Probing AndroVul dataset for studies on Android malware classification
Journal of King Saud University - Computer and Information Sciences(2022)
摘要
Security issues in mobile apps are increasingly relevant as this software have become part of the daily life of billions of people. As the dominant OS, Android is a primary target for ill-intentioned programmers willing to exploit its vulnerabilities by spreading malwares. Significant research has been devoted to the identification of these malwares. The current paper is an extension of our previous effort to contribute to said research with a new benchmark of Android vulnerabilities. We proposed AndroVul, a repository for Android security vulnerabilities, that builds on AndroZoo – a well-known Android app dataset – and contains data on vulnerabilities for a representative sample of about 16,000 Android apps. The present paper adds confirmed malwares from the VirusShare dataset and explores more thoroughly the effectiveness of different machine learning techniques, with respect to the classification of malicious apps. We investigated different classifiers and feature selection techniques as well as different combinations for our input data. Our results suggest that the classifier MPL is the leading classifier, with competitive results that favorably compare to recent malware detection work. Additionally, we investigate how to classify (as benign or malicious) AndroZoo apps based on the number of antivirus flags they are tagged with. We found that different thresholds only marginally affect the machine learning classifier results and that the strictest choice (i.e. one flag) performs best on the confirmed malwares from VirusShare.
更多查看译文
关键词
Mobile security,Static analysis,Reverse engineering,Mobile computing,Machine learning
AI 理解论文
溯源树
样例
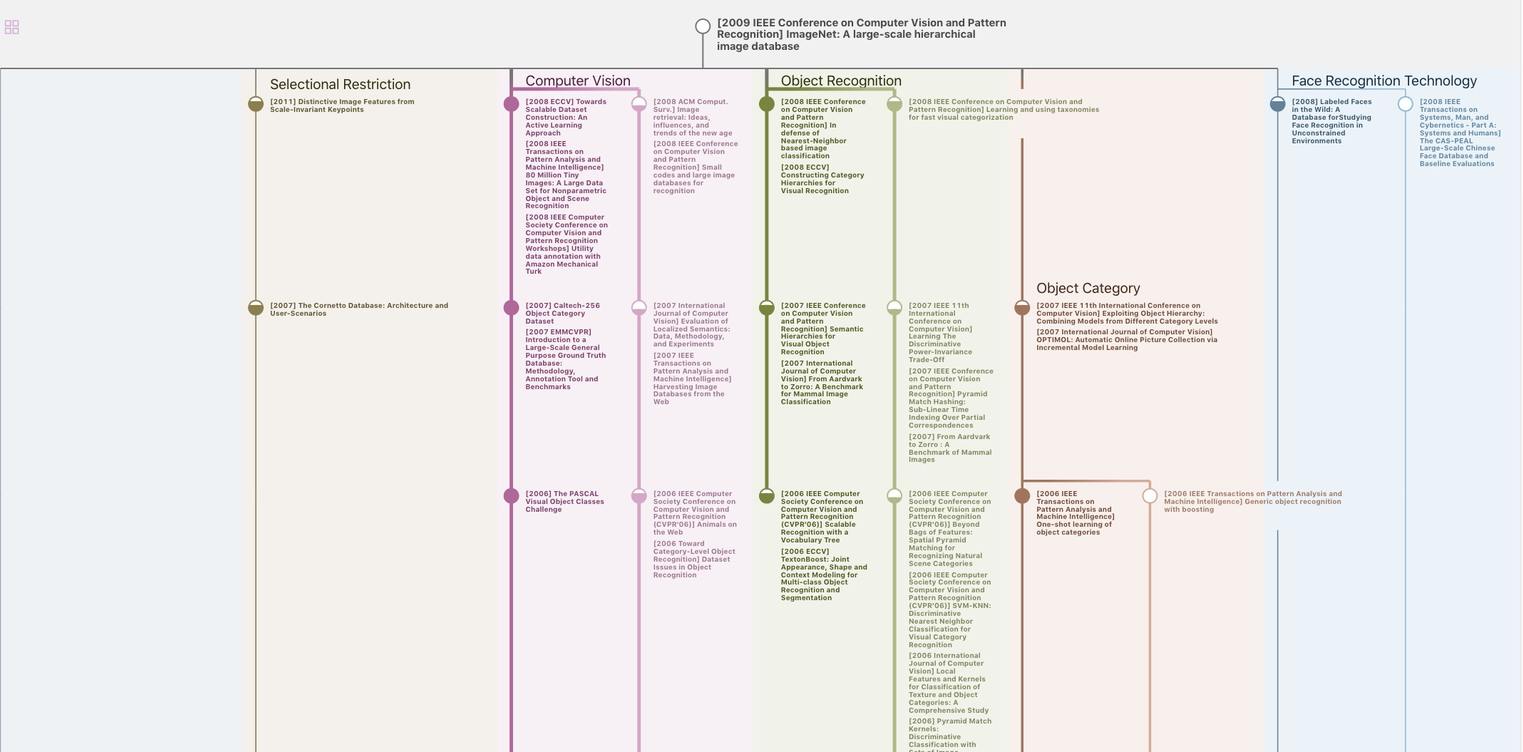
生成溯源树,研究论文发展脉络
Chat Paper
正在生成论文摘要