Identification of Uncertainty in Artificial Neural Networks
semanticscholar(2019)
摘要
Neural networks are the backbone of environment perception systems for autonomous driving. While they achieve state-of-the-art performance in most computer vision tasks, they typically do not provide self-evaluation with respect to their predictions. For autonomous vehicles, though, it is vital that the system actively reasons about its limitations. The aim of this work is to identify uncertainty in neural network decisions for semantic segmentation. To systematically evaluate this, we develop a methodology to compare neural networks’ performance in out-of-distribution detection and uncertainty estimation. As the core contribution of our work, we propose a novel approach to learn uncertainty estimation for out-of-distribution detection from unlabeled parts of the training data. Our approach only extends the training strategy and therefore does not require any changes to network architecture or runtime. We show that resulting networks perform en par with state-of-the-art methods that require much greater computational efforts. Consequently, any given architecture for segmentation can be trained to also provide out-of-distribution detection.
更多查看译文
AI 理解论文
溯源树
样例
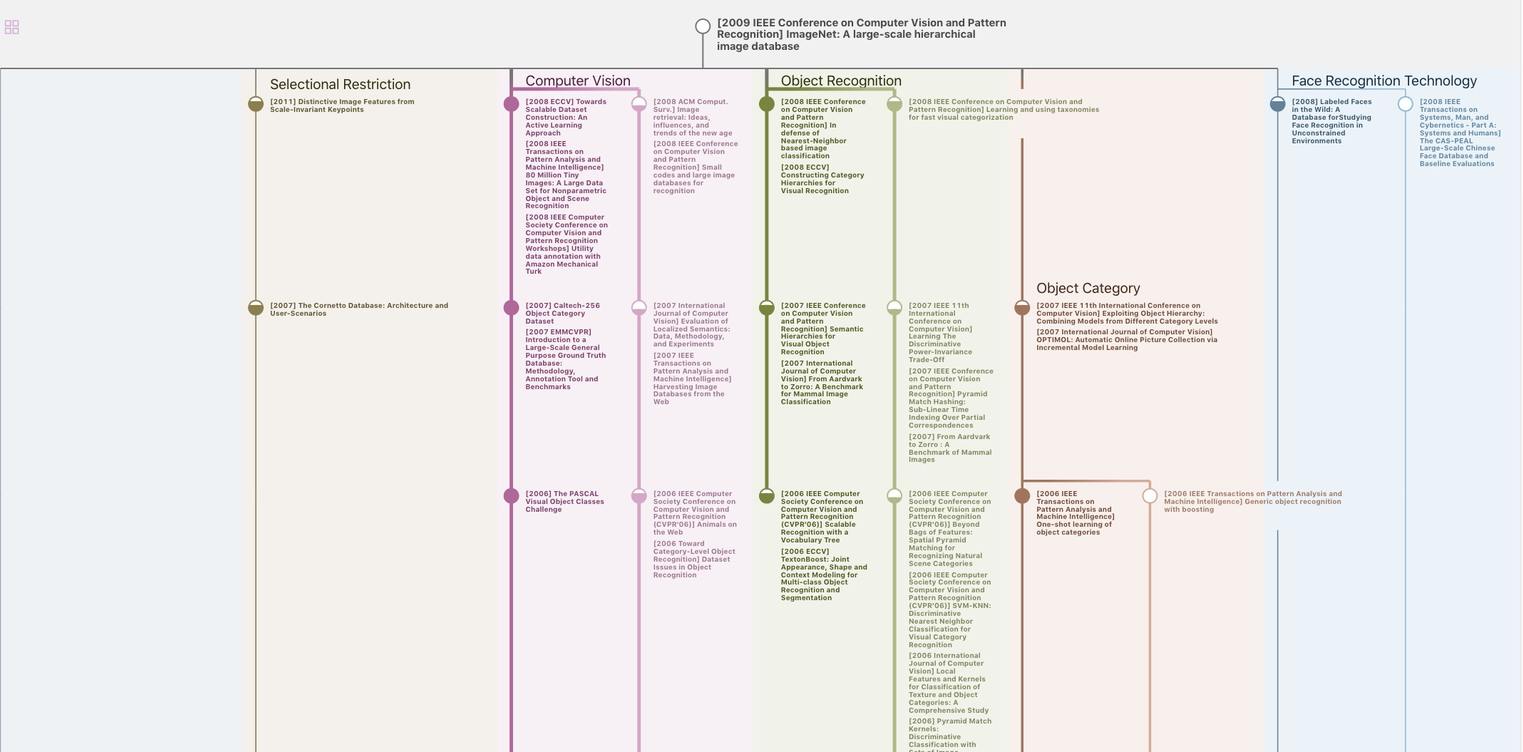
生成溯源树,研究论文发展脉络
Chat Paper
正在生成论文摘要