A New Methodology to Produce More Skillful United States Cool-Season Precipitation Forecasts
JOURNAL OF HYDROMETEOROLOGY(2022)
摘要
The water resources of the western United States have enormous agricultural and municipal demands. At the same time, droughts like the one enveloping the West in the summer of 2021 have disrupted supply of this strained and precious resource. Historically, seasonal forecasts of cool-season (November-March) precipitation from dynamical models such as North American Multi-Model Ensemble (NMME) and the Seasonal Forecasting System 5 (SEAS5) from the European Centre for Medium-RangeWeather Forecasts have lacked sufficient skill to aid inWestern stakeholders' and water managers' decision-making. Here, we propose a new empirical-statistical framework to improve cool-season precipitation forecasts across the contiguous United States (CONUS). This newly developed framework is called the Statistical Climate Ensemble Forecast (SCEF) model. The SCEF framework applies a principal component regression model to predictors and predictands that have undergone dimensionality reduction, where the predictors are large-scale meteorological variables that have been prefiltered in space. The forecasts of the SCEF model captures 12.0% of the total CONUS-wide standardized observed variance over the period 1982/83-2019/20, whereas NMME captures 7.2%. Over the more recent period 2000/01-2019/20, the SCEF, NMME, and SEAS5 models respectively capture 11.8%, 4.0%, and 4.1% of the total CONUS-wide standardized observed variance. An important finding is that much of the improved skill in the SCEF, with respect to models such as NMME and SEAS5, can be attributed to better forecasts across most of the western United States.
更多查看译文
关键词
ENSO, Hydrology, Hydrometeorology, Seasonal forecasting, Statistical forecasting
AI 理解论文
溯源树
样例
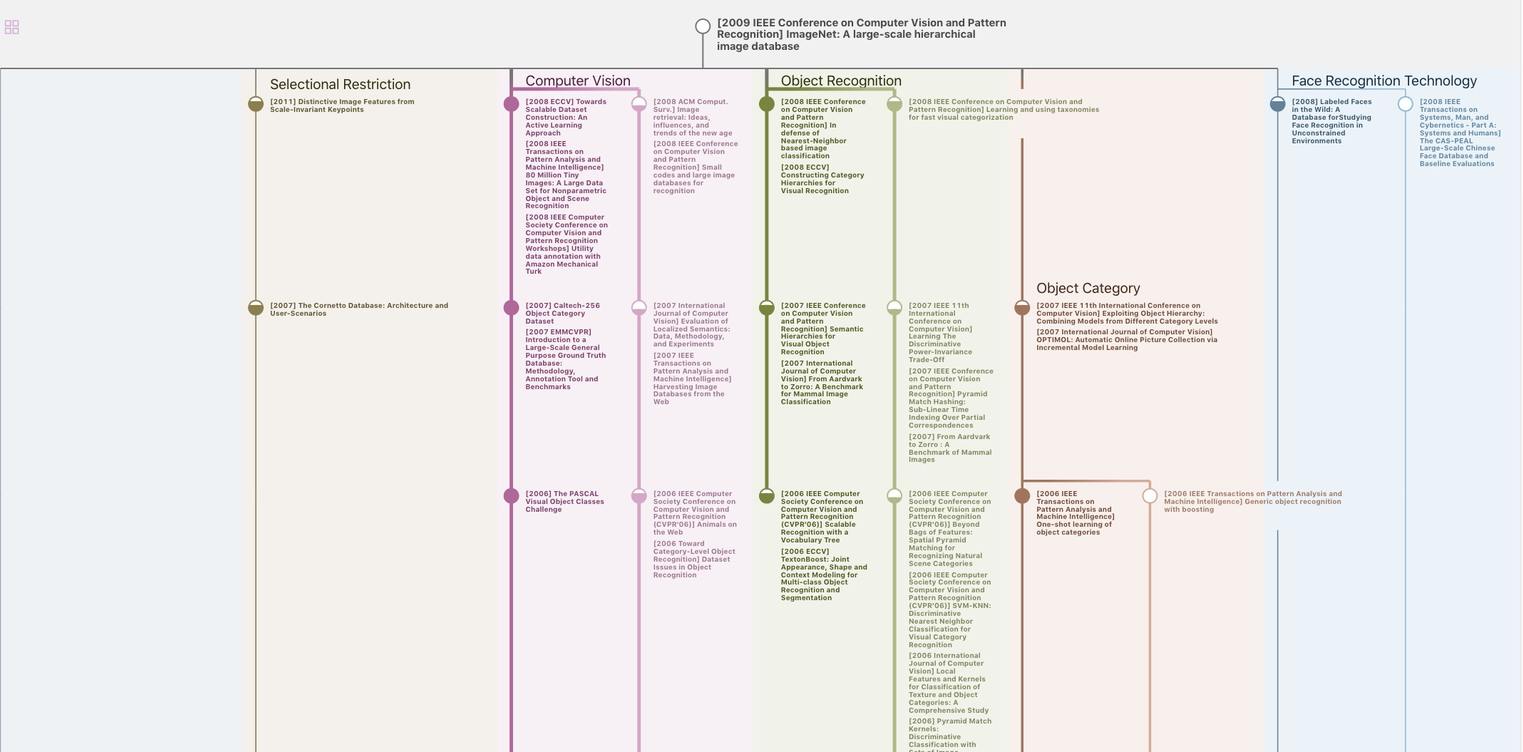
生成溯源树,研究论文发展脉络
Chat Paper
正在生成论文摘要