GraphPiece: Efficiently Generating High-Quality Molecular Graph with Substructures
ArXiv(2021)
摘要
Molecular graph generation is a fundamental but challenging task in various applications such as drug discovery and material science, which requires generating valid molecules with desired properties. Auto-regressive models, which usually construct graphs following sequential actions of adding nodes and edges at the atom-level, have made rapid progress in recent years. However, these atom-level models ignore high-frequency subgraphs that not only capture the regularities of atomic combination in molecules but also are often related to desired chemical properties. In this paper, we propose a method to automatically discover such common substructures, which we call graph pieces, from given molecular graphs. Based on graph pieces, we leverage a variational autoencoder to generate molecules in two phases: piece-level graph generation followed by bond completion. Experiments show that our graph piece variational autoencoder achieves better performance over state-of-the-art baselines on property optimization and constrained property optimization tasks with higher computational efficiency.
更多查看译文
关键词
graphpiece,graphpiece,substructures,high-quality
AI 理解论文
溯源树
样例
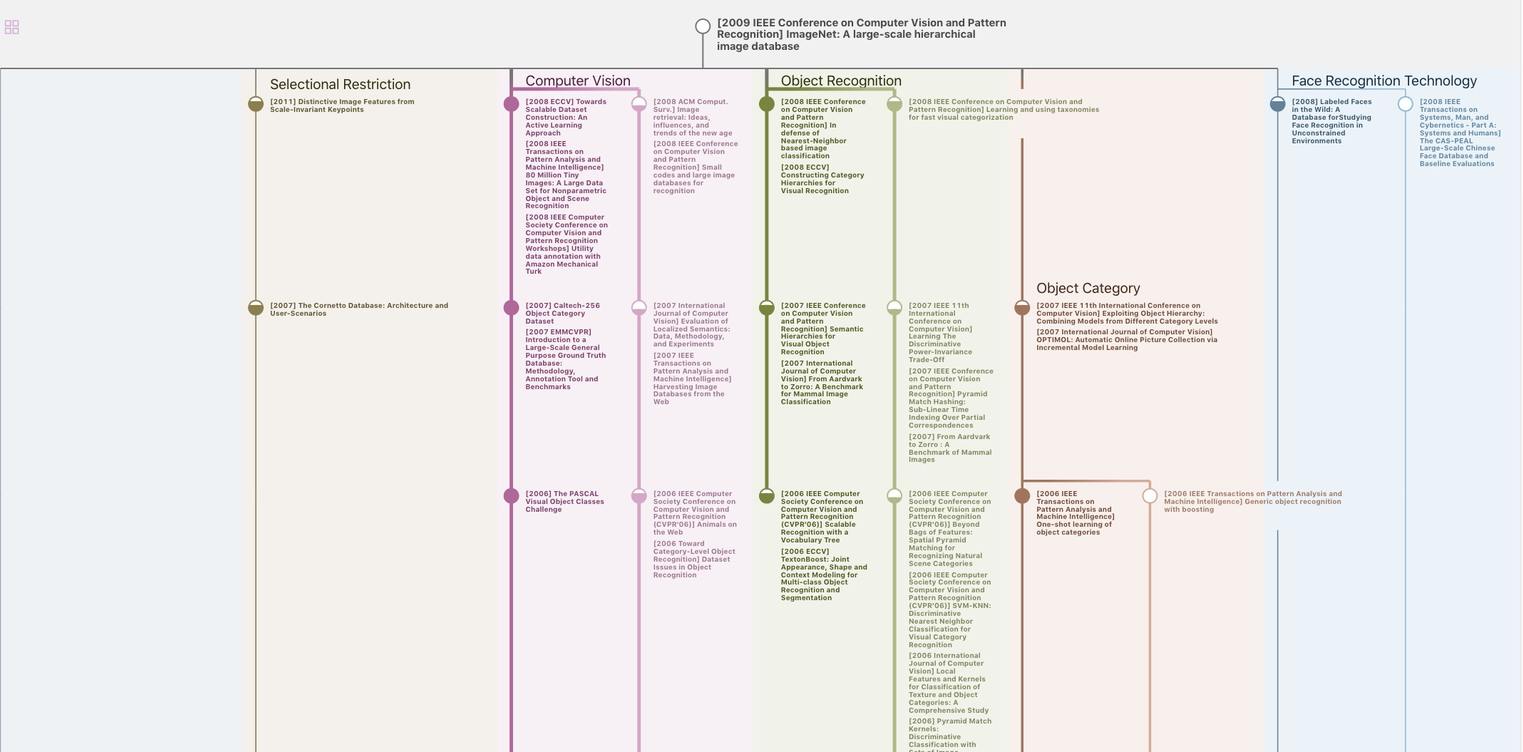
生成溯源树,研究论文发展脉络
Chat Paper
正在生成论文摘要