Combination of Transfer Learning, Recursive Learning and Ensemble Learning for Multi-Day Ahead COVID-19 Cases Prediction in India using Gated Recurrent Unit Networks.
arXiv (Cornell University)(2021)
摘要
The current COVID-19 pandemic has put a huge challenge on the Indian health infrastructure. With more and more people getting affected during the second wave, the hospitals were over-burdened, running out of supplies and oxygen. In this scenario, prediction of the number of COVID-19 cases beforehand might have helped in the better utilization of limited resources and supplies. This manuscript deals with the prediction of new COVID-19 cases, new deaths and total active cases for multiple days in advance. The proposed method uses gated recurrent unit networks as the main predicting model. A study is conducted by building four models that are pre-trained on the data from four different countries (United States of America, Brazil, Spain and Bangladesh) and are fine-tuned or retrained on India's data. Since the four countries chosen have experienced different types of infection curves, the pre-training provides a transfer learning to the models incorporating diverse situations into account. Each of the four models then give a multiple days ahead predictions using recursive learning method for the Indian test data. The final prediction comes from an ensemble of the predictions of the combination of different models. This method with two countries, Spain and Brazil, is seen to achieve the best performance amongst all the combinations as well as compared to other traditional regression models.
更多查看译文
关键词
transfer learning,gated recurrent unit networks,ensemble learning,prediction,recursive learning,multi-day
AI 理解论文
溯源树
样例
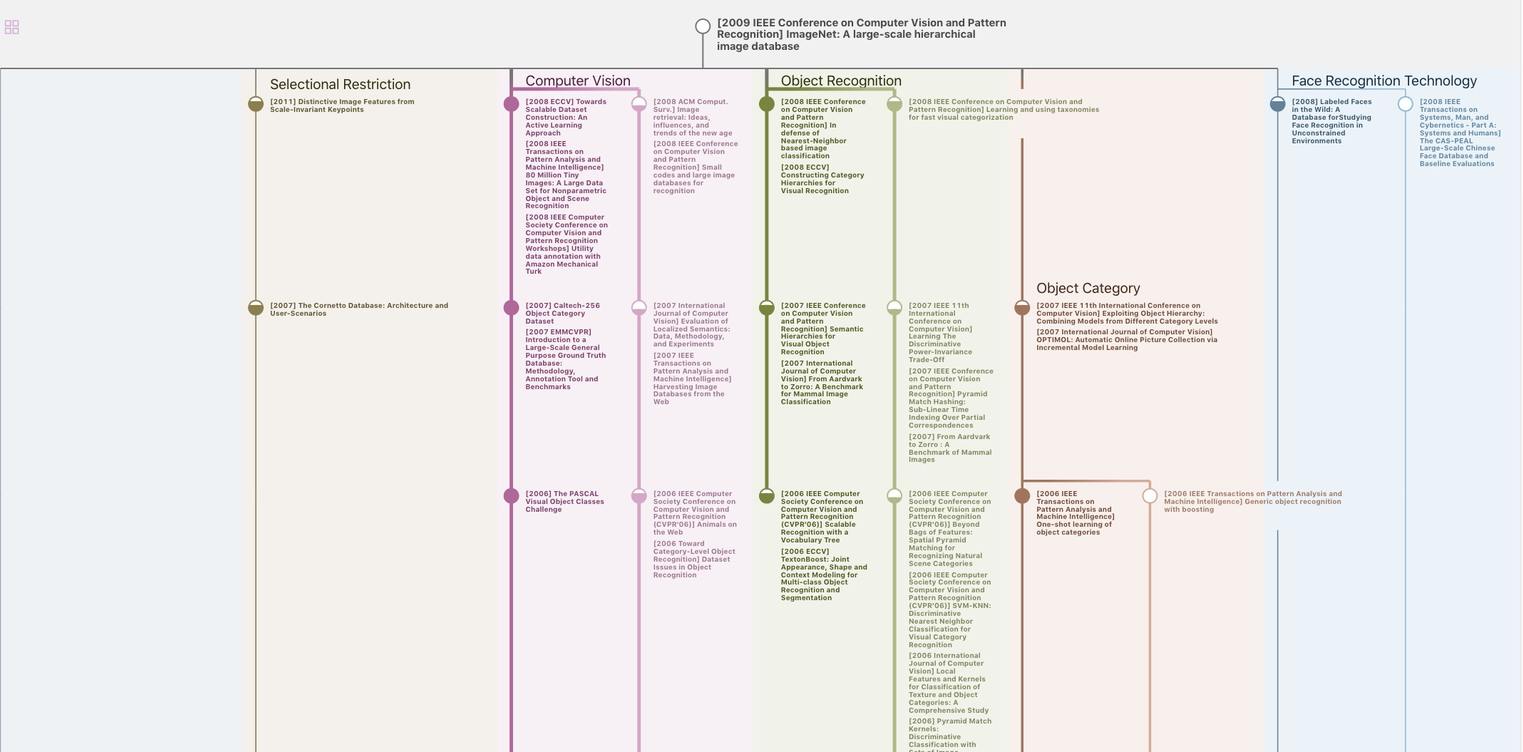
生成溯源树,研究论文发展脉络
Chat Paper
正在生成论文摘要