Estimating the Number of Factors in Exploratory Factor Analysis via Out-of-Sample Prediction Errors
PSYCHOLOGICAL METHODS(2024)
摘要
Translational Abstract Exploratory factor analysis (EFA) is one of the most popular statistical models in psychological science. A key problem in EFA is to estimate the number of factors. In this article, we present a new method for estimating the number of factors based on minimizing the out-of-sample prediction error of candidate factor models. We show in an extensive simulation study that our method slightly outperforms existing methods and is more robust across different specifications of the true factor model than other high performing methods. We provide an implementation of our method in the R-package fspe. Exploratory factor analysis (EFA) is one of the most popular statistical models in psychological science. A key problem in EFA is to estimate the number of factors. In this article, we present a new method for estimating the number of factors based on minimizing the out-of-sample prediction error of candidate factor models. We show in an extensive simulation study that our method slightly outperforms existing methods, including parallel analysis, Bayesian information criterion (BIC), Akaike information criterion (AIC), root mean squared error of approximation (RMSEA), and exploratory graph analysis. In addition, we show that, among the best performing methods, our method is the one that is most robust across different specifications of the true factor model. We provide an implementation of our method in the R-package fspe.
更多查看译文
关键词
exploratory factor analysis,factor retention,model selection,prediction error
AI 理解论文
溯源树
样例
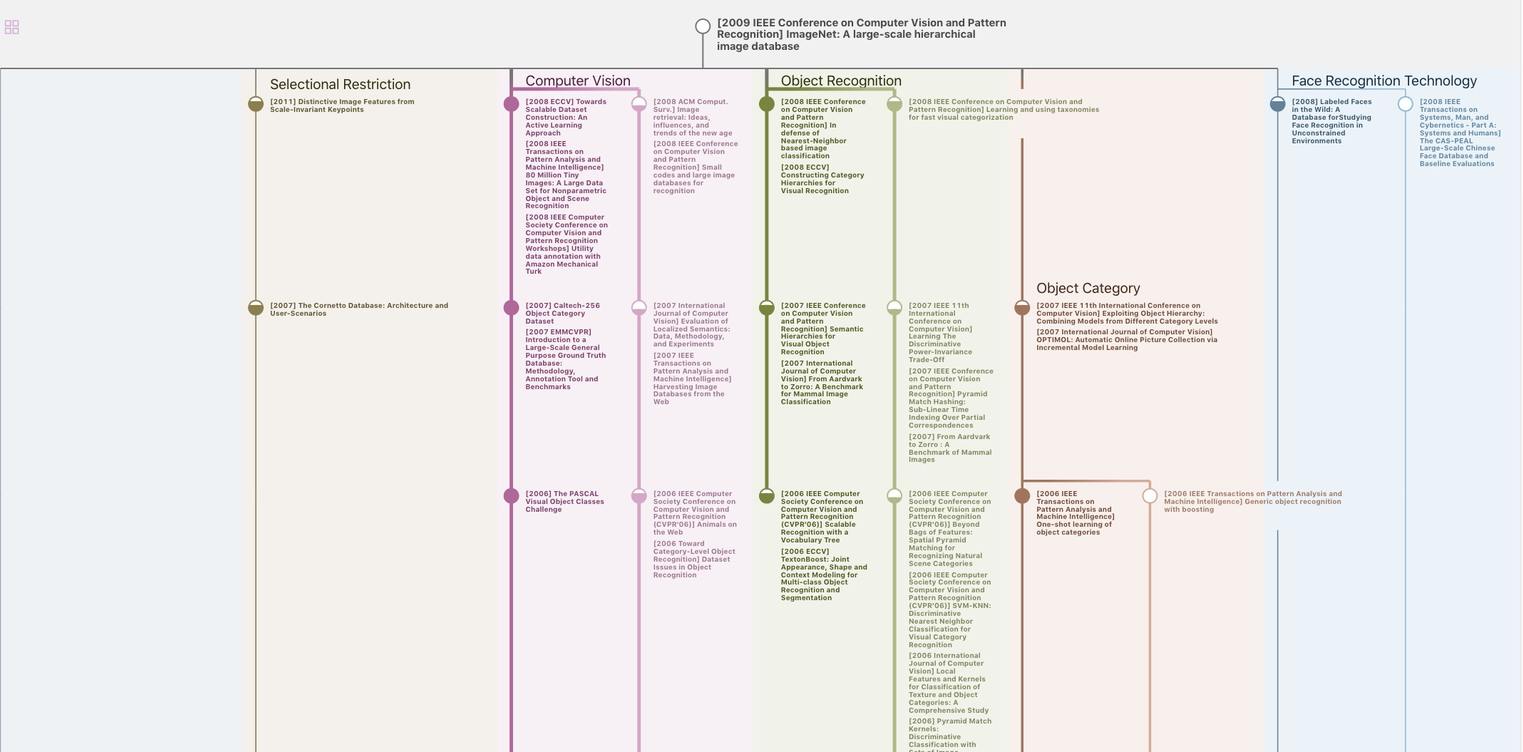
生成溯源树,研究论文发展脉络
Chat Paper
正在生成论文摘要