Physics informed deep learning to super-resolve and cross-calibrate solar magnetograms
Research Square (Research Square)(2021)
摘要
Super-resolution techniques aim to increase the resolution of images by adding detail. Compared to upsampling techniques reliant on interpolation, deep learning-based approaches learn features and their relationships across the training data set to leverage prior knowledge on what low resolution patterns look like in higher resolution images.
As an added benefit, deep neural networks can learn the systematic properties of the target images (i.e.\ texture), combining super-resolution with instrument cross-calibration. While the successful use of super-resolution algorithms for natural images is rooted in creating perceptually convincing results, super-resolution applied to scientific data requires careful quantitative evaluation of performances.
In this work, we demonstrate that deep learning can increase the resolution and calibrate space- and ground-based imagers belonging to different instrumental generations. In addition, we establish a set of measurements to benchmark the performance of scientific applications of deep learning-based super-resolution and calibration.
We super-resolve and calibrate solar magnetic field images taken by the Michelson Doppler Imager (MDI; resolution ~2"/pixel; science-grade, space-based) and the Global Oscillation Network Group (GONG; resolution ~2.5"/pixel; space weather operations, ground-based) to the pixel resolution of images taken by the Helioseismic and Magnetic Imager (HMI; resolution ~0.5"/pixel; last generation, science-grade, space-based).
更多查看译文
关键词
deep learning,solar,super-resolve,cross-calibrate
AI 理解论文
溯源树
样例
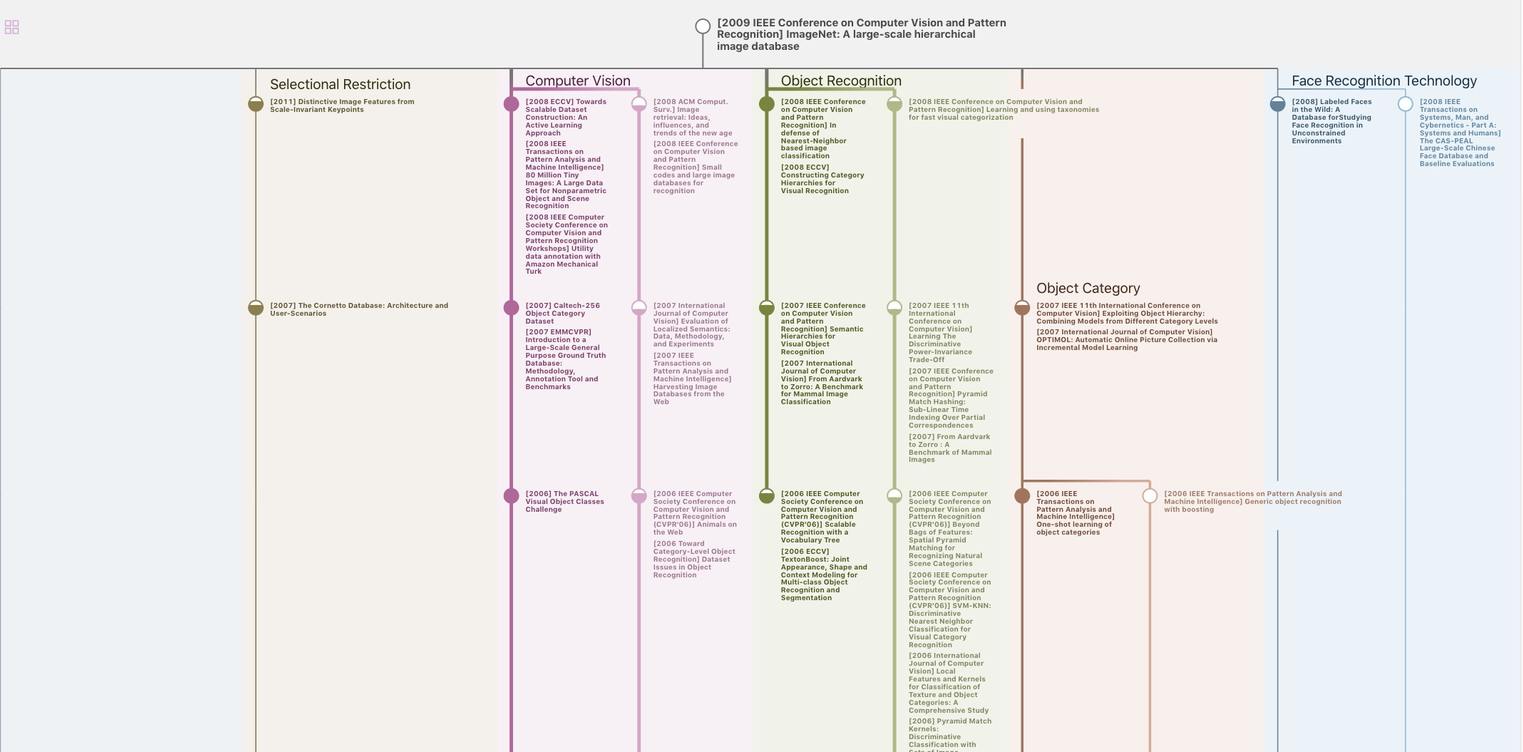
生成溯源树,研究论文发展脉络
Chat Paper
正在生成论文摘要