The sensitivity improved two-test algorithm “SIT2”: a universal optimization strategy for SARS-CoV-2 serology
semanticscholar(2020)
Abstract
Reliable antibody tests are an essential tool to identify individuals who have developed an adaptive immune response to SARS-CoV-2. However, attempts to maximize the specificity of SARS-CoV-2 antibody tests have come at the cost of sensitivity, exacerbating the total test error with increasing seroprevalence. Here, we present a novel method to maximize specificity while maintaining or even increasing sensitivity: the “Sensitivity Improved Two-Test” or “SIT²” algorithm.SIT² involves confirmatory re-testing of samples with results falling in a predefined retesting-zone of an initial screening test, with adjusted cut-offs to increase sensitivity. We verified and compared the performance of SIT² to single tests and orthogonal testing (OTA) in an Austrian cohort (1,117 negative, 64 post-COVID positive samples) and validated the algorithm in an independent British cohort (976 negatives, 536 positives).The specificity of SIT² was superior to single tests and non-inferior to OTA. The sensitivity was maintained or even improved using SIT² when compared to single tests or OTA. SIT² allowed correct identification of infected individuals even when a live virus neutralization assay could not detect antibodies. Compared to single testing or OTA, SIT² significantly reduced total test errors to 0·46% (0·24-0·65) or 1·60% (0·94-2·38) at both 5% or 20% seroprevalence.SIT² proved to be the best diagnostic choice at both 5% and 20% seroprevalence in all tested scenarios. It is an easy algorithm to apply to different available SARS-CoV-2 antibody testing systems and can potentially be helpful for the serology of other infectious diseases.
MoreTranslated text
AI Read Science
Must-Reading Tree
Example
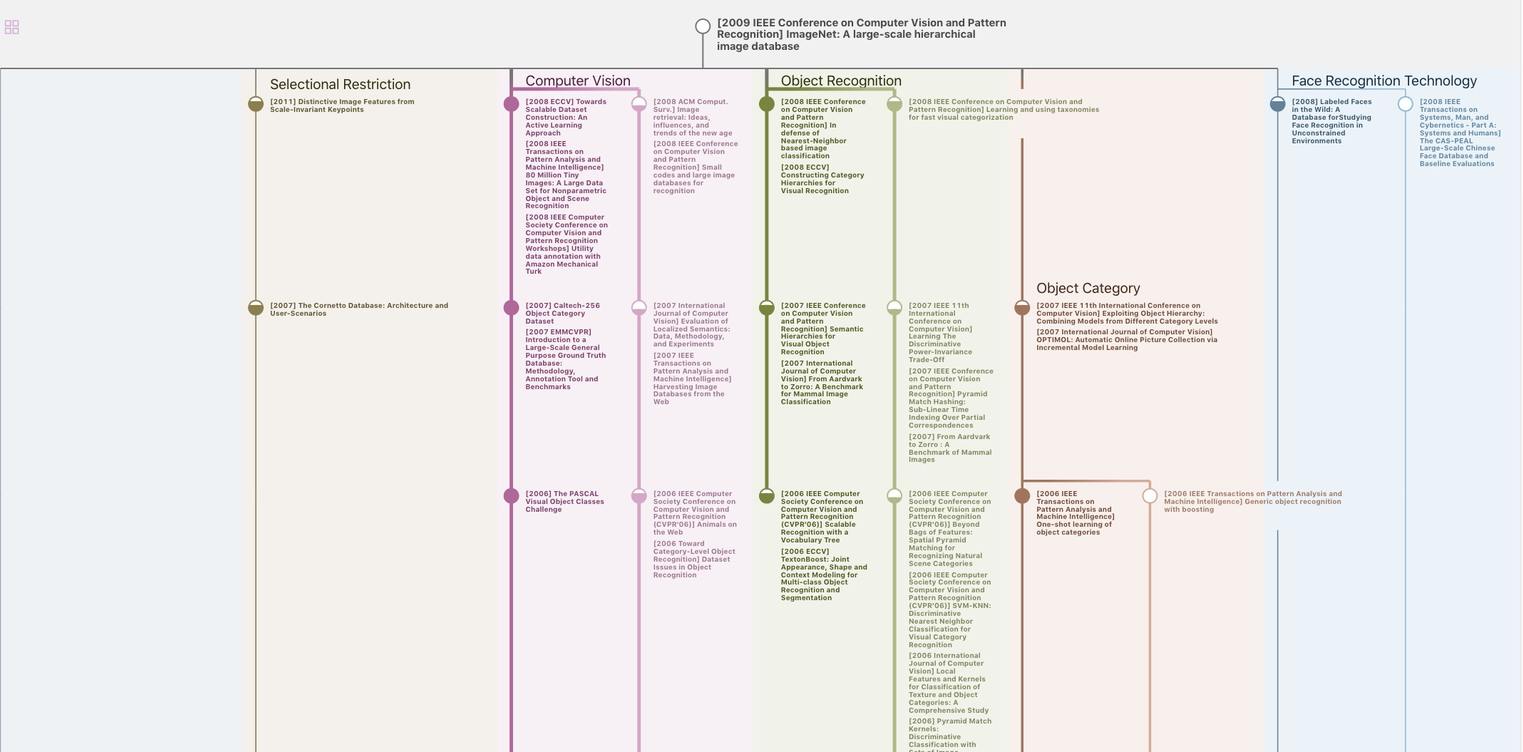
Generate MRT to find the research sequence of this paper
Chat Paper
Summary is being generated by the instructions you defined