Computational Toxicology
Systems Medicine(2021)
摘要
Powerful computer systems and growing amounts of curated data have rocketed the implementation of computational disciplines in all fields of drug discovery sciences, including toxicology. Computational toxicology feeds from the needs of researchers, manufacturers, regulators and patients to fully characterize the safety profiles of drugs to avoid the exposure of patients at risk. In this article, we introduce basic concepts of computational toxicology and discuss algorithms and tools that are widely employed to complement experimental laboratory work. Since more than two decades, lab work and particularly animal experimentation have found more and more counterparts by in-silico simulation and computational prediction. These methods are employed to inform scientists early in the drug discovery process, fill data gaps, explain causalities and ultimately reduce experimentation and cycle times. Since computational toxicology is applied throughout the full drug discovery value chain, from research to clinic, it has given rise to using an extraordinarily broad kit of tools employing data mining, curation of structural alerts, molecular fragment methods, quantitative structure-property relationships and machine learning. While the use of data-driven technologies is exploding, and multiple competing applications become available for predictive modeling, their underlying training datasets for individual endpoints may still be small—too small to allow for global applicability. Care must be taken therefore to ensure that predictive methods have been properly validated and are fit-for-purpose. We discuss such practice, as well as model interpretability and how computational methods can contribute to generate hypotheses and support the progression of new molecules.
更多查看译文
关键词
toxicology,computational
AI 理解论文
溯源树
样例
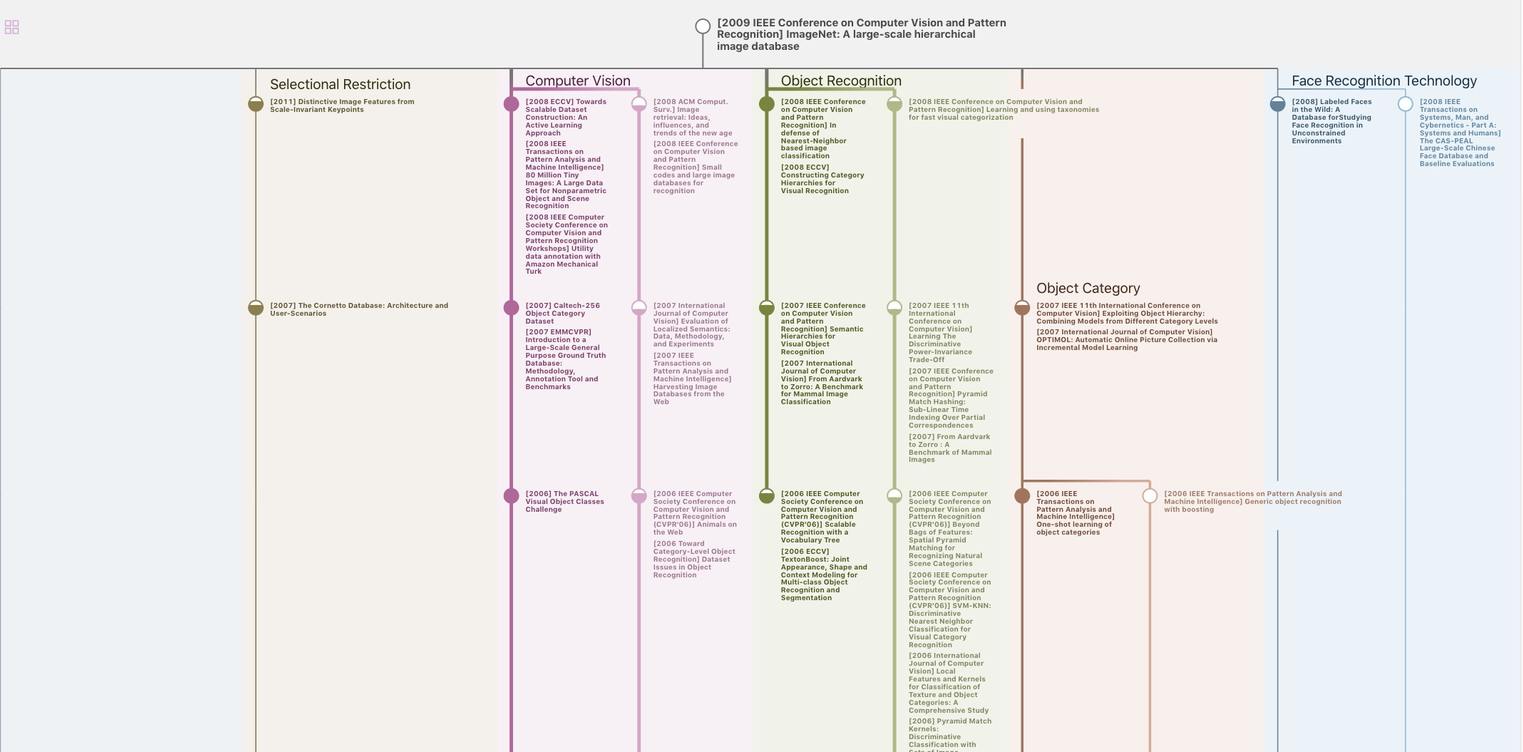
生成溯源树,研究论文发展脉络
Chat Paper
正在生成论文摘要