A Neural Network Approach for High-Dimensional Optimal Control
semanticscholar(2021)
摘要
We propose a neural network approach for solving high-dimensional optimal control problems arising in real-time applications. Our approach yields controls in a feedback form and can therefore handle uncertainties such as perturbations to the system’s state. We accomplish this by fusing the Pontryagin Maximum Principle (PMP) and Hamilton-Jacobi-Bellman (HJB) approaches and parameterizing the value function with a neural network. We train our neural network model using the objective function of the control problem and penalty terms that enforce the HJB equations. Therefore, our training algorithm does not involve data generated by another algorithm. By training on a distribution of initial states, we ensure the controls’ optimality on a large portion of the state-space. Our grid-free approach scales efficiently to dimensions where grids become impractical or infeasible. We demonstrate the effectiveness of our approach on several multi-agent collisionavoidance problems in up to 150 dimensions. Furthermore, we empirically observe that the number of parameters in our approach scales linearly with the dimension of the control problem, thereby mitigating the curse of dimensionality.
更多查看译文
关键词
neural network approach,neural network,control,high-dimensional
AI 理解论文
溯源树
样例
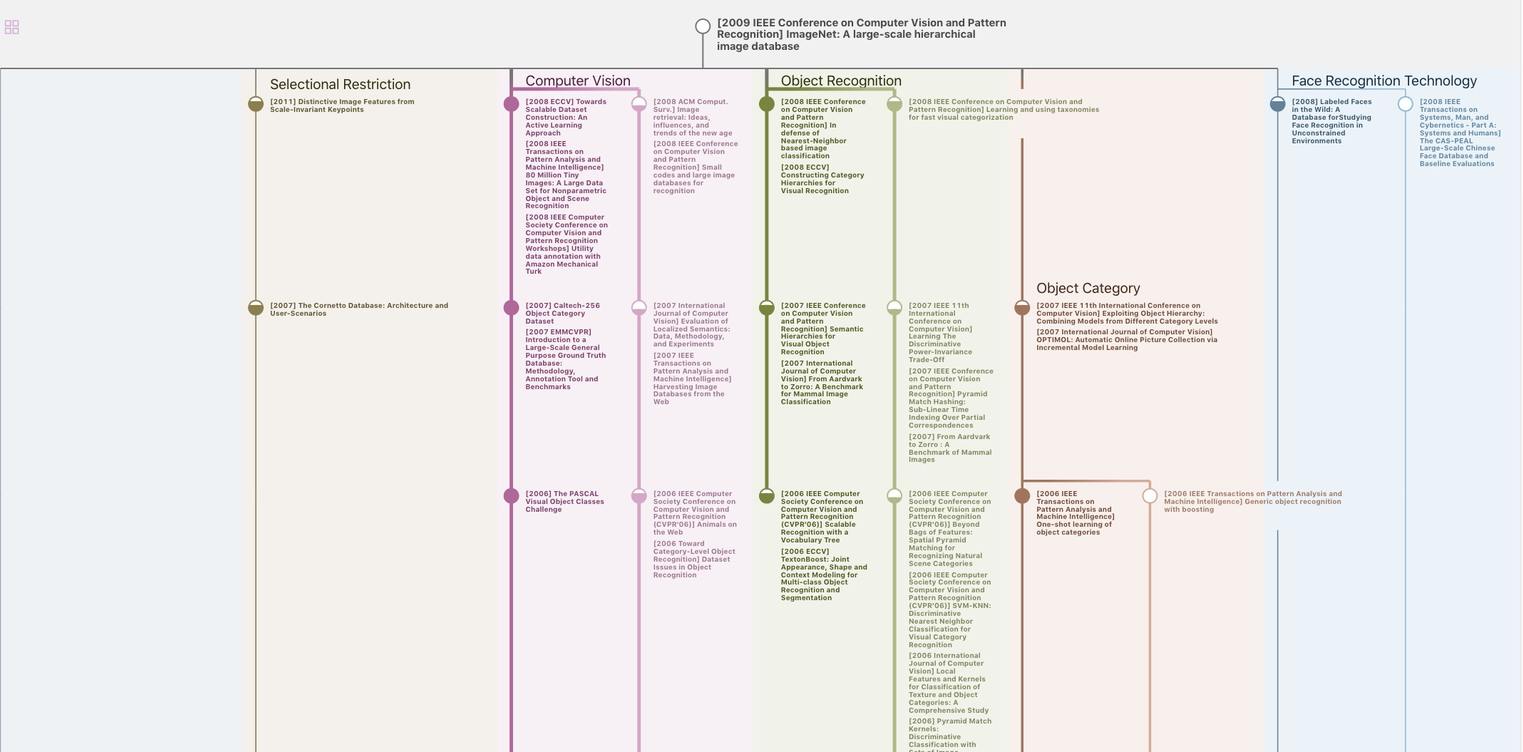
生成溯源树,研究论文发展脉络
Chat Paper
正在生成论文摘要