Unsupervised Adversarial Network Alignment with Reinforcement Learning
ACM TRANSACTIONS ON KNOWLEDGE DISCOVERY FROM DATA(2022)
摘要
Network alignment, which aims at learning amatching between the same entities across multiple information networks, often suffers challenges from feature inconsistency, high-dimensional features, to unstable alignment results. This article presents a novel network alignment framework, Unsupervised Adversarial learning based Network Alignment (UANA), that combines generative adversarial network (GAN) and reinforcement learning (RL) techniques to tackle the above critical challenges. First, we propose a bidirectional adversarial network distribution matching model to perform the bidirectional cross-network alignment translations between two networks, such that the distributions of real and translated networks completely overlap together. In addition, two cross-network alignment translation cycles are constructed for training the unsupervised alignment without the need of prior alignment knowledge. Second, in order to address the feature inconsistency issue, we integrate a dual adversarial autoencoder module with an adversarial binary classification model together to project two copies of the same vertices with high-dimensional inconsistent features into the same low-dimensional embedding space. This facilitates the translations of the distributions of two networks in the adversarial network distribution matching model. Finally, we develop an RL based optimization approach to solve the vertex matching problem in the discrete space of the GAN model, i.e., directly select the vertices in target networks most relevant to the vertices in source networks, without unstable similarity computation that is sensitive to discriminative features and similarity metrics. Extensive evaluation on realworld graph datasets demonstrates the outstanding capability of UANA to address the unsupervised network alignment problem, in terms of both effectiveness and scalability.
更多查看译文
关键词
Unsupervised network alignment,feature inconsistency,high-dimensional features,adversarial network distribution matching,adversarial classification,reinforcement learning
AI 理解论文
溯源树
样例
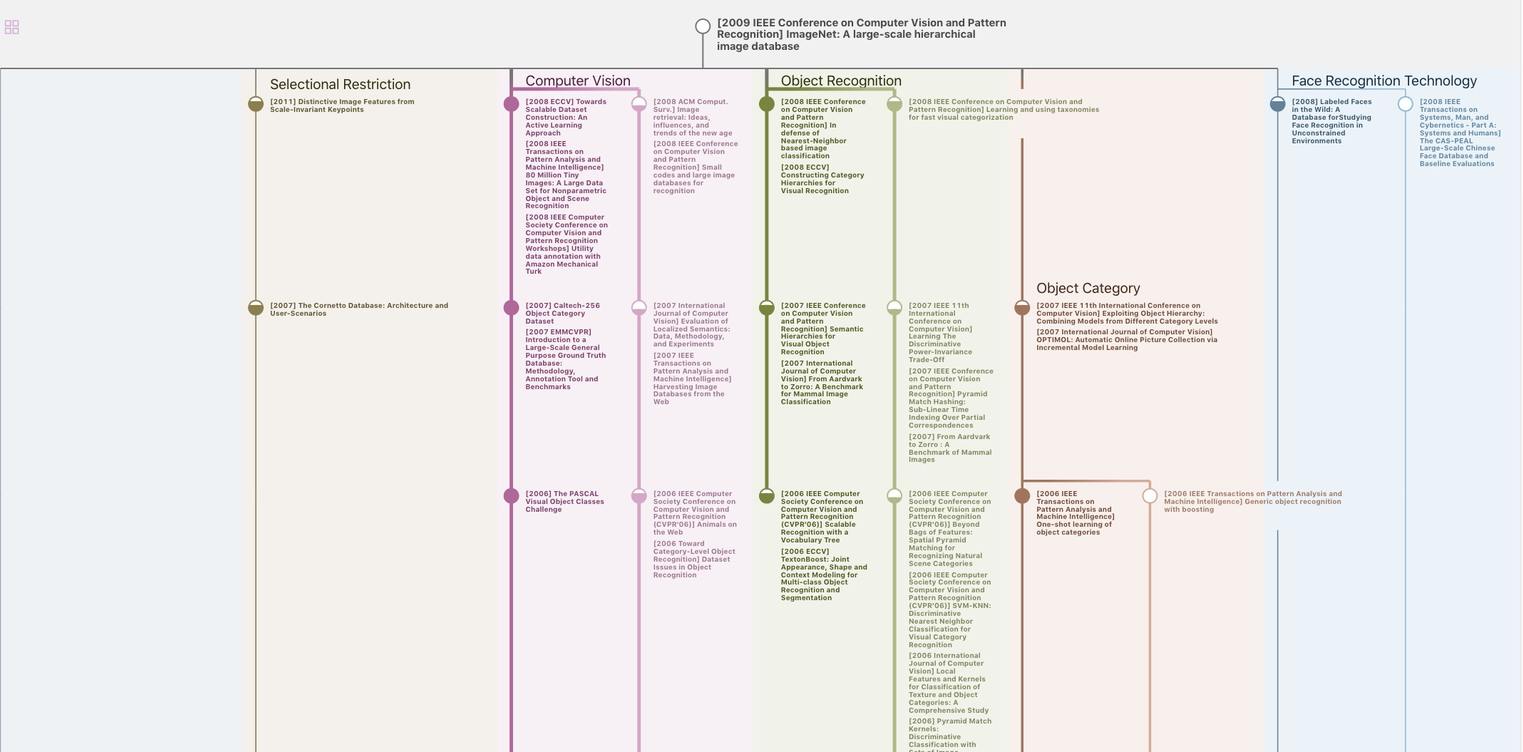
生成溯源树,研究论文发展脉络
Chat Paper
正在生成论文摘要