A Novel Method for Fault Diagnosis of Rolling Bearings Based on Domain-Adversarial Partial Transfer Learning
2020 11th International Conference on Prognostics and System Health Management (PHM-2020 Jinan)(2020)
摘要
With the successful application of deep learning, fault diagnosis of rotating machinery has a significant accuracy improvement. However, these methods based on deep learning need massive labeled samples for training, while it is quite difficult to obtain labeled fault data in the real scenario of engineering. Lacking labeled fault samples, many intelligent methods can not be applied to fault diagnosis. Fortunately, the classical datasets of rotary machines nearly contain all the common fault types. Moreover, transfer learning provides an alternative approach for fault diagnosis of rotating machinery under the situation of lacking labeled fault data. Therefore, a novel intelligent method based on domain-adversarial partial transfer learning is proposed in this paper for the settlement of the above problem, named DA-PTL. The proposed model consists of three parts: feature extractor, domain discriminator, and sample classifier. By transfer learning, the method can realize the fault diagnosis of the target bearings using an open bearing dataset and the gathered data of the target bearings. The experimental results indicate that the proposed method can achieve a good result for fault diagnosis of rolling bearing that is a lack of massive labeled fault samples.
更多查看译文
关键词
domain adversarial,fault diagnosis,partial transfer learning,rolling bearing
AI 理解论文
溯源树
样例
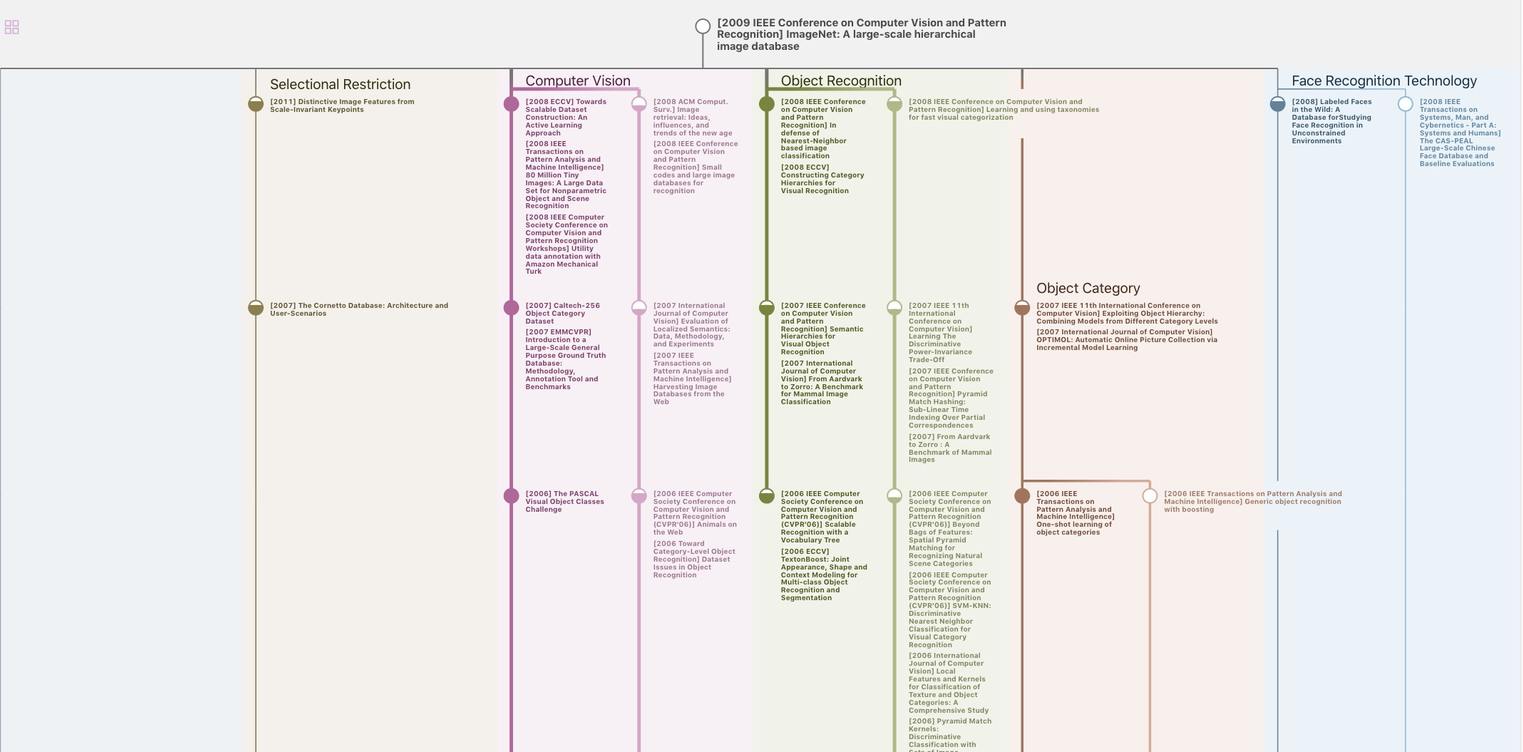
生成溯源树,研究论文发展脉络
Chat Paper
正在生成论文摘要