Progressive-X+: Clustering in the Consensus Space
ArXiv(2021)
摘要
We propose Progressive-X, a new algorithm for finding an unknown number of geometric models, e.g., homographies. The problem is formalized as finding dominant model instances progressively without forming crisp pointto-model assignments. Dominant instances are found via RANSAC-like sampling and a consolidation process driven by a model quality function considering previously proposed instances. New ones are found by clustering in the consensus space. This new formulation leads to a simple iterative algorithm with state-of-the-art accuracy while running in real-time on a number of vision problems. Also, we propose a sampler reflecting the fact that real-world data tend to form spatially coherent structures. The sampler returns connected components in a progressively growing neighborhood-graph. We present a number of applications where the use of multiple geometric models improves accuracy. These include using multiple homographies to estimate relative poses for global SfM; pose estimation from generalized homographies; and trajectory estimation of fast-moving objects.
更多查看译文
关键词
consensus space,clustering
AI 理解论文
溯源树
样例
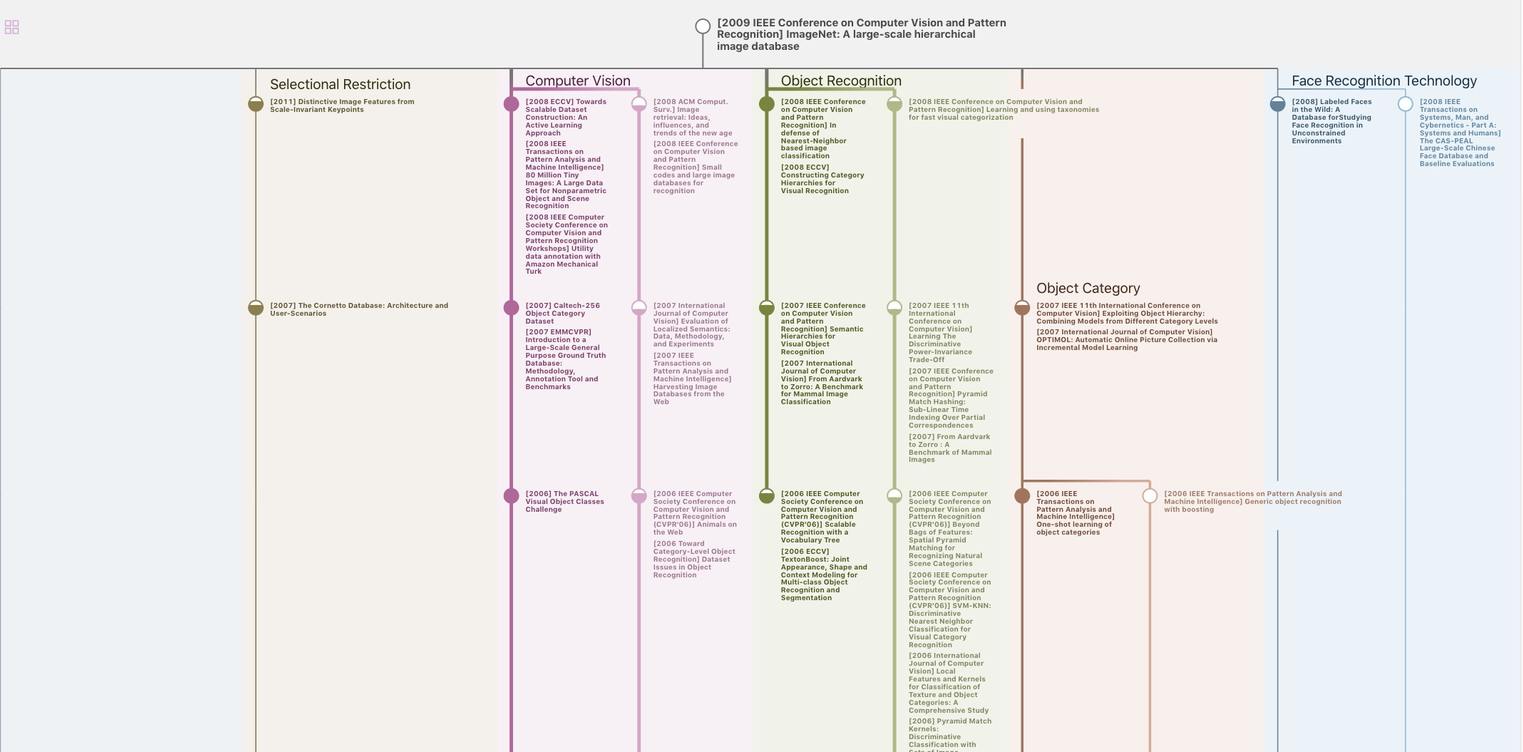
生成溯源树,研究论文发展脉络
Chat Paper
正在生成论文摘要