Application of Deep Knockoffs for fMRI to Generate Surrogate Data
semanticscholar(2021)
摘要
The study of neural structure and activity is a promising field for a better understanding of our brain, as well as for diagnostics of brain disorders. Functional Magnetic Resonance Imaging (fMRI) is one of the techniques used to detect active brain regions while performing tasks, i.e. selecting significant regions. Recently, a new procedure to select significant features, called Knockoff filtering, gained attention. In this paper, we apply the knockoff framework to generate surrogate data and perform non-parametric tests using the surrogate data to build brain activation maps. We implement three different knockoff frameworks, evaluate their quality, and compare the resulting activation maps to the ones produced using parametric testing from a general linear model (GLM), the standard method to generate activation maps. Our results show that the activation maps built with the knockoff framework are comparable to the ones created by the GLM. These results open the door for further investigations of the knockoff framework on fMRI data.
更多查看译文
AI 理解论文
溯源树
样例
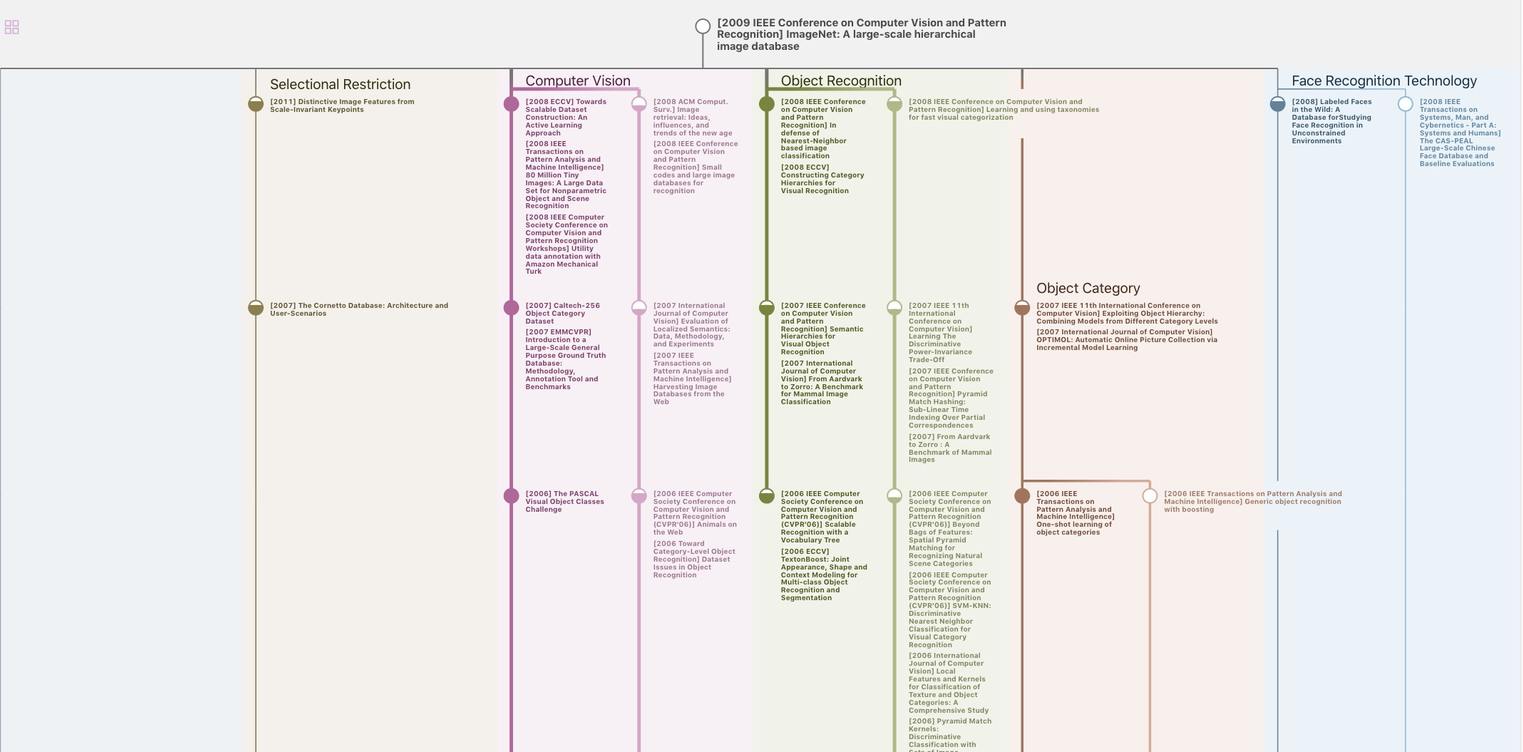
生成溯源树,研究论文发展脉络
Chat Paper
正在生成论文摘要