Global canopy height estimation with GEDI LIDAR waveforms and Bayesian deep learning
ArXiv(2021)
摘要
NASA’s Global Ecosystem Dynamics Investigation (GEDI) is a key climate mission whose goal is to advance our understanding of the role of forests in the global carbon cycle. While GEDI is the first space-based LIDAR explicitly optimized to measure vertical forest structure predictive of aboveground biomass, the accurate interpretation of this vast amount of waveform data across the broad range of observational and environmental conditions is challenging. Here, we present a novel supervised machine learning approach to interpret GEDI waveforms and regress canopy top height globally. We propose a Bayesian convolutional neural network (CNN) to avoid the explicit modelling of unknown effects, such as atmospheric noise. The model learns to extract robust features that generalize to unseen geographical regions and, in addition, yields reliable estimates of predictive uncertainty. Ultimately, the global canopy top height estimates produced by our model have an expected RMSE of 2.7 m with low bias.
更多查看译文
关键词
global canopy height estimation,gedi lidar waveforms,deep learning
AI 理解论文
溯源树
样例
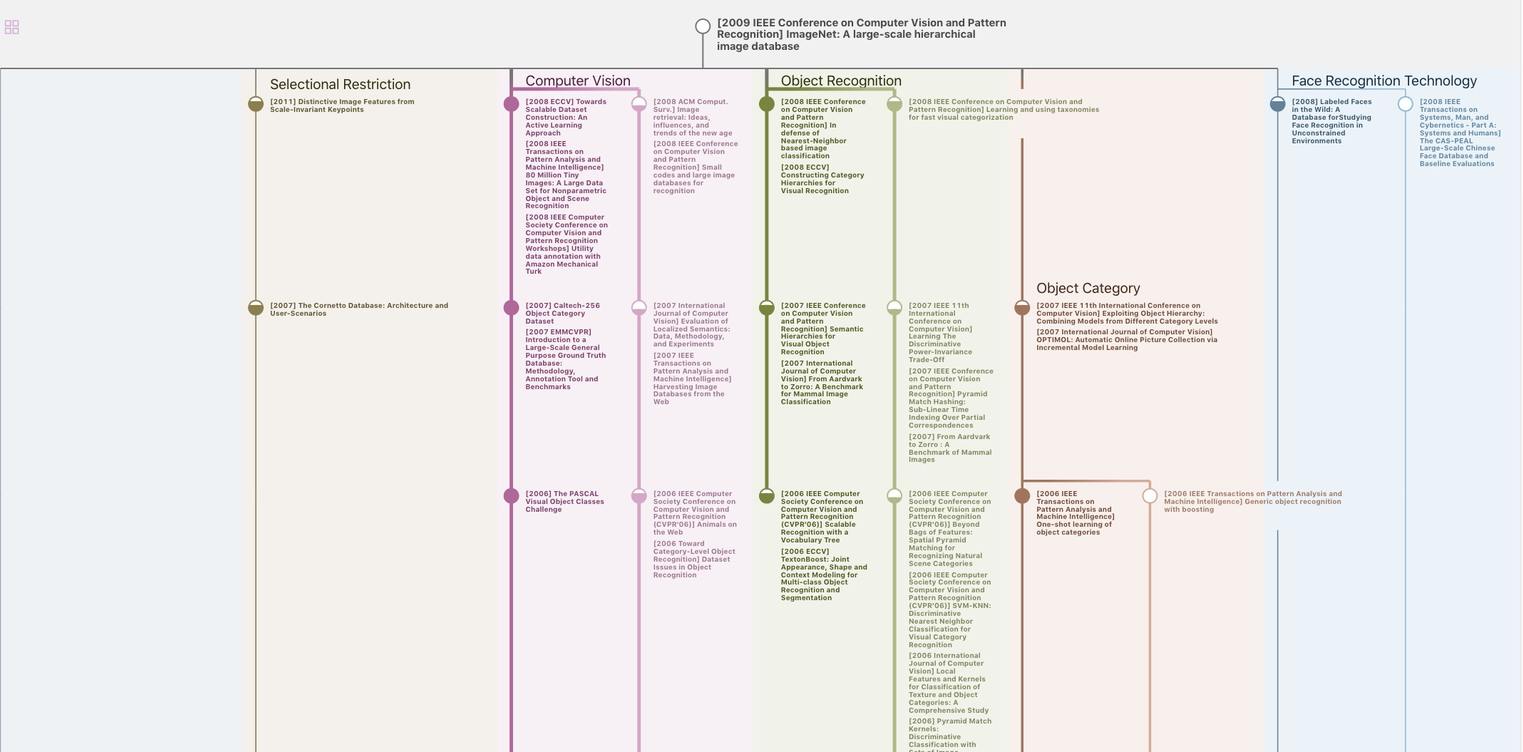
生成溯源树,研究论文发展脉络
Chat Paper
正在生成论文摘要