A Learning-Based Optimization Approach for Autonomous Ridesharing Platforms with Service-Level Contracts and On-Demand Hiring of Idle Vehicles
TRANSPORTATION SCIENCE(2022)
摘要
Current mobility services cannot compete on equal terms with self-owned mobility products concerning service quality. Because of supply and demand imbalances, ridesharing users invariably experience delays, price surges, and rejections. Traditional approaches often fail to respond to demand fluctuations adequately because service levels are, to some extent, bounded by fleet size. With the emergence of autonomous vehicles, however, the characteristics of mobility services change and new opportunities to overcome the prevailing limitations arise. In this paper, we consider an autonomous ridesharing problem in which idle vehicles are hired on-demand in order to meet the service-level requirements of a heterogeneous user base. In the face of uncertain demand and idle vehicle supply, we propose a learning-based optimization approach that uses the dual variables of the underlying assignment problem to iteratively approximate the marginal value of vehicles at each time and location under different availability settings. These approximations are used in the objective function of the optimization problem to dispatch, rebalance, and occasionally hire idle third-party vehicles in a high-resolution transportation network of Manhattan, New York City. The results show that the proposed policy outperforms a reactive optimization approach in a variety of vehicle availability scenarios while hiring fewer vehicles. Moreover, we demonstrate that mobility services can offer strict service-level contracts to different user groups featuring both delay and rejection penalties.
更多查看译文
关键词
autonomous ridesharing platform,stochastic heterogeneous demand,stochastic vehicle supply,machine learning,approximate dynamic programming,service-level contracts,on-demand hiring
AI 理解论文
溯源树
样例
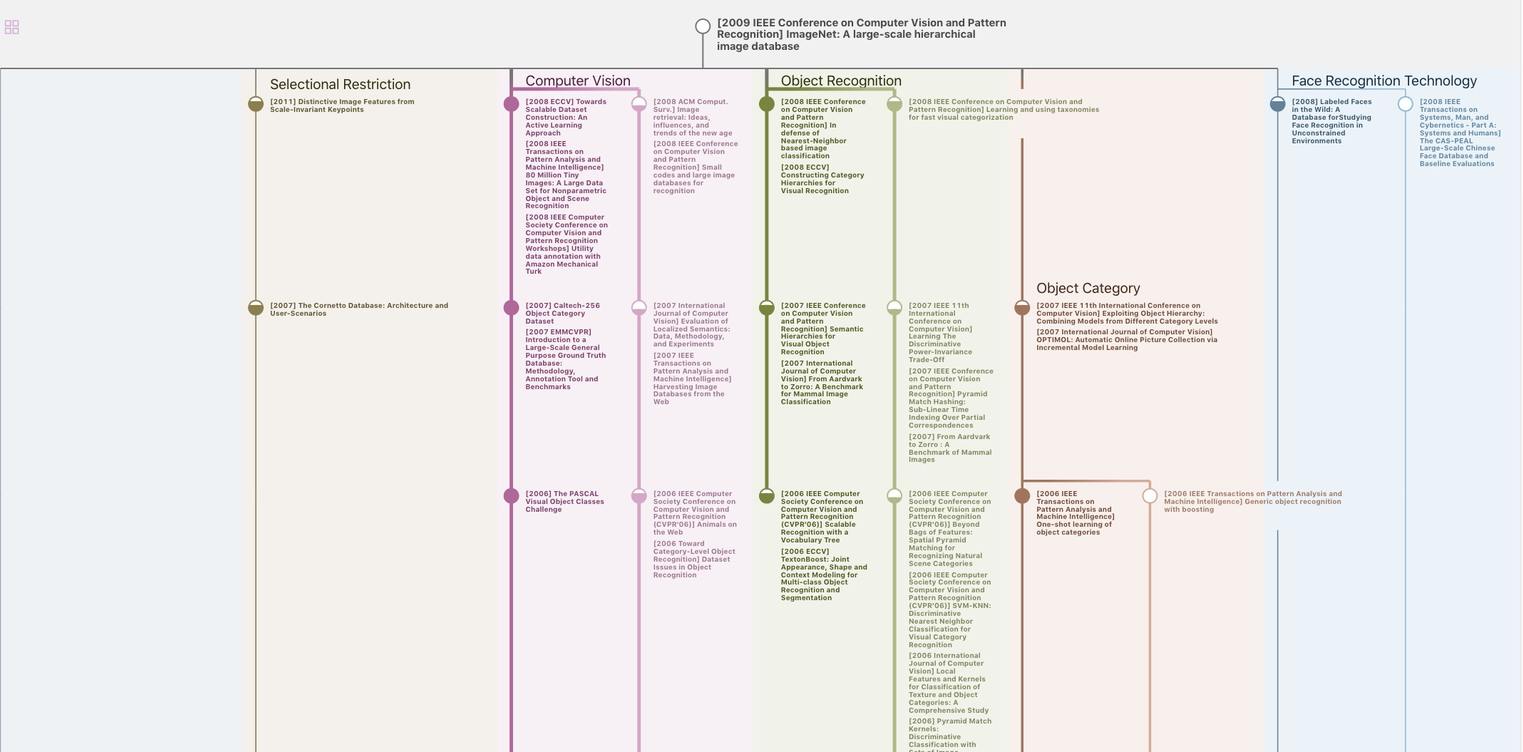
生成溯源树,研究论文发展脉络
Chat Paper
正在生成论文摘要