Automated detection and staging of malaria parasites from cytological smears using convolutional neural networks.
medRxiv(2021)
摘要
Microscopic examination of blood smears remains the gold standard for laboratory inspection and diagnosis of malaria. Smear inspection is, however, time-consuming and dependent on trained microscopists with results varying in accuracy. We sought to develop an automated image analysis method to improve accuracy and standardization of smear inspection that retains capacity for expert confirmation and image archiving. Here, we present a machine learning method that achieves red blood cell (RBC) detection, differentiation between infected/uninfected cells, and parasite life stage categorization from unprocessed, heterogeneous smear images. Based on a pretrained Faster Region-Based Convolutional Neural Networks (R-CNN) model for RBC detection, our model performs accurately, with an average precision of 0.99 at an intersection-over-union threshold of 0.5. Application of a residual neural network-50 model to infected cells also performs accurately, with an area under the receiver operating characteristic curve of 0.98. Finally, combining our method with a regression model successfully recapitulates intraerythrocytic developmental cycle with accurate lifecycle stage categorization. Combined with a mobile-friendly web-based interface, called PlasmoCount, our method permits rapid navigation through and review of results for quality assurance. By standardizing assessment of Giemsa smears, our method markedly improves inspection reproducibility and presents a realistic route to both routine lab and future field-based automated malaria diagnosis.
更多查看译文
关键词
Artificial intelligence,Giemsa stain,Plasmodium falciparum,gametocytes,residual neural networks (ResNets)
AI 理解论文
溯源树
样例
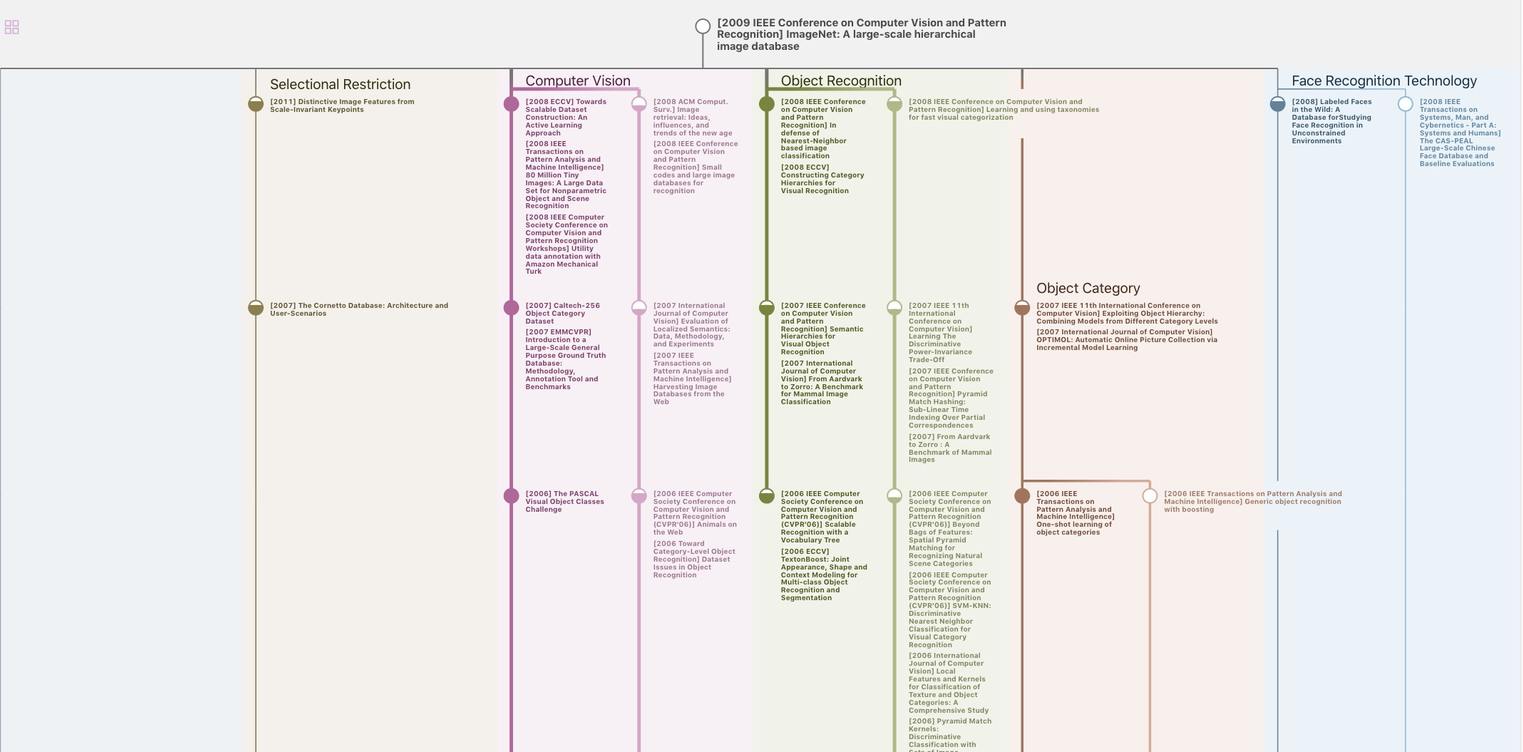
生成溯源树,研究论文发展脉络
Chat Paper
正在生成论文摘要