Preface for the 2nd Edition of the International Knowledge Graph Construction Workshop
semanticscholar(2021)
摘要
More and more knowledge graphs are constructed for private use, e.g., the Amazon Product Graph [1] or the Fashion Knowledge Graph by Zalando5,or public use, e.g., DBpedia6 or Wikidata7. While techniques to automatically construct KGs from existing Web objects exist (e.g., scraping Web tables), there is still room for improvement. So far, constructing knowledge graphs was considered an engineering task, however, more scientifically robust methods keep on emerging. These methods were widely questioned for their verbosity, low performance or difficulty of use, while the data sources’ variety and complexity cause further syntax and semantic interoperability issues. Declarative methods (mapping languages) for describing rules to construct knowledge graphs and approaches to execute those rules keep on emerging. Nevertheless constructing knowledge graphs is still not a straightforward task because several existing challenges remain and yet the barriers to construct knowledge graphs are not lowered enough to be easily and broadly adopted by industry. These reasons and the vastly populated knowledge graph construction W3C Community Group8 show that there are still open questions that require further investigation to come up with groundbreaking solutions. Addressing challenges related to knowledge graphs construction requires wellfounded research, including the investigation of concepts and development of tools as well as methods for their evaluation. R2RML was recommended in 2012 by W3C, and since then, different extensions, alternatives and implementations were proposed [2, 3, 4]. Certain approaches followed the ETL-like paradigm, e.g., SDM-RDFizer [5], RocketRML [6], FunMap [7] and CARML9, while others the query-answering paradigm, e.g., Ultrawrap [8], Morph-RDB [9] and Ontop [10].
更多查看译文
AI 理解论文
溯源树
样例
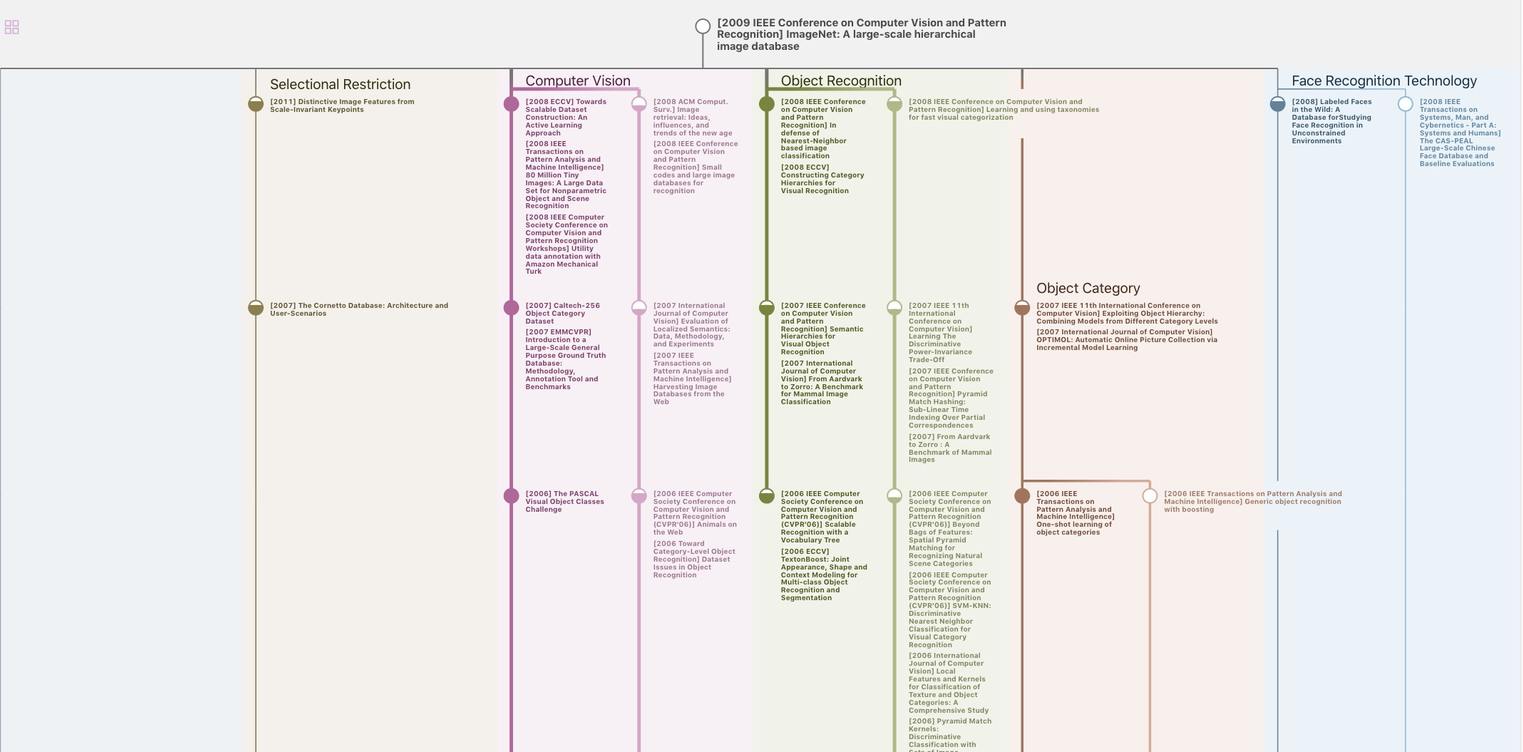
生成溯源树,研究论文发展脉络
Chat Paper
正在生成论文摘要