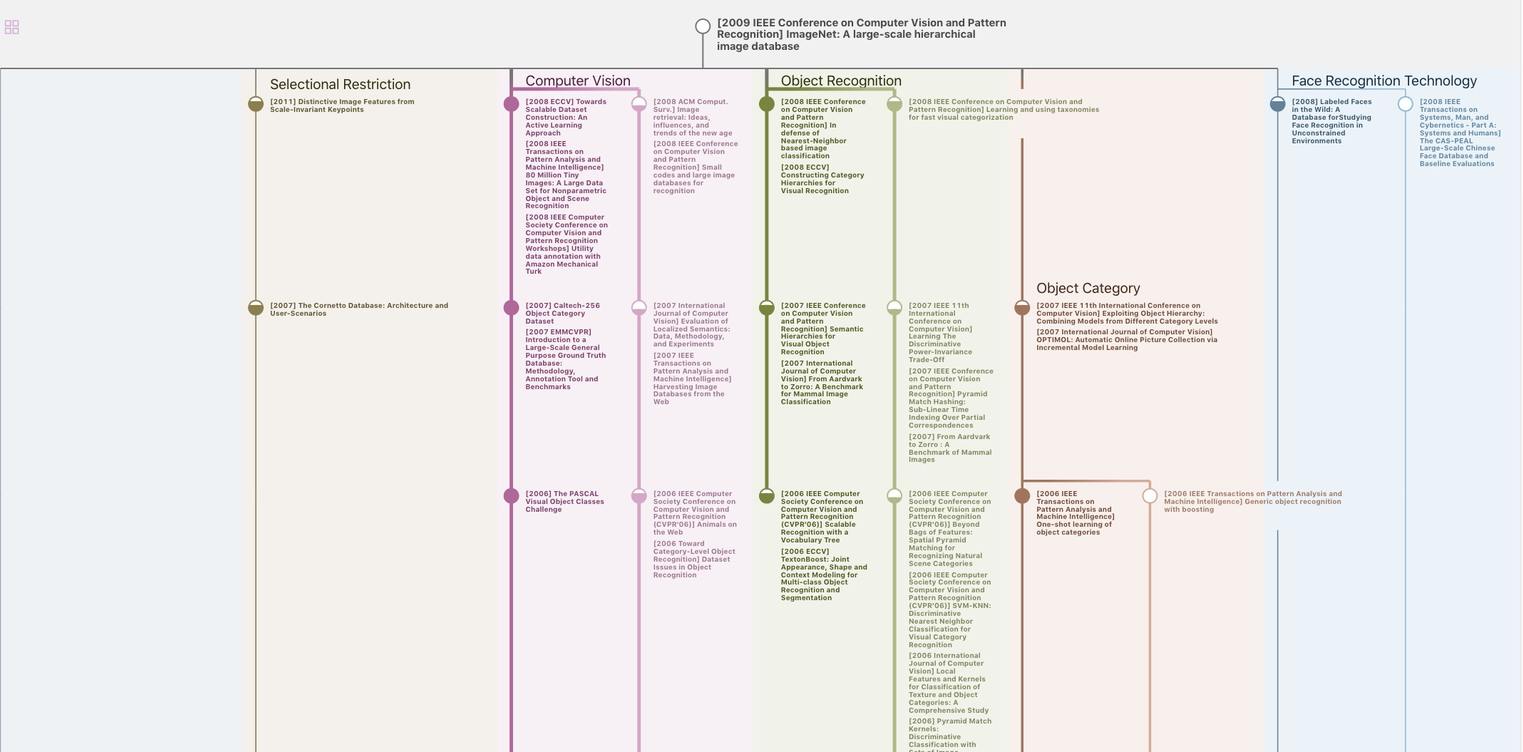
生成溯源树,研究论文发展脉络
Chat Paper
正在生成论文摘要