Exploiting the Hidden Tasks of GANs: Making Implicit Subproblems Explicit
arXiv (Cornell University)(2021)
摘要
We present an alternative perspective on the training of generative adversarial networks (GANs), showing that the training step for a GAN generator decomposes into two implicit subproblems. In the first, the discriminator provides new target data to the generator in the form of "inverse examples" produced by approximately inverting classifier labels. In the second, these examples are used as targets to update the generator via least-squares regression, regardless of the main loss specified to train the network. We experimentally validate our main theoretical result and demonstrate significant improvements over standard GAN training made possible by making these subproblems explicit.
更多查看译文
关键词
gans,implicit subproblems
AI 理解论文
溯源树
样例
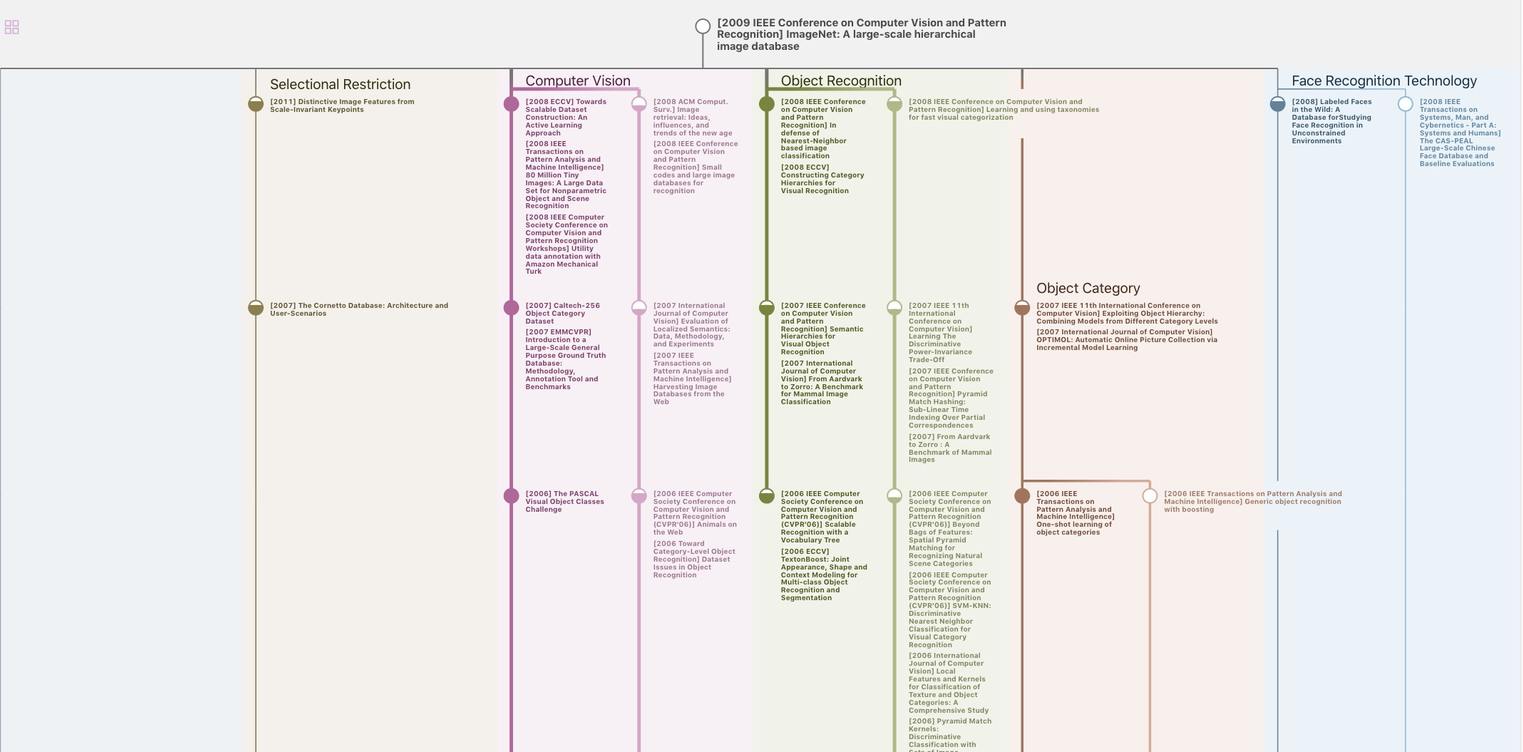
生成溯源树,研究论文发展脉络
Chat Paper
正在生成论文摘要