Dynamics and Neural Collapse in Deep Classifiers trained with the Square Loss
semanticscholar(2021)
摘要
Recent results suggest that square loss performs on par with cross-entropy loss in classification tasks for deep networks. While the theoretical understanding of training deep networks with the cross-entropy loss has been growing, the study of square loss for classification has been lacking. Here we study the dynamics of training under Gradient Descent techniques and show that we can expect convergence to minimum norm solutions when both Weight Decay (WD) and normalization techniques, like Batch Normalization (BN), are used. We perform numerical simulations that show approximate independence on initial conditions as suggested by our analysis, while in the absence of BN+WD we find that good solutions can be achieved for small initializations. We prove that quasi-interpolating solutions obtained by gradient descent in the presence of WD are expected to show the recently discovered behavior of Neural Collapse and describe other predictions of the theory. This is an update to CBMM Memo 112. This material is based upon work supported by the Center for Brains, Minds and Machines (CBMM), funded by NSF STC award CCF-1231216. Dynamics and Neural Collapse in Deep Classifiers trained with the Square Loss Akshay Rangamani1, Mengjia Xu1,2, Andrzej Banburski1, Qianli Liao1, Tomaso Poggio1 1Center for Brains, Minds and Machines, MIT 2Division of Applied Mathematics, Brown University
更多查看译文
AI 理解论文
溯源树
样例
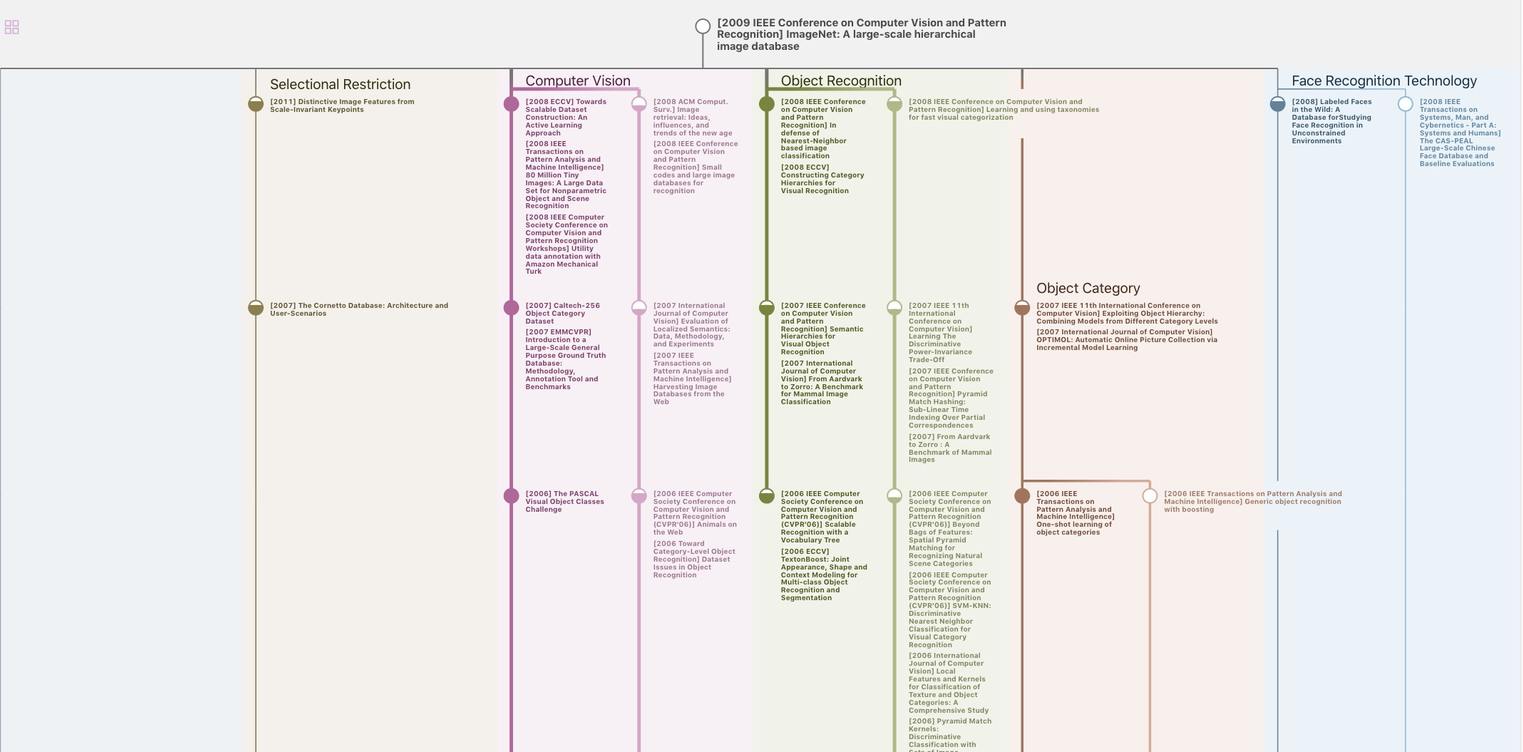
生成溯源树,研究论文发展脉络
Chat Paper
正在生成论文摘要